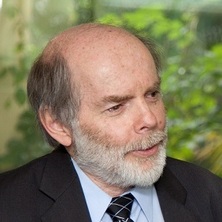
Overview
Background
Professor Geoffrey McLachlan's research interests are in: data mining, statistical analysis of microarray, gene expression data, finite mixture models and medical statistics.
Professor McLachlan received his PhD from the University of Queensland in 1974 and his DSc from there in 1994. His current research projects in statistics are in the related fields of classification, cluster and discriminant analyses, image analysis, machine learning, neural networks, and pattern recognition, and in the field of statistical inference. The focus in the latter field has been on the theory and applications of finite mixture models and on estimation via the EM algorithm.
A common theme of his research in these fields has been statistical computation, with particular attention being given to the computational aspects of the statistical methodology. This computational theme extends to Professor McLachlan's more recent interests in the field of data mining.
He is also actively involved in research in the field of medical statistics and, more recently, in the statistical analysis of microarray gene expression data.
Availability
- Professor Geoffrey McLachlan is:
- Available for supervision
- Media expert
Fields of research
Qualifications
- Bachelor (Honours) of Science (Advanced), The University of Queensland
- Doctor of Philosophy, The University of Queensland
- Doctoral Diploma of Science (Advanced), The University of Queensland
- Australian Mathematical Society, Australian Mathematical Society
Works
Search Professor Geoffrey McLachlan’s works on UQ eSpace
2016
Journal Article
A block minorization-maximization algorithm for heteroscedastic regression
Nguyen, Hien D., Lloyd-Jones, Luke R. and McLachlan, Geoffrey J. (2016). A block minorization-maximization algorithm for heteroscedastic regression. IEEE Signal Processing Letters, 23 (8) 7501879, 1131-1135. doi: 10.1109/LSP.2016.2586180
2016
Journal Article
Finite mixtures of canonical fundamental skew t-distributions: The unification of the restricted and unrestricted skew t-mixture models
Lee, Sharon X and McLachlan, Geoffrey J (2016). Finite mixtures of canonical fundamental skew t-distributions: The unification of the restricted and unrestricted skew t-mixture models. Statistics and Computing, 26 (3), 573-589. doi: 10.1007/s11222-015-9545-x
2016
Journal Article
Laplace mixture autoregressive models
Nguyen, Hien D., McLachlan, Geoffrey J., Ullmann, Jeremy F. P. and Janke, Andrew L. (2016). Laplace mixture autoregressive models. Statistics and Probability Letters, 110, 18-24. doi: 10.1016/j.spl.2015.11.006
2016
Journal Article
A benchmark for evaluation of algorithms for identification of cellular correlates of clinical outcomes
Aghaeepour, Nima, Chattopadhyay, Pratip, Chikina, Maria, Dhaene, Tom, Van Gassen, Sofie, Kursa, Miron, Lambrecht, Bart N., Malek, Mehrnoush, McLachlan, G. J., Qian, Yu, Qiu, Peng, Saeys, Yvan, Stanton, Rick, Tong, Dong, Vens, Celine, Walkowiak, Slawomir, Wang, Kui, Finak, Greg, Gottardo, Raphael, Mosmann, Tim, Nolan, Garry P., Scheuermann, Richard H. and Brinkman, Ryan R. (2016). A benchmark for evaluation of algorithms for identification of cellular correlates of clinical outcomes. Cytometry Part A, 89 (1), 16-21. doi: 10.1002/cyto.a.22732
2016
Journal Article
Mixtures of spatial spline regressions for clustering and classification
Nguyen, Hien D., McLachlan, Geoffrey J. and Wood, Ian A. (2016). Mixtures of spatial spline regressions for clustering and classification. Computational Statistics and Data Analysis, 93, 76-85. doi: 10.1016/j.csda.2014.01.011
2016
Journal Article
Extending mixtures of factor models using the restricted multivariate skew-normal distribution
Lin, Tsung-I, McLachlan, Geoffrey J. and Lee, Sharon X. (2016). Extending mixtures of factor models using the restricted multivariate skew-normal distribution. Journal of Multivariate Analysis, 143, 398-413. doi: 10.1016/j.jmva.2015.09.025
2016
Conference Publication
Unsupervised component-wise EM learning for finite mixtures of skew t-distributions
Lee, Sharon X. and McLachlan, Geoffrey J. (2016). Unsupervised component-wise EM learning for finite mixtures of skew t-distributions. 12th International Conference, ADMA 2016, Gold Coast, QLD, Australia, 12-15 December 2016. New York, NY, United States: Springer. doi: 10.1007/978-3-319-49586-6_49
2016
Conference Publication
On mixture modelling with multivariate skew distributions
Lee, Sharon X. and McLachlan, Geoffrey J. (2016). On mixture modelling with multivariate skew distributions. COMPSTAT: International Conference on Computational Statistics, Oviedo, Spain, 23-26 August 2016. The Hague, The Netherlands: The International Statistical Institute/International Association for Statistical Computing.
2016
Conference Publication
A simple parallel EM algorithm for statistical learning via mixture models
Lee, Sharon X., Leemaqz, Kaleb L. and McLachlan, Geoffrey J. (2016). A simple parallel EM algorithm for statistical learning via mixture models. International Conference on Digital Image Computing, Gold Coast, QLD, Australia, 30 November - 2 December,2016. Piscataway, NJ, United States: IEEE (Institute for Electrical and Electronic Engineers). doi: 10.1109/DICTA.2016.7796997
2016
Book Chapter
Application of mixture models to large datasets
Lee, Sharon X., McLachlan, Geoffrey J. and Pyne, Saumyadipta (2016). Application of mixture models to large datasets. Big data analytics: methods and applications. (pp. 57-74) edited by Saumyadipta Pyne, B. L. S. Prakasa Rao and S. B. Rao. New Delhi, India: Springer India. doi: 10.1007/978-81-322-3628-3_4
2016
Journal Article
Laplace mixture of linear experts
Nguyen, Hien D. and McLachlan, Geoffrey J. (2016). Laplace mixture of linear experts. Computational Statistics and Data Analysis, 93, 177-191. doi: 10.1016/j.csda.2014.10.016
2016
Journal Article
Modeling of inter-sample variation in flow cytometric data with the joint clustering and matching procedure
Lee, Sharon X., McLachlan, Geoffrey J. and Pyne, Saumyadipta (2016). Modeling of inter-sample variation in flow cytometric data with the joint clustering and matching procedure. Cytometry Part A, 89 (1), 30-43. doi: 10.1002/cyto.a.22789
2016
Book Chapter
Mixture models for standard p-dimensional Euclidean data
McLachlan, Geoffrey J. and Rathnayake, Suren I. (2016). Mixture models for standard p-dimensional Euclidean data. Handbook of cluster analysis. (pp. 145-171) edited by Christian Hennig, Marina Meila, Fionn Murtagh and Roberto Rocci. Boca Raton, FL, United States: CRC Press. doi: 10.1201/b19706-14
2016
Conference Publication
Robust estimation of mixtures of skew-normal distributions
García-Escudero, L. A., Greselin, F., Mayo-Iscar, A. and McLachlan, G. J. (2016). Robust estimation of mixtures of skew-normal distributions. Scientific Meeting of the Italian Statistical Society, Salerno, Italy, 8-10 November 2016. Fisciano, Italy: Dipartimento di Scienze Economiche e Statistiche, University of Salerno..
2016
Book Chapter
Mixture distributions - further developments
McLachlan, Geoffrey J. (2016). Mixture distributions - further developments. Wiley statsref: statistics reference online. (pp. 1-13) Chichester, United Kingdom: John Wiley & Sons. doi: 10.1002/9781118445112.stat00947.pub2
2016
Conference Publication
Finding group structures in "Big Data" in healthcare research using mixture models
Ng, Shu-Kay and McLachlan, Geoffrey J. (2016). Finding group structures in "Big Data" in healthcare research using mixture models. IEEE International Conference on Bioinformatics and Biomedicine, Shenzhen, China, 15-18 December 2016. Piscataway, NJ, United States: IEE Computer Society. doi: 10.1109/BIBM.2016.7822692
2015
Journal Article
Application of multiple imputation for missing values in three-way three-mode multi-environment trial data
Tian, Ting, McLachlan, Geoffrey J., Dieter, Mark J. and Basford, Kaye E. (2015). Application of multiple imputation for missing values in three-way three-mode multi-environment trial data. PLoS One, 10 (12) e0144370, e0144370.1-e0144370.25. doi: 10.1371/journal.pone.0144370
2015
Journal Article
Special issue on "New trends on model-based clustering and classification"
Ingrassia, Salvatore, McLachlan, Geoffrey J. and Govaert, Gerard (2015). Special issue on "New trends on model-based clustering and classification". Advances in Data Analysis and Classification, 9 (4), 367-369. doi: 10.1007/s11634-015-0224-8
2015
Edited Outputs
Advances in Data Analysis and Classification
Advances in Data Analysis and Classification. (2015). 9 (4)
2015
Journal Article
Maximum likelihood estimation of Gaussian mixture models without matrix operations
Nguyen, Hien D. and McLachlan, Geoffrey J. (2015). Maximum likelihood estimation of Gaussian mixture models without matrix operations. Advances in Data Analysis and Classification, 9 (4), 371-394. doi: 10.1007/s11634-015-0209-7
Funding
Current funding
Past funding
Supervision
Availability
- Professor Geoffrey McLachlan is:
- Available for supervision
Before you email them, read our advice on how to contact a supervisor.
Supervision history
Current supervision
-
Doctor Philosophy
Role of Finite Mixture Models in Semi-Supervised Learning
Principal Advisor
Other advisors: Dr Sharon Lee
-
Doctor Philosophy
Using statistical approaches to gain insight into patterns of variation in melanoma transcriptomic data
Associate Advisor
Other advisors: Dr Vivi Arief, Dr Quan Nguyen, Emeritus Professor Kaye Basford
-
Doctor Philosophy
Learning a mineralised fault network at the Cracow Gold Mine from geologically-informed 3D synthetic seismic data
Associate Advisor
Other advisors: Dr Dion Weatherley, Professor Rick Valenta
Completed supervision
-
2024
Doctor Philosophy
Detecting the unexpected in astronomical data using complexity based approaches
Principal Advisor
-
2023
Doctor Philosophy
Improving Predictability of Minerals Processing Models by Developing a Methodology based-on Machine Learning Techniques
Principal Advisor
-
-
2015
Doctor Philosophy
Finite Mixture Models for Regression Problems
Principal Advisor
Other advisors: Dr Ian Wood
-
2014
Doctor Philosophy
Finite Mixture Modelling using Multivariate Skew Distributions
Principal Advisor
Other advisors: Dr Ian Wood
-
2012
Doctor Philosophy
Detection of Differentially Expressed Genes via Mixture Models and Cluster Analysis
Principal Advisor
Other advisors: Dr Ian Wood
-
2009
Doctor Philosophy
Statistical analysis of high-dimensional gene expression data
Principal Advisor
-
2005
Doctor Philosophy
CLUSTERING WITH MIXED VARIABLES
Principal Advisor
-
2004
Master Science
Modelling the statistical behaviour of temperature using a modified Brennan and Schwartz 1982 interest rate model
Principal Advisor
Other advisors: Associate Professor Michael Bulmer
-
2024
Doctor Philosophy
The Wealth of Features: towards a coherent cooperative game theory for feature importance
Associate Advisor
Other advisors: Associate Professor Sally Shrapnel, Dr Ian Wood
-
2019
Doctor Philosophy
Maximum pseudolikelihood estimation with Markov random fields in the segmentation of brain magnetic resonance images
Associate Advisor
Other advisors: Dr Ian Wood
-
-
2016
Doctor Philosophy
Estimation of missing values in multivariate multi-environment trial data for three-way pattern analysis
Associate Advisor
Other advisors: Emeritus Professor Kaye Basford
-
-
Doctor Philosophy
TOPOLOGICAL MODELS OF TRANSMEMBRANE PROTEINS FOR SUBCELLULAR LOCALIZATION PREDICTION
Associate Advisor
Other advisors: Associate Professor Marcus Gallagher, Professor Mikael Boden
Media
Enquiries
Contact Professor Geoffrey McLachlan directly for media enquiries about:
- Bioinformatics
- Computation - statistics
- Computer learning
- Data mining
- Gene expression data
- Image analysis - statistics
- Machine learning
- Neural networks
- Pattern recognition - statistics
- Statistical methodology
- Statistics
Need help?
For help with finding experts, story ideas and media enquiries, contact our Media team: