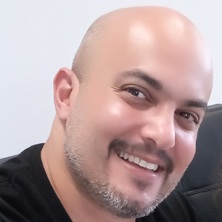
Overview
Availability
- Professor Fred Roosta is:
- Available for supervision
Fields of research
Qualifications
- Doctor of Philosophy, The University of British Columbia
Research interests
-
Artificial Intelligence
-
Machine Learning
-
Numerical Optimization
-
Numerical Analysis
-
Computational Statistics
-
Scientific Computing
Works
Search Professor Fred Roosta’s works on UQ eSpace
2016
Conference Publication
Sub-sampled Newton methods with non-uniform sampling
Xu, Peng, Yang, Jiyan, Roosta-Khorasani, Farbod, Re, Christopher and Mahoney, Michael (2016). Sub-sampled Newton methods with non-uniform sampling. Neural Information Processing Systems 2016, Barcelona Spain, 5 - 10 December 2016 . La Jolla, CA United States: Neural Information Processing Systems Foundation.
2015
Journal Article
Schur properties of convolutions of gamma random variables
Roosta-Khorasani, Farbod and Szekely, Gábor J. (2015). Schur properties of convolutions of gamma random variables. Metrika, 78 (8), 997-1014. doi: 10.1007/s00184-015-0537-9
2015
Journal Article
Improved bounds on sample size for implicit matrix trace estimators
Roosta-Khorasani, Farbod and Ascher, Uri (2015). Improved bounds on sample size for implicit matrix trace estimators. Foundations of Computational Mathematics, 15 (5), 1187-1212. doi: 10.1007/s10208-014-9220-1
2015
Journal Article
Assessing stochastic algorithms for large scale nonlinear least squares problems using extremal probabilities of linear combinations of gamma random variables
Roosta-Khorasani, Farbod, Székely, Gábor J. and Ascher, Uri M. (2015). Assessing stochastic algorithms for large scale nonlinear least squares problems using extremal probabilities of linear combinations of gamma random variables. SIAM/ASA Journal on Uncertainty Quantification, 3 (1), 61-90. doi: 10.1137/14096311X
2014
Journal Article
Data completion and stochastic algorithms for PDE inversion problems with many measurements
Roosta-Khorasani, Farbod, van den Doel, Kees and Ascher, Uri (2014). Data completion and stochastic algorithms for PDE inversion problems with many measurements. Electronic Transactions on Numerical Analysis, 42, 177-196.
2014
Journal Article
Stochastic algorithms for inverse problems involving pdes and many measurements
Roosta-Khorasani, Farbod, Van Den Doel, Kees and Ascher, Uri (2014). Stochastic algorithms for inverse problems involving pdes and many measurements. SIAM Journal on Scientific Computing, 36 (5), S3-S22. doi: 10.1137/130922756
Supervision
Availability
- Professor Fred Roosta is:
- Available for supervision
Before you email them, read our advice on how to contact a supervisor.
Available projects
-
Non-convex Optimization for Machine Learning
Design, analysis, and implementation of novel optimization algorithms for optimization of modern non-convex machine learning problems.
-
Interpretable AI - Theory and Practice
This project will extend and innovate, both theoretically and practically, interpretable methods in AI that are transparent and explainable to improve trust and usability. It will also explore novel approaches for uncertainty quantification and understanding causality.
-
Exploring Predictivity--Parsimony Trade-off In Scientific Machine Learning
This project will investigate, both theoretically and empirically, novel statistical techniques to explore the trade-offs between high-generalization performance and low-model complexity for scientific machine learning.
-
Novel Machine Learning Models for Scientific Discovery
To extend the application range of machine learning to scientific domains, this project will design, analyze and implement novel machine learning techniques that learn from data, while conform with known properties of the underlying scientific models.
-
Automated Discovery of Optimization and Linear Algebra Algorithms
Using reinforcement learning to automate algorithmic discovery, this project aims to develop novel variants of first- and second-order optimization methods, randomized numerical linear algebra techniques, and mixed-integer programming approaches.
-
Second-order Optimization Algorithms for Machine Learning
This project aims to develop the next generation of second-order optimization methods for training complex machine learning models, with particular focus on constrained problems arising in scientific machine learning applications.
-
Distributed Optimization Algorithms for Large-scale Machine Learning
This project aims to design, analyze and implement efficient optimization algorithms suitable for distributed computing environments, with focus on large-scale machine learning.
Supervision history
Current supervision
-
Doctor Philosophy
Stochastic Simulation and Optimization Methods for Machine Learning
Principal Advisor
-
Doctor Philosophy
AI/ML Framework for Mixed-integer Nonlinear Optimisation
Principal Advisor
-
Doctor Philosophy
Interpretable AI-Theory and Practice
Principal Advisor
Other advisors: Dr Quan Nguyen
-
Doctor Philosophy
Novel Machine Learning Models for Scientific Discovery
Principal Advisor
-
Doctor Philosophy
Faithful-Newton Framework: Bridging between Inner and Outer Solvers
Principal Advisor
Other advisors: Associate Professor Marcus Gallagher
-
Doctor Philosophy
Newton type methods for constrained optimization
Principal Advisor
-
Doctor Philosophy
Offline Reinforcement Learning Theory and Algorithms
Associate Advisor
Other advisors: Dr Nan Ye
-
Doctor Philosophy
Forecasting the Market Capitalisation of ASX Listed Junior Resource Companies through an Artificial Neural Network
Associate Advisor
Other advisors: Associate Professor Mehmet Kizil, Dr Micah Nehring
Completed supervision
-
2023
Doctor Philosophy
Newton-MR Methods for Non-convex Smooth Unconstrained Optimizations
Principal Advisor
-
2022
Doctor Philosophy
Efficient second-order optimisation methods for large scale machine learning
Principal Advisor
Other advisors: Associate Professor Marcus Gallagher
-
2022
Doctor Philosophy
Discounting-free Policy Gradient Reinforcement Learning from Transient States
Associate Advisor
Other advisors: Associate Professor Marcus Gallagher
-
2020
Doctor Philosophy
Results on Infinitely Wide Multi-layer Perceptrons
Associate Advisor
Other advisors: Associate Professor Marcus Gallagher
-
2018
Doctor Philosophy
Advances in Monte Carlo Methodology
Associate Advisor
Other advisors: Dr Slava Vaisman
Media
Enquiries
For media enquiries about Professor Fred Roosta's areas of expertise, story ideas and help finding experts, contact our Media team: