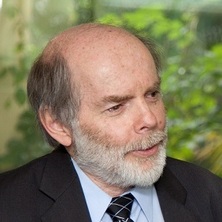
Overview
Background
Professor Geoffrey McLachlan's research interests are in: data mining, statistical analysis of microarray, gene expression data, finite mixture models and medical statistics.
Professor McLachlan received his PhD from the University of Queensland in 1974 and his DSc from there in 1994. His current research projects in statistics are in the related fields of classification, cluster and discriminant analyses, image analysis, machine learning, neural networks, and pattern recognition, and in the field of statistical inference. The focus in the latter field has been on the theory and applications of finite mixture models and on estimation via the EM algorithm.
A common theme of his research in these fields has been statistical computation, with particular attention being given to the computational aspects of the statistical methodology. This computational theme extends to Professor McLachlan's more recent interests in the field of data mining.
He is also actively involved in research in the field of medical statistics and, more recently, in the statistical analysis of microarray gene expression data.
Availability
- Professor Geoffrey McLachlan is:
- Available for supervision
- Media expert
Fields of research
Qualifications
- Bachelor (Honours) of Science (Advanced), The University of Queensland
- Doctor of Philosophy, The University of Queensland
- Doctoral Diploma of Science (Advanced), The University of Queensland
- Australian Mathematical Society, Australian Mathematical Society
Works
Search Professor Geoffrey McLachlan’s works on UQ eSpace
2021
Journal Article
Utilising a deep neural network as a surrogate model to approximate phenomenological models of a comminution circuit for faster simulations
Koh, Edwin J.Y., Amini, Eiman, McLachlan, Geoffrey J. and Beaton, Nick (2021). Utilising a deep neural network as a surrogate model to approximate phenomenological models of a comminution circuit for faster simulations. Minerals Engineering, 170 107026, 1-11. doi: 10.1016/j.mineng.2021.107026
2021
Journal Article
Data fusion using factor analysis and low-rank matrix completion
Ahfock, Daniel, Pyne, Saumyadipta and McLachlan, Geoffrey J. (2021). Data fusion using factor analysis and low-rank matrix completion. Statistics and Computing, 31 (5) 58. doi: 10.1007/s11222-021-10033-7
2021
Journal Article
Multi‐node expectation–maximization algorithm for finite mixture models
Lee, Sharon X., McLachlan, Geoffrey J. and Leemaqz, Kaleb L. (2021). Multi‐node expectation–maximization algorithm for finite mixture models. Statistical Analysis and Data Mining: The ASA Data Science Journal, 14 (4) sam.11529, 297-304. doi: 10.1002/sam.11529
2021
Journal Article
Bayesian analysis of generalized linear mixed models with spatial correlated and unrestricted skew normal errors
Farzammehr, M. A., Mohammadzadeh, M, Zadkarami, M. R. and McLachlan, G. J. (2021). Bayesian analysis of generalized linear mixed models with spatial correlated and unrestricted skew normal errors. Communications in Statistics: Theory and Methods, 51 (24), 1-22. doi: 10.1080/03610926.2021.1897843
2021
Journal Article
Harmless label noise and informative soft-labels in supervised classification
Ahfock, Daniel and McLachlan, Geoffrey J. (2021). Harmless label noise and informative soft-labels in supervised classification. Computational Statistics and Data Analysis, 161 107253, 107253. doi: 10.1016/j.csda.2021.107253
2021
Conference Publication
Extending FaultSeg3D to Minerals Seismic: Part 1 – A synthetic 3D-seismic training-volume generator for preparing data replicating a hardrock terrane to train an automatic-fault-prediction algorithm
Chatterjee, Robindra , Valenta, Richard , McLachlan, Geoffrey and Weatherley, Dion (2021). Extending FaultSeg3D to Minerals Seismic: Part 1 – A synthetic 3D-seismic training-volume generator for preparing data replicating a hardrock terrane to train an automatic-fault-prediction algorithm. Australian Earth Science Convention, Virtual, 9-12 February 2021.
2021
Conference Publication
On Mean And/or Variance Mixtures of Normal Distributions
Lee, Sharon X. and McLachlan, Geoffrey J. (2021). On Mean And/or Variance Mixtures of Normal Distributions. 12th Scientific Meeting of the Classification and Data Analysis Group of the Italian Statistical Society (CLADAG 2019), Cassino, Italy, 11–13 September 2019. Cham, Switzerland: Springer. doi: 10.1007/978-3-030-69944-4_13
2021
Journal Article
On formulations of skew factor models: Skew factors and/or skew errors
Lee, Sharon X. and McLachlan, Geoffrey J. (2021). On formulations of skew factor models: Skew factors and/or skew errors. Statistics and Probability Letters, 168 108935, 108935. doi: 10.1016/j.spl.2020.108935
2021
Book Chapter
Automated gating and dimension reduction of high-dimensional cytometry data
Lee, Sharon X., McLachlan, Geoffrey J. and Pyne, Saumyadipta (2021). Automated gating and dimension reduction of high-dimensional cytometry data. Mathematical, computational and experimental T cell immunology. (pp. 281-294) edited by Carmen Molina-París and Grant Lythe . Cham, Switzerland: Springer. doi: 10.1007/978-3-030-57204-4_16
2021
Book Chapter
Estimation of classification rules from partially classified data
McLachlan, Geoffrey and Ahfock, Daniel (2021). Estimation of classification rules from partially classified data. Data analysis and rationality in a complex world. (pp. 149-157) edited by Theodore Chadjipadelis, Berthold Lausen, Angelos Markos, Tae Rim Lee, Angela Montanari and Rebecca Nugent. Cham, Switzerland: Springer. doi: 10.1007/978-3-030-60104-1_17
2020
Journal Article
An apparent paradox: a classifier based on a partially classified sample may have smaller expected error rate than that if the sample were completely classified
Ahfock, Daniel and McLachlan, Geoffrey J. (2020). An apparent paradox: a classifier based on a partially classified sample may have smaller expected error rate than that if the sample were completely classified. Statistics and Computing, 30 (6), 1779-1790. doi: 10.1007/s11222-020-09971-5
2020
Journal Article
Mixtures of factor analyzers with scale mixtures of fundamental skew normal distributions
Lee, Sharon X., Lin, Tsung-I and McLachlan, Geoffrey J. (2020). Mixtures of factor analyzers with scale mixtures of fundamental skew normal distributions. Advances in Data Analysis and Classification, 15 (2), 481-512. doi: 10.1007/s11634-020-00420-9
2020
Journal Article
A Mixture of Regressions Model of COVID-19 Death Rates and Population Comorbidities
Maleki, M. , McLachlan, G. J. , Gurewitsch, R. , Aruru, M. and Pyne, S. (2020). A Mixture of Regressions Model of COVID-19 Death Rates and Population Comorbidities. Statistics and Applications, 18 (1), 295-306.
2020
Journal Article
Approximation by finite mixtures of continuous density functions that vanish at infinity
Nguyen, T. Tin, Nguyen, Hien D., Chamroukhi, Faicel and McLachlan, Geoffrey J. (2020). Approximation by finite mixtures of continuous density functions that vanish at infinity. Cogent Mathematics and Statistics, 7 (1). doi: 10.1080/25742558.2020.1750861
2020
Journal Article
Mini-batch learning of exponential family finite mixture models
Nguyen, Hien D., Forbes, Florence and McLachlan, Geoffrey J. (2020). Mini-batch learning of exponential family finite mixture models. Statistics and Computing, 30 (4), 731-748. doi: 10.1007/s11222-019-09919-4
2020
Journal Article
A bivariate joint frailty model with mixture framework for survival analysis of recurrent events with dependent censoring and cure fraction
Tawiah, Richard, McLachlan, Geoffrey J. and Ng, Shu Kay (2020). A bivariate joint frailty model with mixture framework for survival analysis of recurrent events with dependent censoring and cure fraction. Biometrics, 76 (3) biom.13202, 753-766. doi: 10.1111/biom.13202
2020
Conference Publication
Modelling asset return using multivariate asymmetric mixture models with applications to estimation of Value-at-Risk
Lee, Sharon X. and McLachlan, Geoffrey J. (2020). Modelling asset return using multivariate asymmetric mixture models with applications to estimation of Value-at-Risk. 20th International Congress on Modelling and Simulation - Adapting to Change: The Multiple Roles of Modelling, MODSIM 2013 , Adelaide, SA, Australia, 1 - 6 December 2013. Modelling and Simulation Society of Australia and New Zealand Inc. (MSSANZ).
2020
Book Chapter
Comprehensive chemometrics: chemical and biochemical data analysis
McLachlan, G. J., Rathnayake, S. and Lee, S. X. (2020). Comprehensive chemometrics: chemical and biochemical data analysis. Comprehensive chemometrics: chemical and biochemical data analysis. (pp. 267-304) edited by Steven Brown, Roma Tauler and Beata Walczak. Oxford, United Kingdom: Elsevier.
2019
Journal Article
On approximations via convolution-defined mixture models
Nguyen, Hien D. and McLachlan, Geoffrey (2019). On approximations via convolution-defined mixture models. Communications in Statistics - Theory and Methods, 48 (16), 3945-3955. doi: 10.1080/03610926.2018.1487069
2019
Journal Article
False discovery rate control for grouped or discretely supported p-values with application to a neuroimaging study
Nguyen, Hien D., Yee, Yohan, McLachlan, Geoffrey J. and Lerch, Jason P. (2019). False discovery rate control for grouped or discretely supported p-values with application to a neuroimaging study. SORT, 43 (2), 1-22. doi: 10.2436/20.8080.02.87
Funding
Current funding
Past funding
Supervision
Availability
- Professor Geoffrey McLachlan is:
- Available for supervision
Before you email them, read our advice on how to contact a supervisor.
Supervision history
Current supervision
-
Doctor Philosophy
Role of Finite Mixture Models in Semi-Supervised Learning
Principal Advisor
Other advisors: Dr Sharon Lee
-
Doctor Philosophy
Learning a mineralised fault network at the Cracow Gold Mine from geologically-informed 3D synthetic seismic data
Associate Advisor
Other advisors: Dr Dion Weatherley, Professor Rick Valenta
-
Doctor Philosophy
Using statistical approaches to gain insight into patterns of variation in melanoma transcriptomic data
Associate Advisor
Other advisors: Dr Vivi Arief, Dr Quan Nguyen, Emeritus Professor Kaye Basford
Completed supervision
-
2024
Doctor Philosophy
Detecting the unexpected in astronomical data using complexity based approaches
Principal Advisor
-
2023
Doctor Philosophy
Improving Predictability of Minerals Processing Models by Developing a Methodology based-on Machine Learning Techniques
Principal Advisor
-
-
2015
Doctor Philosophy
Finite Mixture Models for Regression Problems
Principal Advisor
Other advisors: Dr Ian Wood
-
2014
Doctor Philosophy
Finite Mixture Modelling using Multivariate Skew Distributions
Principal Advisor
Other advisors: Dr Ian Wood
-
2012
Doctor Philosophy
Detection of Differentially Expressed Genes via Mixture Models and Cluster Analysis
Principal Advisor
Other advisors: Dr Ian Wood
-
2009
Doctor Philosophy
Statistical analysis of high-dimensional gene expression data
Principal Advisor
-
2005
Doctor Philosophy
CLUSTERING WITH MIXED VARIABLES
Principal Advisor
-
2004
Master Science
Modelling the statistical behaviour of temperature using a modified Brennan and Schwartz 1982 interest rate model
Principal Advisor
Other advisors: Associate Professor Michael Bulmer
-
2024
Doctor Philosophy
The Wealth of Features: towards a coherent cooperative game theory for feature importance
Associate Advisor
Other advisors: Associate Professor Sally Shrapnel, Dr Ian Wood
-
2019
Doctor Philosophy
Maximum pseudolikelihood estimation with Markov random fields in the segmentation of brain magnetic resonance images
Associate Advisor
Other advisors: Dr Ian Wood
-
-
2016
Doctor Philosophy
Estimation of missing values in multivariate multi-environment trial data for three-way pattern analysis
Associate Advisor
Other advisors: Emeritus Professor Kaye Basford
-
-
Doctor Philosophy
TOPOLOGICAL MODELS OF TRANSMEMBRANE PROTEINS FOR SUBCELLULAR LOCALIZATION PREDICTION
Associate Advisor
Other advisors: Associate Professor Marcus Gallagher, Professor Mikael Boden
Media
Enquiries
Contact Professor Geoffrey McLachlan directly for media enquiries about:
- Bioinformatics
- Computation - statistics
- Computer learning
- Data mining
- Gene expression data
- Image analysis - statistics
- Machine learning
- Neural networks
- Pattern recognition - statistics
- Statistical methodology
- Statistics
Need help?
For help with finding experts, story ideas and media enquiries, contact our Media team: