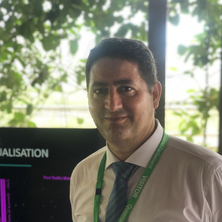
Overview
Background
Opportunities for Students
I am keen to supervise motivated postgraduate and PhD students who are passionate about AI, cybersecurity, or networking. My research group offers hands-on projects, including developing AI-driven intrusion detection systems, securing IoT ecosystems, and optimising SDN frameworks. Students will gain experience with state-of-the-art ML tools, collaborate with industry partners, and contribute to high-impact publications. Ideal candidates should have strong programming skills (e.g., Python, C++) and a basic understanding of ML or networking concepts, though enthusiasm and a willingness to learn are equally valued.
Why Join My Group?
My research is inherently interdisciplinary, bridging AI, cybersecurity, and networking to address real-world problems. Students will work on cutting-edge projects with access to UQ’s world-class facilities and opportunities to collaborate with global experts. Whether you’re interested in defending against cyber threats or shaping the future of IoT and SDN, my group provides a dynamic environment to grow as a researcher.
About Me
As a passionate researcher at The University of Queensland, I explore the intersection of Artificial Intelligence (AI) and Machine Learning (ML) with cutting-edge applications in cybersecurity, Internet of Things (IoT), and Software Defined Networking (SDN). My work focuses on developing innovative, real-world solutions to protect digital systems and optimise network performance, mentoring the next generation of researchers to tackle global challenges.
Availability
- Dr Siamak Layeghy is:
- Available for supervision
Qualifications
- Doctor of Philosophy, The University of Queensland
Research interests
-
AI/ML for Cybersecurity
I develop advanced intrusion detection systems (NIDS and HIDS) using techniques like Transformers, Generative Adversarial Networks (GANs), and Transfer Learning to detect and mitigate cyber threats in real time.
-
Edge Learning and IoT Security
My work focuses on lightweight AI models for resource-constrained IoT devices, enabling secure and efficient edge computing.
-
Software Defined Networking (SDN)
I explore network optimisation and programmable data planes (e.g., P4) to enhance Quality of Service (QoS) and constrained routing for next-generation networks.
Research impacts
Research Vision
My research leverages AI and ML to secure and optimise emerging technologies. By combining advanced techniques like Large Language Models (LLMs), Graph Neural Networks (GNNs), and Federated Learning with practical applications, I aim to create robust, scalable systems for network security, edge computing, and programmable networks. My goal is to address pressing challenges in cybersecurity and IoT, ensuring safe and efficient digital ecosystems.
My Google Scholar: https://scholar.google.com.au/citations?user=uB6MlpQAAAAJ&hl=en
Works
Search Professor Siamak Layeghy’s works on UQ eSpace
Featured
2024
Journal Article
FlowTransformer: A transformer framework for flow-based network intrusion detection systems
Manocchio, Liam Daly, Layeghy, Siamak, Lo, Wai Weng, Kulatilleke, Gayan K., Sarhan, Mohanad and Portmann, Marius (2024). FlowTransformer: A transformer framework for flow-based network intrusion detection systems. Expert Systems with Applications, 241 122564, 1-15. doi: 10.1016/j.eswa.2023.122564
Featured
2024
Journal Article
Benchmarking the benchmark — Comparing synthetic and real-world Network IDS datasets
Layeghy, Siamak, Gallagher, Marcus and Portmann, Marius (2024). Benchmarking the benchmark — Comparing synthetic and real-world Network IDS datasets. Journal of Information Security and Applications, 80 103689, 1-18. doi: 10.1016/j.jisa.2023.103689
Featured
2023
Journal Article
Exploring Edge TPU for Network Intrusion Detection in IoT
Hosseininoorbin, Seyedehfaezeh, Layeghy, Siamak, Sarhan, Mohanad, Jurdak, Raja and Portmann, Marius (2023). Exploring Edge TPU for Network Intrusion Detection in IoT. Journal of Parallel and Distributed Computing, 179 104712, 1-11. doi: 10.1016/j.jpdc.2023.05.001
Featured
2023
Journal Article
DI-NIDS: domain invariant network intrusion detection system
Layeghy, Siamak, Baktashmotlagh, Mahsa and Portmann, Marius (2023). DI-NIDS: domain invariant network intrusion detection system. Knowledge-Based Systems, 273 110626, 110626. doi: 10.1016/j.knosys.2023.110626
Featured
2023
Journal Article
Explainable cross-domain evaluation of ML-based network intrusion detection systems
Layeghy, Siamak and Portmann, Marius (2023). Explainable cross-domain evaluation of ML-based network intrusion detection systems. Computers and Electrical Engineering, 108 108692, 1-15. doi: 10.1016/j.compeleceng.2023.108692
Featured
2022
Journal Article
Anomal-E: A self-supervised network intrusion detection system based on graph neural networks
Caville, Evan, Lo, Wai Weng, Layeghy, Siamak and Portmann, Marius (2022). Anomal-E: A self-supervised network intrusion detection system based on graph neural networks. Knowledge-Based Systems, 258 110030, 1-11. doi: 10.1016/j.knosys.2022.110030
Featured
2022
Conference Publication
E-GraphSAGE: a graph neural network based intrusion detection system for IoT
Lo, Wai Weng, Layeghy, Siamak, Sarhan, Mohanad, Gallagher, Marcus and Portmann, Marius (2022). E-GraphSAGE: a graph neural network based intrusion detection system for IoT. NOMS 2022-2022 IEEE/IFIP Network Operations and Management Symposium, Budapest, Hungary, 25-29 April 2022. Piscataway, NJ, United States: Institute of Electrical and Electronics Engineers . doi: 10.1109/noms54207.2022.9789878
Featured
2021
Conference Publication
NetFlow datasets for machine learning-based network intrusion detection systems
Sarhan, Mohanad, Layeghy, Siamak, Moustafa, Nour and Portmann, Marius (2021). NetFlow datasets for machine learning-based network intrusion detection systems. 10th EAI International Conference, BDTA 2020 and 13th EAI International Conference on Wireless Internet, WiCON 2020, Virtual Event, 11 December 2020. Cham, Switzerland: Springer Science and Business Media Deutschland GmbH. doi: 10.1007/978-3-030-72802-1_9
2025
Journal Article
An empirical evaluation of preprocessing methods for machine learning based network intrusion detection systems
Manocchio, Liam Daly, Layeghy, Siamak, Gallagher, Marcus and Portmann, Marius (2025). An empirical evaluation of preprocessing methods for machine learning based network intrusion detection systems. Engineering Applications of Artificial Intelligence, 158 111289, 1-16. doi: 10.1016/j.engappai.2025.111289
2025
Journal Article
EcoShower: estimating shower duration using non-intrusive multi-modal sensor data via LSTM and Gated Transformer models
Sablica, Lukas, Grün, Bettina, Layeghy, Siamak, Dolnicar, Sara and Portmann, Marius (2025). EcoShower: estimating shower duration using non-intrusive multi-modal sensor data via LSTM and Gated Transformer models. Expert Systems with Applications, 277 127202, 127202-277. doi: 10.1016/j.eswa.2025.127202
2025
Journal Article
P4-Secure: in-band DDoS detection in software defined networks
Daly Manocchio, Liam, Chen, Yaying, Layeghy, Siamak, Gwynne, David and Portmann, Marius (2025). P4-Secure: in-band DDoS detection in software defined networks. IEEE Transactions on Network and Service Management, 22 (2), 2120-2137. doi: 10.1109/tnsm.2025.3552844
2025
Journal Article
Leveraging LSTM and reinforcement learning for adaptive sensing in CIoT nodes
Ghosh, Sushmita, Layeghy, Siamak, De, Swades, Chatterjee, Shouri and Portmann, Marius (2025). Leveraging LSTM and reinforcement learning for adaptive sensing in CIoT nodes. IEEE Transactions on Consumer Electronics, 71 (1), 178-188. doi: 10.1109/tce.2024.3516498
2025
Other Outputs
NF-UNSW-NB15-v3
Luay, Majed, Layeghy, Siamak, Mohanad, Sarhan, Sayedehfaezeh, Hoseininoorbin, Moustafa, Nour and Portmann, Marius (2025). NF-UNSW-NB15-v3. The University of Queensland. (Dataset) doi: 10.48610/6e0eda1
2025
Other Outputs
NF-CSE-CIC-IDS2018-v3
Luay, Majed, Layeghy, Siamak, Mohanad, Sarhan, Sayedehfaezeh, Hoseininoorbin, Moustafa, Nour and Portmann, Marius (2025). NF-CSE-CIC-IDS2018-v3. The University of Queensland. (Dataset) doi: 10.48610/ece9b83
2025
Other Outputs
NF-BoT-IoT-v3
Luay, Majed, Layeghy, Siamak, Mohanad, Sarhan, Sayedehfaezeh, Hoseininoorbin, Nour, Moustafa and Portmann, Marius (2025). NF-BoT-IoT-v3. The University of Queensland. (Dataset) doi: 10.48610/73c4ebc
2025
Other Outputs
NF-ToN-IoT-v3
Luay, Majed, Layeghy, Siamak, Mohanad, Sarhan, Sayedehfaezeh, Hoseininoorbin, Moustafa, Nour and Portmann, Marius (2025). NF-ToN-IoT-v3. The University of Queensland. (Dataset) doi: 10.48610/44d7c5e
2024
Conference Publication
Towards Explainable Network Intrusion Detection using Large Language Models
Houssel, Paul R. B., Singh, Priyanka, Layeghy, Siamak and Portmann, Marius (2024). Towards Explainable Network Intrusion Detection using Large Language Models. 2024 IEEE/ACM International Conference on Big Data Computing, Applications and Technologies (BDCAT), Sharjah, United Arab Emirates, 16-19 December 2024. Piscataway, NJ United States: Institute of Electrical and Electronics Engineers. doi: 10.1109/bdcat63179.2024.00021
2024
Journal Article
FlowTransformer: A flexible python framework for flow-based network data analysis
Manocchio, Liam Daly, Layeghy, Siamak and Portmann, Marius (2024). FlowTransformer: A flexible python framework for flow-based network data analysis. Software Impacts, 22 100702, 100702. doi: 10.1016/j.simpa.2024.100702
2024
Journal Article
Does every hotel room need a minifridge? Empirical evidence from consumer self-reports and an automatic sensor-based system measuring electricity consumption and guest use
Dolnicar, Sara, Greene, Danyelle, Layeghy, Siamak and Portmann, Marius (2024). Does every hotel room need a minifridge? Empirical evidence from consumer self-reports and an automatic sensor-based system measuring electricity consumption and guest use. Annals of Tourism Research Empirical Insights, 5 (2) 100134. doi: 10.1016/j.annale.2024.100134
2024
Journal Article
A configurable anonymisation approach for network flow data: Balancing utility and privacy
Manocchio, Liam Daly, Layeghy, Siamak, Gwynne, David and Portmann, Marius (2024). A configurable anonymisation approach for network flow data: Balancing utility and privacy. Computers and Electrical Engineering, 118 109465, 1-16. doi: 10.1016/j.compeleceng.2024.109465
Funding
Current funding
Past funding
Supervision
Availability
- Dr Siamak Layeghy is:
- Available for supervision
Before you email them, read our advice on how to contact a supervisor.
Available projects
-
Machine Learning for Computer Networking
This project aims to harness Machine Learning and AI techniques, with a focus on Large Language Models, for the configuration and management of Computer Networks.
Your application will be assessed on a competitive basis.
We take into account your:
- previous academic record
- publication record
- honours and awards
- employment history
A working knowledge of AI, software engineering and data science would be of benefit to someone working on this project.
You will demonstrate academic achievement in the field/s of computer networking and machine learning and the potential for scholastic success.
A background or knowledge of Large Language Models (LLMs) is highly desirable. You apply for this scholarship when you submit an application for your program. You don’t need to submit a separate scholarship application.
Supervision history
Current supervision
-
Doctor Philosophy
Enhancing the Privacy-Preserving ML techniques with Functional Encryption approach
Principal Advisor
Other advisors: Professor Marius Portmann
-
Doctor Philosophy
Machine Learning for Improving Services and Management of Software Defined Networks
Principal Advisor
Other advisors: Professor Marius Portmann
-
Doctor Philosophy
Enhancing Cyberbullying Detection in Arabic Social Media through Explainable AI and Natural Language Processing: A Human-Centric Approach
Principal Advisor
Other advisors: Professor Marius Portmann
-
Doctor Philosophy
Towards Practical Machine Learning Based Network Intrusion Detection
Associate Advisor
Other advisors: Associate Professor Marcus Gallagher, Professor Marius Portmann
-
Doctor Philosophy
Low-energy LoRaWAN-based automatic and continuous measurement of organisational environmental performance.
Associate Advisor
Other advisors: Professor Sara Dolnicar, Professor Marius Portmann
-
Doctor Philosophy
eXtended Management Network System (xNMS)
Associate Advisor
Other advisors: Professor Marius Portmann
-
Doctor Philosophy
Exploring the Capabilities of LoRaWAN IoT Technology for Multisensor Data Collection and Analysis
Associate Advisor
Other advisors: Professor Sara Dolnicar, Professor Marius Portmann
Completed supervision
-
2023
Doctor Philosophy
Deep Learning at the Edge: Exploring in-situ Classification in IoT
Associate Advisor
Other advisors: Professor Marius Portmann
-
2023
Doctor Philosophy
The Detection of Network Cyber Attacks Using Machine Learning
Associate Advisor
Other advisors: Associate Professor Marcus Gallagher, Professor Marius Portmann
-
2023
Master Philosophy
Graph Representation Learning for Cyberattack Detection and Forensics
Associate Advisor
Other advisors: Associate Professor Marcus Gallagher, Professor Marius Portmann
Media
Enquiries
For media enquiries about Dr Siamak Layeghy's areas of expertise, story ideas and help finding experts, contact our Media team: