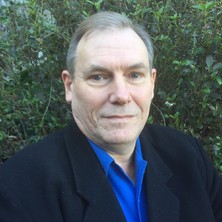
Overview
Background
Brian C. Lovell, born in Brisbane, Australia in 1960, received his BE in Electrical Engineering (Honours I) in 1982, BSc in Computer Science in 1983, and PhD in Signal Processing in 1991, all from the University of Queensland (UQ). Currently, he is the Project Leader of the Advanced Surveillance Group at UQ. Professor Lovell served as the President of the International Association of Pattern Recognition from 2008 to 2010, is a Senior Member of the IEEE, a Fellow of the IEAust, Fellow of the Asia-Pacific AI Association, and has been a voting member for Australia on the Governing Board of the International Association for Pattern Recognition since 1998.
He is an Honorary Professor at IIT Guwahati, India; an Associate Editor of the Pattern Recognition Journal; an Associate Editor-in-Chief of the Machine Learning Research Journal; a member of the IAPR TC4 on Biometrics; and a member of the Awards Committee and Education Committee of the IEEE Biometrics Council.
In addition, Professor Lovell has chaired and co-chaired numerous international conferences in the field of pattern recognition, including ICPR2008, ACPR2011, ICIP2013, ICPR2016, and ICPR2020. His Advanced Surveillance Group has collaborated with port, rail, and airport organizations, as well as several national and international agencies, to develop technology-based solutions for operational and security concerns.
His current research projects are in the fields of:
- Artificial Intelligence
- StyleGAN
- Stable Diffusion
- Deep Learning
- Biometrics
- Robust Face Recognition using Deep Learning
- Masked Face Recognition for COVID-19 Pandemic
- Adversarial Attacks on AI Systems
- Digital Pathology
- Neurofibroma Detection and Assessment
- Object Detection with Deep Learning
I am actively recruiting PhD students in Artificial Intelligence to work with my team. If you are interested and have a strong record from a good university, with a publication in a good conference such as CVPR, ICCV, ECCV, or MICCAI please send your CV to me. Full Scholarships (Tuition and Living) can be awarded within one month for truly exceptional candidates.
Availability
- Professor Brian Lovell is:
- Available for supervision
- Media expert
Fields of research
Qualifications
- Bachelor (Honours) of Engineering, The University of Queensland
- Bachelor of Science, The University of Queensland
- Doctor of Philosophy, The University of Queensland
Research interests
-
Face Recognition with Deep Learning
We develop new technologies to improve face recognition. Our group is first in the world to develop face recognition databases based entirely on synthetic faces. Other aspects of face recognition and affective computing (determining emotions from facial expressions) are current research themes within the group.
-
Object Detection Using Deep Learning
We are researching improved techniques to identify small objects with high precision
-
Synthetic Face and Image Generation
We were the first to investigate training face recognition systems on synthetic faces.
Research impacts
I have been pleased that my biometrics and other research has and is being been adopted commercially worldwide. My earlier face recognition systems have been installed by the University of San Francisco and Swinburne University among many other sites. More recently we have developed face recognition systems that are insensitive to the wearing of masks. These systems depend on our EDITH Ethical Face database of synthetic faces. To the best of our knowledge, we are the only group worldwide who can synthesise faces to order to train advanced ethical face recognition systems.
These systems have been adopted in the UK in 2020 by Facewatch Ltd and are currently being considered by the UK National Health Service and also Queensland Health to manage COVID 19 quarantine facilities and border control. In 2020-2021 we developed a touchless face mask fitting system for health workers to reduce the wastage of PPE and improve COVID19 management. This system is deployed on Queensland Health IT infrastructure in February 2021 and is planned to be made available nationally and internationally. The system has the potential to save millions of dollars in wasted PPE.
PRIZES, HONOURS AND AWARDS
Fellow of the IAPR, 2008 Multiple Best Paper prizes. Awarded Certificate of Recognition as most downloaded author at UQ by UQCybrary. Over 26,000 copies of my research papers were downloaded from the UQ EPrints archive in the 12 months ending May, 2005. APICTA Trophy for Best Research and Development, 2011, Face Recognition in a Crowd IFSEC Trophy 2011, Best CCTV Product of the Year (excluding cameras and lens), Face Recognition in a Crowd Technology Winner, ADS Security Innovation Award, 2021, Galahad facial detection and recognition software, awarded by the UK Home Office at the Security and Policing Show on March 9, 2021.
Works
Search Professor Brian Lovell’s works on UQ eSpace
2018
Journal Article
High-Latitude Neutral Density Structures Investigated by Utilizing Multi-Instrument Satellite Data and NRLMSISE-00 Simulations
Horvath, Ildiko and Lovell, Brian C. (2018). High-Latitude Neutral Density Structures Investigated by Utilizing Multi-Instrument Satellite Data and NRLMSISE-00 Simulations. Journal of Geophysical Research: Space Physics, 123 (2), 1663-1677. doi: 10.1002/2017JA024600
2018
Journal Article
Investigating the Development of Abnormal Subauroral Ion Drift (ASAID) and Abnormal Subauroral Polarization Stream (ASAPS) During the Magnetically Active Times of September 2003
Horvath, Ildiko and Lovell, Brian C. (2018). Investigating the Development of Abnormal Subauroral Ion Drift (ASAID) and Abnormal Subauroral Polarization Stream (ASAPS) During the Magnetically Active Times of September 2003. Journal of Geophysical Research: Space Physics, 123 (2), 1566-1582. doi: 10.1002/2017JA024870
2018
Conference Publication
TV-GAN: generative adversarial network based thermal to visible face recognition
Zhang, Teng, Wiliem, Arnold, Yang, Siqi and Lovell, Brian C. (2018). TV-GAN: generative adversarial network based thermal to visible face recognition. International Conference on Biometrics (ICB), Gold Coast, QLD, Australia, 20 - 23 February 2018. Piscataway, NJ, United States: Institute of Electrical and Electronics Engineers. doi: 10.1109/ICB2018.2018.00035
2018
Conference Publication
An innovative approach to estimate carbon status for improved crop load management in apple
Darbyshire, R., San, W. Y. K., Plozza, T., Lovell, B. C., Flachowsky, H., Wünsche, J. and Stefanelli, D. (2018). An innovative approach to estimate carbon status for improved crop load management in apple. International Symposium on Flowering, Fruit Set and Alternate Bearing, Palermo, Italy, 19-23 June 2017. Leuven, Belgium: International Society for Horticultural Science. doi: 10.17660/ActaHortic.2018.1229.43
2018
Journal Article
Investigating high-latitude energy deposition events occurring during the 17 January 2005 Geomagnetic Storm
Horvath, Ildiko and Lovell, Brian C. (2018). Investigating high-latitude energy deposition events occurring during the 17 January 2005 Geomagnetic Storm. Journal of Geophysical Research: Space Physics, 123 (8), 6760-6775. doi: 10.1029/2018JA025465
2018
Conference Publication
Using LIP to gloss over faces in single-stage face detection networks
Yang, Siqi, Wiliem, Arnold, Chen, Shaokang and Lovell, Brian C. (2018). Using LIP to gloss over faces in single-stage face detection networks. 15th European Conference on Computer Vision, ECCV 2018, Munich, Germany, 8-14 September 2018. Heidelberg, Germany: Springer. doi: 10.1007/978-3-030-01267-0_39
2018
Conference Publication
It takes two to tango: cascading off-the-shelf face detectors
Yang, Siqi, Wiliem, Arnold and Lovell, Brian C. (2018). It takes two to tango: cascading off-the-shelf face detectors. IEEE Conference on Computer Vision and Pattern Recognition Workshops in Biometrics (CVPRW), Salt Lake City, UT, United States, 18 - 22 June 2018. Piscataway, NJ, United States: IEEE Computer Society. doi: 10.1109/CVPRW.2018.00095
2018
Conference Publication
SlideNet: fast and accurate slide quality assessment based on deep neural networks
Zhang, Teng, Carvajal, Johanna, Smith, Daniel F., Zhao, Kun, Wiliem, Arnold, Hobson, Peter, Jennings, Anthony and Lovell, Brian C. (2018). SlideNet: fast and accurate slide quality assessment based on deep neural networks. 24th International Conference on Pattern Recognition, ICPR 2018, Beijing, China, 20 - 24 August 2018. Washington, DC, United States: IEEE Computer Society. doi: 10.1109/ICPR.2018.8546205
2017
Journal Article
Computer face-matching technology using two-dimensional photographs accurately matches the facial gestalt of unrelated individuals with the same syndromic form of intellectual disability
Dudding-Byth, Tracy, Baxter, Anne, Holliday, Elizabeth G., Hackett, Anna, O'Donnell, Sheridan, White, Susan M., Attia, John, Brunner, Han, de Vries, Bert, Koolen, David, Kleefstra, Tjitske, Ratwatte, Seshika, Riveros, Carlos, Brain, Steve and Lovell, Brian C. (2017). Computer face-matching technology using two-dimensional photographs accurately matches the facial gestalt of unrelated individuals with the same syndromic form of intellectual disability. BMC Biotechnology, 17 (1) 90, 1-9. doi: 10.1186/s12896-017-0410-1
2017
Journal Article
Investigating the Development of Localized Neutral Density Increases During the 24 August 2005 Geomagnetic Storm
Horvath, Ildiko and Lovell, Brian C. (2017). Investigating the Development of Localized Neutral Density Increases During the 24 August 2005 Geomagnetic Storm. Journal of Geophysical Research: Space Physics, 122 (11), 11,765-11,783. doi: 10.1002/2017JA024362
2017
Journal Article
Special issue on ubiquitous biometrics
He, Ran, Lovell, Brian C., Chellappa, Rama, Jain, Anil K. and Sun, Zhenan (2017). Special issue on ubiquitous biometrics. Pattern Recognition, 66, 1-3. doi: 10.1016/j.patcog.2017.02.002
2017
Journal Article
Investigating the polar ionosphere during the development of neutral density enhancements on 24-25 September 2000
Horvath, Ildiko and Lovell, Brian C. (2017). Investigating the polar ionosphere during the development of neutral density enhancements on 24-25 September 2000. Journal of Geophysical Research, 122 (4), 4600-4616. doi: 10.1002/2016JA023799
2017
Journal Article
Investigating the development of double-peak subauroral ion drift (DSAID)
Horvath, Ildiko and Lovell, Brian C. (2017). Investigating the development of double-peak subauroral ion drift (DSAID). Journal of Geophysical Research: Space Physics, 122 (4), 4526-4542. doi: 10.1002/2016JA023506
2017
Conference Publication
How do you develop a face detector for the unconstrained environment?
San, Wai Y. K., Chen, Shaokang, Wiliem, Arnold, Di, Binn and Lovell, Brian C. (2017). How do you develop a face detector for the unconstrained environment?. 2016 International Conference on Image and Vision Computing New Zealand, IVCNZ 2016, Palmerston North, New Zealand, 21 - 22 November 2016. Piscataway, NJ, United States: IEEE Computer Society. doi: 10.1109/IVCNZ.2016.7804414
2017
Conference Publication
The GIST of aligning faces
Yang, Siqi, Wiliem, Arnold and Lovell, Brian C. (2017). The GIST of aligning faces. 2016 23rd International Conference on Pattern Recognition (ICPR), Cancun, Mexico, 4-8 December 2016. Piscataway, NJ, United States: Institute of Electrical and Electronics Engineers. doi: 10.1109/ICPR.2016.7900095
2017
Conference Publication
An early experience toward developing computer aided diagnosis for gram-stained smears images
Carvajal, Johanna, Smith, Daniel F., Zhao, Kun, Wiliem, Arnold, Finucane, Paul, Hobson, Peter, Jennings, Anthony, McDougall, Rodney and Lovell, Brian (2017). An early experience toward developing computer aided diagnosis for gram-stained smears images. 30th IEEE/CVF Conference on Computer Vision and Pattern Recognition Workshops (CVPRW), Honolulu, HI, United States, 21-26 July 2017. Piscataway, NJ, United States: IEEE. doi: 10.1109/CVPRW.2017.113
2017
Conference Publication
Landmark manifold: revisiting the Riemannian manifold approach for facial emotion recognition
Zhao, Kun, Yang, Siqi, Wiliem, Arnold and Lovell, Brian C. (2017). Landmark manifold: revisiting the Riemannian manifold approach for facial emotion recognition. 2016 23rd International Conference on Pattern Recognition (ICPR), Cancun, Mexico, 4-8 December 2016. Piscataway, NJ, United States: Institute of Electrical and Electronics Engineers . doi: 10.1109/ICPR.2016.7899782
2017
Conference Publication
To face or not to face: towards reducing false positive of face detection
Yang, Siqi, Wiliem, Arnold and Lovell, Brian C. (2017). To face or not to face: towards reducing false positive of face detection. 2016 International Conference on Image and Vision Computing New Zealand, IVCNZ 2016, Palmerston North, New Zealand, 21 - 22 November 2016. Piscataway, NJ, United States: Institute of Electrical and Electronics Engineers. doi: 10.1109/IVCNZ.2016.7804415
2017
Conference Publication
Towards Miss Universe automatic prediction: the evening gown competition
Carvajal, Johanna, Wiliem, Arnold, Sanderson, Conrad and Lovell, Brian (2017). Towards Miss Universe automatic prediction: the evening gown competition. 23rd International Conference on Pattern Recognition, ICPR 2016, Cancun, Mexico, 4 - 8 December 2016. Piscataway, NJ, United States: Institute of Electrical and Electronics Engineers. doi: 10.1109/ICPR.2016.7899781
2017
Book Chapter
Solving classification problems on human epithelial type 2 cells for anti-nuclear antibodies test: traditional versus contemporary approaches
Wiliem, Arnold and Lovell, Brian C. (2017). Solving classification problems on human epithelial type 2 cells for anti-nuclear antibodies test: traditional versus contemporary approaches. Pattern recognition and Big Data. (pp. 605-632) edited by Amita Pal and Sankar K. Pal. Singapore: World Scientific. doi: 10.1142/9789813144552_0018
Funding
Current funding
Supervision
Availability
- Professor Brian Lovell is:
- Available for supervision
Before you email them, read our advice on how to contact a supervisor.
Available projects
-
Detecting and Classifying Neurofibromas using Deep Learning
Neurofibromatosis type 1 (NF1) is one of the most common single-gene inherited disorders globally, with an incidence of 1/2500 individuals. While several phenotypes are associated with the disorder, the most common manifestation is cutaneous neurofibroma. The majority of adults develop these distressing cutaneous tumours (cNF), which increase in severity with age. Adult patients report cosmetic disfigurement due to cNF as the greatest burden of living with NF1. There is no way to predict tumour severity which can range from <100 to thousands. Youth and families experience reduced quality of life due to concerns about this uncertain future. We don’t yet understand why this condition is so variable or have any effective medical treatments. In the proposed research, we will assemble a consortium of internationally recognised experts in NF1 with access and capacity to recruit and phenotype patients to drive the largest genome-wide association and epigenome-wide association studies of the modifier gene networks driving the cutaneous phenotypic variance in NF1. We will then use individualised pharmacological annotation of these networks to identify precision treatment options to mitigate the most distressing and life quality damaging aspects of this devastating illness.
-
Classifying Gram Stain Images Using Transformers and Deep Learning
Microscopic diagnosis of Gram stain smears is one of the most time and labor intensive tasks in the clinical setting. With the recent development of automated digital pathology scanners, it is now possible to economically obtain high-resolution Gram stain whole slide images for routine diagnosis. This finally opens the doorway to automated identification of bacteria types from digital images in a clinical setting. However, Gram stain whole slide images comprise billions of pixels and suffer from high morphological heterogeneity as well as from many different types of artifacts. Identifying multiple types of tiny bacteria with various densities from an extremely large whole slide image is incredibly challenging. To this end, we propose an end-to-end framework with a novel loss function that tackles the patch aggregation while considering the correlation of different labels in this multi-label scenario. Our framework first effectively integrates the relations among multiple patch features, and then utilizes a class aggregator to generate a robust slide-level feature representation under multi-label setting. Furthermore, we propose a novel loss function integrating two regularization terms: 1) a negative regulator that reduces the confusion between bacteria and negative samples without any bacteria, and 2) an adversarial loss that alleviates the impact of background specification among various tissue samples. We show that the proposed method achieves superior performance compared to several state-of-the-art methods.
-
Incremental Learning for AI
Incremental learning requires a model to continually learn new tasks from streaming data. However, traditional fine-tuning of a well-trained deep neural network on a new task will dramatically degrade performance on the old task — a problem known as catastrophic forgetting. We address this issue in the context of anchor-free object detection, which is a new trend in computer vision as it is simple, fast, and flexible. Simply adapting current incremental learning strategies fails on these anchor-free detectors due to lack of consideration of their specific model structures. To deal with the challenges of incremental learning on anchor-free object detectors, we propose a novel incremental learning paradigm called Selective and Inter-related Distillation (SID). In addition, a novel evaluation metric is proposed to better assess the performance of detectors under incremental learning conditions. By selective distilling at the proper locations and further transferring additional instance relation knowledge, our method demonstrates significant advantages on the benchmark datasets PASCAL VOC and COCO.
-
Text to Face Synthesis using Stable Diffusion for Biometrics Research
Text-to-Face (TTF) synthesis is a challenging task with great potential for diverse computer vision applications. Compared to Text-to-Image (TTI) synthesis tasks, the textual description of faces can be much more complicated and detailed due to the variety of facial attributes and the parsing of high dimensional abstract natural language. We propose a text-to-face model that should not only produce images in high resolution (10241024) and text-to-image consistency, but also output multiple faces to cover a wide range of unspecified facial features in a natural way. By fine-tuning the multi-label classifier and image encoder, our model obtains the vectors and image embeddings which are used to manipulate the noise vector sampled from the normal distribution. Afterwards, the manipulated noise vector is fed into a pre-trained high-resolution image generator to produce a set of faces with desired facial attributes. We refer to our model as TTF-HD. Experimental results show that TTF-HD generates high-quality faces and achieves state-of-the-art performance.
Supervision history
Current supervision
-
Doctor Philosophy
Advanced Strategies to Alleviate Challenges of Data Scarcity in Deep Learning for Medical Image Analysis
Principal Advisor
Other advisors: Associate Professor Marcus Gallagher
-
Doctor Philosophy
Out-of-Distribution Generalisation and Detection in Feature Embedding Space
Principal Advisor
Other advisors: Associate Professor Mahsa Baktashmotlagh
-
Doctor Philosophy
Generating data-driven continuous optimization problems for benchmarking
Associate Advisor
Other advisors: Associate Professor Marcus Gallagher
-
Doctor Philosophy
Modelling cloud movement to generate short term solar irradiance predictions and subsequent expected PV power production
Associate Advisor
Other advisors: Professor Eve McDonald-Madden, Dr Hui Ma
-
Doctor Philosophy
Enhancing Building Fire Safety by Utilising Machine Learning Techniques
Associate Advisor
Other advisors: Dr Xin Yu
Completed supervision
-
2024
Doctor Philosophy
Data Augmentation through Image Synthesis and Editing in Multi-domains via Disentangled Latent Space
Principal Advisor
-
2024
Doctor Philosophy
Modelling Efficient and Robust Solutions for Microbiology Image Analysis Using Deep Learning
Principal Advisor
-
2023
Doctor Philosophy
Whole Slide Image Processing in the Immunofluorescence Domain
Principal Advisor
-
2023
Doctor Philosophy
Modeling Data Scarcity Solutions with Deep Learning for Histopathology Image Analysis
Principal Advisor
-
-
2020
Doctor Philosophy
On the Robustness of Object and Face Detection: False Positives, Attacks and Adaptability
Principal Advisor
-
2020
Master Philosophy
Accurate Dense Depth From Light Field Technology For Object Segmentation And 3D Computer Vision
Principal Advisor
-
2017
Doctor Philosophy
Exploring The Frontier of Smart Video Surveillance: Novel Domains and Fine-Grain Event Understanding
Principal Advisor
-
-
2016
Doctor Philosophy
Fast and Accurate Image and Video Analysis on Riemannian Manifolds
Principal Advisor
-
2016
Doctor Philosophy
Techniques for Binocular Markerless Visual Tracking of 3D Articulated Bodies
Principal Advisor
-
2016
Doctor Philosophy
Action Analysis and Video Summarisation to Efficiently Manage and Interpret Video Data
Principal Advisor
-
2016
Doctor Philosophy
Countering Digital Replay Attacks for Face Verification on Consumer Smart Devices using Structured Illumination
Principal Advisor
Other advisors: Emeritus Professor Paul Bailes
-
-
-
-
2014
Doctor Philosophy
Towards Robust Representation and Classification of Low-resolution Image and Video Data
Principal Advisor
-
2014
Doctor Philosophy
Video Analysis Based on Learning on Special Manifolds for Visual Recognition
Principal Advisor
-
2013
Doctor Philosophy
Improving Representation and Classification of Image and Video Data for Surveillance Applications
Principal Advisor
-
2012
Doctor Philosophy
Contextual Spatial Analysis and Processing for Visual Surveillance Applications
Principal Advisor
-
2012
Doctor Philosophy
Towards Robust Identity Inference Under Surveillance Environments: From Still Images to Video Sequences
Principal Advisor
-
2011
Doctor Philosophy
Real-Time Face Detection on High Resolution Smart Camera System
Principal Advisor
-
-
2008
Master Philosophy
Robust Person and Vehicle Tracking for Intelligent Visual Surveillance
Principal Advisor
-
2008
Doctor Philosophy
Efficient and invariant regularisation with application to computer graphics
Principal Advisor
-
2007
Doctor Philosophy
ROBUST FACE RECOGNITION FOR VIDEO SURVEILLANCE USING A SINGLE GALLERY IMAGE
Principal Advisor
-
2006
Doctor Philosophy
EFFICIENT METHODS FOR 3D RECONSTRUCTION FROM MULTIPLE IMAGES
Principal Advisor
-
2006
Doctor Philosophy
THE MULTIPLE-VIEW GEOMETRY OF IMPLICIT CURVES AND SURFACES
Principal Advisor
-
2005
Doctor Philosophy
Training Hidden Markov Models for Spatio-Temporal Pattern Recognition
Principal Advisor
-
2005
Doctor Philosophy
ROBUST DISCRIMINATIVE PRINCIPAL COMPONENT ANALYSIS FOR FACE RECOGNITION
Principal Advisor
-
2005
Doctor Philosophy
GLOBALLY MINIMAL CONTOURS AND SURFACES FOR IMAGE SEGMENTATION
Principal Advisor
-
2004
Master Engineering Sc
OPTICAL FLOW FROM COLOUR IMAGE SEQUENCES
Principal Advisor
-
2004
Doctor Philosophy
HAND GESTURE RECOGNITION BY HIDDEN MARKOV MODELS
Principal Advisor
-
2003
Master Philosophy
Three-dimensional reconstruction of highly complex and mobile surrounds from stereo images and image sequences
Principal Advisor
-
-
2008
Doctor Philosophy
A SEMANTIC FRAMEWORK FOR THE MANAGEMENT, ANALYSIS AND ASSIMILATION OF MIXED-MEDIA SCIENTIFIC DATA
Associate Advisor
-
2006
Doctor Philosophy
AUTOMATIC BANK CHECK PROCESSSING AND AUTHENTICATION USING SIGNATURE VERIFICATION
Associate Advisor
-
2004
Master Philosophy
SECURE LOCATION SERVICES - VULNERABILITY ANALYSIS AND PROVISION OF SECURITY IN LOCATION SYSTEMS
Associate Advisor
-
2004
Master Philosophy
COLLABORATIVE VIDEO INDEXING, ANNOTATION AND DISCUSSION OVER HIGH-BANDWIDTH NETWORKS
Associate Advisor
Media
Enquiries
Contact Professor Brian Lovell directly for media enquiries about:
- Artificial Intelligence
- Biometrics
- Border control
- Computer modelling
- Computer vision
- Deep Learning
- Face Recognition
- Face-recognition technology
- Identification technology
- Image processing
- Information technology
- National security surveillance
- Networks - neural
- Neural networks - artificial
- Pattern Recognition
- Pattern recognition - digital imaging
- Signal Processing
- Wearable Technologies
Need help?
For help with finding experts, story ideas and media enquiries, contact our Media team: