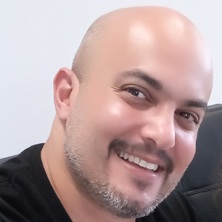
Overview
Availability
- Professor Fred Roosta is:
- Available for supervision
Fields of research
Qualifications
- Doctor of Philosophy, The University of British Columbia
Research interests
-
Artificial Intelligence
-
Machine Learning
-
Numerical Optimization
-
Numerical Analysis
-
Computational Statistics
-
Scientific Computing
Works
Search Professor Fred Roosta’s works on UQ eSpace
2021
Conference Publication
Non-PSD matrix sketching with applications to regression and optimization
Feng, Zhili, Roosta, Fred and Woodruff, David P. (2021). Non-PSD matrix sketching with applications to regression and optimization. Conference on Uncertainty in Artificial Intelligence, Online, 27-29 July 2021. San Diego, CA, United States: Association For Uncertainty in Artificial Intelligence (AUAI).
2020
Journal Article
Newton-type methods for non-convex optimization under inexact Hessian information
Xu, Peng, Roosta, Fred and Mahoney, Michael W. (2020). Newton-type methods for non-convex optimization under inexact Hessian information. Mathematical Programming, 184 (1-2), 35-70. doi: 10.1007/s10107-019-01405-z
2020
Conference Publication
Newton-admm: a distributed GPU-accelerated optimizer for multiclass classification problems
Fang, Chih-Hao, Kylasa, Sudhir B., Roosta, Fred, Mahoney, Michael W. and Grama, Ananth (2020). Newton-admm: a distributed GPU-accelerated optimizer for multiclass classification problems. International Conference on High Performance Computing, Networking, Storage and Analysis (SC), Atlanta, GA, United States, 9-19 November 2020. Piscataway, NJ, United States: IEEE Computer Society. doi: 10.1109/SC41405.2020.00061
2020
Conference Publication
Second-order optimization for non-convex machine learning: an empirical study
Xu, Peng, Roosta, Fred and Mahoney, Michael W. (2020). Second-order optimization for non-convex machine learning: an empirical study. SIAM International Conference on Data Mining, Cincinnati, OH, United States, 7-9 May 2020. Philadelphia, PA, United States: Society for Industrial and Applied Mathematics. doi: 10.1137/1.9781611976236.23
2020
Conference Publication
DINO: Distributed Newton-type optimization method
Crane, Rixon and Roosta, Fred (2020). DINO: Distributed Newton-type optimization method. International Conference on Machine Learning, Virtual, 12-18 July 2020. San Diego, CA, United States: International Conference on Machine Learning.
2020
Book Chapter
Parallel optimization techniques for machine learning
Kylasa, Sudhir, Fang, Chih-Hao, Roosta, Fred and Grama, Ananth (2020). Parallel optimization techniques for machine learning. Parallel algorithms in computational science and engineering. (pp. 381-417) edited by Ananth Grama and Ahmed H. Sameh. Cham, Switzerland: Birkhauser. doi: 10.1007/978-3-030-43736-7_13
2019
Conference Publication
GPU accelerated sub-sampled Newton's method for convex classification problems
Kylasa, Sudhir, Roosta, Fred (Farbod), Mahoney, Michael W. and Grama, Ananth (2019). GPU accelerated sub-sampled Newton's method for convex classification problems. SIAM International Conference on Data Mining, Calgary, Canada, 2-4 May 2019. Philadelphia, PA, United States: Society for Industrial and Applied Mathematics. doi: 10.1137/1.9781611975673.79
2019
Book Chapter
Optimization methods for inverse problems
Ye, Nan, Roosta-Khorasani, Farbod and Cui, Tiangang (2019). Optimization methods for inverse problems. 2017 MATRIX annals. (pp. 121-140) edited by David R. Wood, Jan de Gier, Cheryl E. Praeger and Terence Tao. Cham, Switzerland: Springer. doi: 10.1007/978-3-030-04161-8_9
2019
Conference Publication
DINGO: Distributed Newton-type method for gradient-norm optimization
Crane, Rixon and Roosta, Fred (2019). DINGO: Distributed Newton-type method for gradient-norm optimization. Advances in Neural Information Processing Systems, Vancouver, BC, Canada, 8-14 December 2019. Maryland Heights, MO United States: Morgan Kaufmann Publishers.
2019
Conference Publication
Exchangeability and kernel invariance in trained MLPs
Tsuchida, Russell, Roosta, Fred and Gallagher, Marcus (2019). Exchangeability and kernel invariance in trained MLPs. Twenty-Eighth International Joint Conference on Artificial Intelligence (IJCAI-19, Macao, China, 10-16 August 2019. Marina del Rey, CA USA: International Joint Conferences on Artificial Intelligence. doi: 10.24963/ijcai.2019/498
2018
Journal Article
Sub-sampled Newton methods
Roosta-Khorasani, Farbod and Mahoney, Michael W. (2018). Sub-sampled Newton methods. Mathematical Programming, 174 (1-2), 293-326. doi: 10.1007/s10107-018-1346-5
2018
Conference Publication
Out-of-sample extension of graph adjacency spectral embedding
Levin, Keith, Roosta-Khorasani, Farbod, Mahoney, Michael W. and Priebe, Carey E. (2018). Out-of-sample extension of graph adjacency spectral embedding. 35th International Conference on Machine Learning, Stockholm, Sweden, 10-15 July 2018. Cambridge, MA, United States: M I T Press.
2018
Conference Publication
Invariance of weight distributions in rectified MLPs
Tsuchida, Russell, Roosta-Khorasani, Farbod and Gallagher, Marcus (2018). Invariance of weight distributions in rectified MLPs. 35th International Conference on Machine Learning, Stockholm, Sweden, 10-15 July 2018. Cambridge, MA, United States: M I T Press.
2018
Conference Publication
GIANT: Globally improved approximate Newton method for distributed optimization
Wang, Shusen, Roosta-Khorasani, Farbod, Xu, Peng and Mahoney, Michael W. (2018). GIANT: Globally improved approximate Newton method for distributed optimization. 32nd Conference on Neural Information Processing Systems, NeurIPS 2018, Montreal, QC, Canada, 2 - 8 December, 2018. Maryland Heights, MO, United States: Neural information processing systems foundation.
2018
Conference Publication
FLAG n’ FLARE: fast linearly-coupled adaptive gradient methods
Cheng, Xiang, Roosta-Khorasani, Farbod, Palombo, Stefan, Bartlett, Peter L. and Mahoney, Michael W. (2018). FLAG n’ FLARE: fast linearly-coupled adaptive gradient methods. Twenty-First International Conference on Artificial Intelligence and Statistics, Lanzarote, Canary Islands, 9-11 April 2018. Cambridge, MA, United States: M I T Press.
2017
Journal Article
Variational perspective on local graph clustering
Fountoulakis, Kimon, Roosta-Khorasani, Farbod, Shun, Julian, Cheng, Xiang and Mahoney, Michael W. (2017). Variational perspective on local graph clustering. Mathematical Programming, 174 (1-2), 553-573. doi: 10.1007/s10107-017-1214-8
2017
Conference Publication
The Union of Intersections (UoI) method for interpretable data driven discovery and prediction
Bouchard, Kristofer E, Bujan, Alejandro F, Roosta-Khorasani, Farbod, Prabhat, Snijders, Jian-Hua Mao, Chang, Edward F, Mahoney, Michael W and Bhattacharyya, Sharmodeep (2017). The Union of Intersections (UoI) method for interpretable data driven discovery and prediction. 31st Annual Conference on Neural Information Processing Systems (NIPS), Long Beach, CA United States, 4-9 December 2017. Maryland Heights, MO, United States: Morgan Kaufmann Publishers.
2016
Journal Article
Algorithms that satisfy a stopping criterion, probably
Ascher, Uri and Roosta-Khorasani, Farbod (2016). Algorithms that satisfy a stopping criterion, probably. Vietnam Journal of Mathematics, 44 (1), 49-69. doi: 10.1007/s10013-015-0167-6
2016
Conference Publication
Sub-sampled Newton methods with non-uniform sampling
Xu, Peng, Yang, Jiyan, Roosta-Khorasani, Farbod, Re, Christopher and Mahoney, Michael (2016). Sub-sampled Newton methods with non-uniform sampling. Neural Information Processing Systems 2016, Barcelona Spain, 5 - 10 December 2016 . La Jolla, CA United States: Neural Information Processing Systems Foundation.
2016
Conference Publication
Parallel local graph clustering
Shun, Julian, Roosta-Khorasani, Farbod, Fountoulakis, Kimon and Mahoney, Michael W. (2016). Parallel local graph clustering. International Conferenceon Very Large Data Bases, New Delhi, India, 5-9 September 2016. New York, United States: Association for Computing Machinery. doi: 10.14778/2994509.2994522
Supervision
Availability
- Professor Fred Roosta is:
- Available for supervision
Before you email them, read our advice on how to contact a supervisor.
Available projects
-
Non-convex Optimization for Machine Learning
Design, analysis, and implementation of novel optimization algorithms for optimization of modern non-convex machine learning problems.
-
Interpretable AI - Theory and Practice
This project will extend and innovate, both theoretically and practically, interpretable methods in AI that are transparent and explainable to improve trust and usability. It will also explore novel approaches for uncertainty quantification and understanding causality.
-
Exploring Predictivity--Parsimony Trade-off In Scientific Machine Learning
This project will investigate, both theoretically and empirically, novel statistical techniques to explore the trade-offs between high-generalization performance and low-model complexity for scientific machine learning.
-
Novel Machine Learning Models for Scientific Discovery
To extend the application range of machine learning to scientific domains, this project will design, analyze and implement novel machine learning techniques that learn from data, while conform with known properties of the underlying scientific models.
-
Automated Discovery of Optimization and Linear Algebra Algorithms
Using reinforcement learning to automate algorithmic discovery, this project aims to develop novel variants of first- and second-order optimization methods, randomized numerical linear algebra techniques, and mixed-integer programming approaches.
-
Second-order Optimization Algorithms for Machine Learning
This project aims to develop the next generation of second-order optimization methods for training complex machine learning models, with particular focus on constrained problems arising in scientific machine learning applications.
-
Distributed Optimization Algorithms for Large-scale Machine Learning
This project aims to design, analyze and implement efficient optimization algorithms suitable for distributed computing environments, with focus on large-scale machine learning.
Supervision history
Current supervision
-
Doctor Philosophy
Stochastic Simulation and Optimization Methods for Machine Learning
Principal Advisor
-
Doctor Philosophy
Interpretable AI-Theory and Practice
Principal Advisor
Other advisors: Dr Quan Nguyen
-
Doctor Philosophy
Novel Machine Learning Models for Scientific Discovery
Principal Advisor
-
Doctor Philosophy
Characterizing Influence and Sensitivity in the Interpolating Regime
Principal Advisor
Other advisors: Associate Professor Marcus Gallagher
-
Doctor Philosophy
Newton type methods for constrained optimization
Principal Advisor
-
Doctor Philosophy
Forecasting the Market Capitalisation of ASX Listed Junior Resource Companies through an Artificial Neural Network
Associate Advisor
Other advisors: Associate Professor Mehmet Kizil, Dr Micah Nehring
-
Doctor Philosophy
Efficient graph representation learning with neural networks and self-supervised learning
Associate Advisor
Other advisors: Dr Nan Ye
Completed supervision
-
2023
Doctor Philosophy
Newton-MR Methods for Non-convex Smooth Unconstrained Optimizations
Principal Advisor
Other advisors: Professor Dirk Kroese
-
2022
Doctor Philosophy
Efficient second-order optimisation methods for large scale machine learning
Principal Advisor
Other advisors: Associate Professor Marcus Gallagher
-
2022
Doctor Philosophy
Discounting-free Policy Gradient Reinforcement Learning from Transient States
Associate Advisor
Other advisors: Associate Professor Marcus Gallagher
-
2020
Doctor Philosophy
Results on Infinitely Wide Multi-layer Perceptrons
Associate Advisor
Other advisors: Associate Professor Marcus Gallagher
-
2018
Doctor Philosophy
Advances in Monte Carlo Methodology
Associate Advisor
Other advisors: Dr Slava Vaisman, Professor Dirk Kroese
Media
Enquiries
For media enquiries about Professor Fred Roosta's areas of expertise, story ideas and help finding experts, contact our Media team: