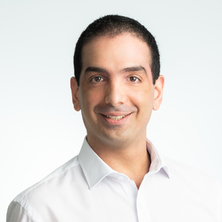
Overview
Background
Peyman Moghadam is an Adjunct Associate Professor at the University of Queensland (UQ). He is a Principal Research Scientist at CSIRO Data61 as well as Professor (Adjunct) at the Queensland University of Technology (QUT). He leads the Embodied AI Research Cluster at CSIRO Data61, working at the intersection of Robotics and Machine learning. He is also the Spatiotemporal AI portfolio Leader at the CSIRO's Machine Learning and Artificial Intelligence (MLAI) Future Science Platform and oversees research and development of MLAI methods for scientific discovery in spatiotemporal data streams. In 2022, he served as a Visiting Professor at ETH Zürich. In 2019, he held a Visiting Scientist appointment at the University of Bonn. Peyman has led several large-scale multidisciplinary projects and won numerous awards, including CSIRO's Julius Career Award, National, and Queensland state iAward for Research and Development, CSIRO’s Collaboration Medal and the Lord Mayor’s Budding Entrepreneurs Award. His current research interests include self-supervised learning for robotics, embodied AI, 3D multi-modal perception (3D++), robotics, and computer vision.
Availability
- Dr Peyman Moghadam is:
- Available for supervision
Fields of research
Research interests
-
Embodied Intelligence; Self-Supervised Learning; spatiotemporal learning
-
Robotics, Computer Vision, Machine Learning, Deep Learning
-
Beyond visible Spectrum Perception (Hyperspectral, Thermal)
-
3D LiDAR SLAM; 3D Scene understanding; 3D Segmentation
Works
Search Professor Peyman Moghadam’s works on UQ eSpace
2024
Journal Article
WildScenes: A benchmark for 2D and 3D semantic segmentation in large-scale natural environments
Vidanapathirana, Kavisha, Knights, Joshua, Hausler, Stephen, Cox, Mark, Ramezani, Milad, Jooste, Jason, Griffiths, Ethan, Mohamed, Shaheer, Sridharan, Sridha, Fookes, Clinton and Moghadam, Peyman (2024). WildScenes: A benchmark for 2D and 3D semantic segmentation in large-scale natural environments. The International Journal of Robotics Research. doi: 10.1177/02783649241278369
2024
Conference Publication
Reg-NF: Efficient Registration of Implicit Surfaces within Neural Fields
Hausler, Stephen, Hall, David, Mahendren, Sutharsan and Moghadam, Peyman (2024). Reg-NF: Efficient Registration of Implicit Surfaces within Neural Fields. IEEE. doi: 10.1109/icra57147.2024.10610982
2024
Journal Article
Graph neural networks-enhanced relation prediction for ecotoxicology (GRAPE)
Anand, Gaurangi, Koniusz, Piotr, Kumar, Anupama, Golding, Lisa A., Morgan, Matthew J. and Moghadam, Peyman (2024). Graph neural networks-enhanced relation prediction for ecotoxicology (GRAPE). Journal of Hazardous Materials, 472 ARTN 134456, 134456. doi: 10.1016/j.jhazmat.2024.134456
2023
Journal Article
FactoFormer: factorized hyperspectral transformers with self-supervised pre-training
Mohamed, Shaheer, Haghighat, Maryam, Fernando, Tharindu, Sridharan, Sridha, Fookes, Clinton and Moghadam, Peyman (2023). FactoFormer: factorized hyperspectral transformers with self-supervised pre-training. IEEE Transactions on Geoscience and Remote Sensing, 62 5501614, 1-1. doi: 10.1109/tgrs.2023.3343392
2023
Journal Article
Pose-graph attentional graph neural network for lidar place recognition
Ramezani, Milad, Wang, Liang, Knights, Joshua, Li, Zhibin, Pounds, Pauline and Moghadam, Peyman (2023). Pose-graph attentional graph neural network for lidar place recognition. IEEE Robotics and Automation Letters, 9 (2), 1182-1189. doi: 10.1109/lra.2023.3341766
2023
Journal Article
GeoAdapt: self-supervised test-time adaptation in LiDAR place recognition using geometric priors
Knights, Joshua, Hausler, Stephen, Sridharan, Sridha, Fookes, Clinton and Moghadam, Peyman (2023). GeoAdapt: self-supervised test-time adaptation in LiDAR place recognition using geometric priors. IEEE Robotics and Automation Letters, PP (99) 3337698, 915-922. doi: 10.1109/lra.2023.3337698
2023
Journal Article
CL3: generalization of contrastive loss for lifelong learning
Roy, Kaushik, Simon, Christian, Moghadam, Peyman and Harandi, Mehrtash (2023). CL3: generalization of contrastive loss for lifelong learning. Journal of Imaging, 9 (12) 259, 1-16. doi: 10.3390/jimaging9120259
2023
Journal Article
Exploiting field dependencies for learning on categorical data
Li, Zhibin, Koniusz, Piotr, Zhang, Lu, Pagendam, Daniel Edward and Moghadam, Peyman (2023). Exploiting field dependencies for learning on categorical data. IEEE Transactions on Pattern Analysis and Machine Intelligence, 45 (11), 13509-13522. doi: 10.1109/tpami.2023.3298028
2023
Conference Publication
Uncertainty-aware lidar place recognition in novel environments
Mason, Keita, Knights, Joshua, Ramezani, Milad, Moghadam, Peyman and Miller, Dimity (2023). Uncertainty-aware lidar place recognition in novel environments. 2023 IEEE/RSJ International Conference on Intelligent Robots and Systems (IROS), Detroit, MI United States, 1 - 5 October 2023. Piscataway, NJ United States: Institute of Electrical and Electronics Engineers. doi: 10.1109/iros55552.2023.10341383
2023
Journal Article
Subspace distillation for continual learning
Roy, Kaushik, Simon, Christian, Moghadam, Peyman and Harandi, Mehrtash (2023). Subspace distillation for continual learning. Neural Networks, 167, 65-79. doi: 10.1016/j.neunet.2023.07.047
2023
Conference Publication
Deep robust multi-robot re-localisation in natural environments
Ramezani, Milad, Griffiths, Ethan, Haghighat, Maryam, Pitt, Alex and Moghadam, Peyman (2023). Deep robust multi-robot re-localisation in natural environments. 2023 IEEE/RSJ International Conference on Intelligent Robots and Systems (IROS), Detroit, MI United States, 1 - 5 October 2023. Piscataway, NJ United States: Institute of Electrical and Electronics Engineers. doi: 10.1109/iros55552.2023.10341798
2023
Conference Publication
Measuring situational awareness latency in human-robot teaming experiments
Senaratne, Hashini, Pitt, Alex, Talbot, Fletcher, Moghadam, Peyman, Sikka, Pavan, Howard, David, Williams, Jason, Kulić, Dana and Paris, Cécile (2023). Measuring situational awareness latency in human-robot teaming experiments. 32nd IEEE International Conference on Robot and Human Interactive Communication (RO-MAN), Busan, South Korea, 28-31 August 2023. Piscataway, NJ, United States: Institute of Electrical and Electronics Engineers. doi: 10.1109/ro-man57019.2023.10309377
2023
Conference Publication
Learning partial correlation based deep visual representation for image classification
Rahman, Saimunur, Koniusz, Piotr, Wang, Lei, Zhou, Luping, Moghadam, Peyman and Sun, Changming (2023). Learning partial correlation based deep visual representation for image classification. IEEE/CVF Conference on Computer Vision and Pattern Recognition (CVPR), Vancouver, BC Canada, 17-24 June 2023. Washington, DC United States: IEEE Computer Society. doi: 10.1109/cvpr52729.2023.00603
2023
Conference Publication
Wild-places: a large-scale dataset for lidar place recognition in unstructured natural environments
Knights, Joshua, Vidanapathirana, Kavisha, Ramezani, Milad, Sridharan, Sridha, Fookes, Clinton and Moghadam, Peyman (2023). Wild-places: a large-scale dataset for lidar place recognition in unstructured natural environments. 2023 IEEE International Conference on Robotics and Automation (ICRA), London, United Kingdom, 29 May - 2 June 2023. Washington, DC, United States: IEEE Computer Society. doi: 10.1109/icra48891.2023.10160432
2023
Journal Article
Spectral geometric verification: re-ranking point cloud retrieval for metric localization
Vidanapathirana, Kavisha, Moghadam, Peyman, Sridharan, Sridha and Fookes, Clinton (2023). Spectral geometric verification: re-ranking point cloud retrieval for metric localization. IEEE Robotics and Automation Letters, 8 (5), 2494-2501. doi: 10.1109/lra.2023.3255560
2023
Journal Article
Plant species richness prediction from DESIS hyperspectral data: a comparison study on feature extraction procedures and regression models
Guo, Yiqing, Mokany, Karel, Ong, Cindy, Moghadam, Peyman, Ferrier, Simon and Levick, Shaun R. (2023). Plant species richness prediction from DESIS hyperspectral data: a comparison study on feature extraction procedures and regression models. ISPRS Journal of Photogrammetry and Remote Sensing, 196, 120-133. doi: 10.1016/j.isprsjprs.2022.12.028
2023
Conference Publication
L3DMC: Lifelong learning using distillation via mixed-curvature space
Roy, Kaushik, Moghadam, Peyman and Harandi, Mehrtash (2023). L3DMC: Lifelong learning using distillation via mixed-curvature space. MICCAI 2023: 26th International Conference, Vancouver, BC, Canada, 8–12 October 2023. Cham, Switzerland: Springer Nature Switzerland. doi: 10.1007/978-3-031-43895-0_12
2023
Conference Publication
Flashback for continual learning
Mahmoodi, Leila, Harandi, Mehrtash and Moghadam, Peyman (2023). Flashback for continual learning. IEEE/CVF International Conference on Computer Vision (ICCV), Paris, France, 2-6 October 2023. Piscataway, NJ, United States: IEEE Computer Society. doi: 10.1109/ICCVW60793.2023.00368
2022
Conference Publication
InCloud: incremental learning for point cloud place recognition
Knights, Joshua, Moghadam, Peyman, Ramezani, Milad, Sridharan, Sridha and Fookes, Clinton (2022). InCloud: incremental learning for point cloud place recognition. 2022 IEEE/RSJ International Conference on Intelligent Robots and Systems (IROS), Kyoto, Japan, 23-27 October 2022. Piscataway, NJ, United States: Institute of Electrical and Electronics Engineers. doi: 10.1109/iros47612.2022.9981252
2022
Conference Publication
A real-time edge-AI system for reef surveys
Li, Yang, Liu, Jiajun, Kusy, Brano, Marchant, Ross, Do, Brendan, Merz, Torsten, Crosswell, Joey, Steven, Andy, Tychsen-Smith, Lachlan, Ahmedt-Aristizabal, David, Oorloff, Jeremy, Moghadam, Peyman, Babcock, Russ, Malpani, Megha and Oerlemans, Ard (2022). A real-time edge-AI system for reef surveys. ACM MobiCom '22: The 28th Annual International Conference on Mobile Computing and Networking, Sydney, NSW Australia, 17-21 October 2022. New York, NY, USA: ACM. doi: 10.1145/3495243.3558278
Supervision
Availability
- Dr Peyman Moghadam is:
- Available for supervision
Before you email them, read our advice on how to contact a supervisor.
Available projects
-
Self-Supervised Learning for 3D Multimodal Perception
Potential impact of deep learning is limited due to the lack of large, annotated, and high-quality datasets in domains of interest. Annotating such datasets is laborious, costly and time-consuming. This project proposes to develop self-supervised learning systems to extract and use the relevant context given by strong prior spatio-temporal models (e.g. dense 3D reconstructions) as supervisory signals in training. This new concept will investigate model structures that encodes spatio-temporal data, and show rapid adaptation of models to new domains (few-shot learning) using trained embeddings layers (self-supervised, or prior data).
-
3D Scene Understanding
Simultaneous Localization and Mapping (SLAM) is a key enabling component of driverless vehicles, robotics and augmented reality. The SLAM goal is to estimate pose of the vehicle and simultaneously generate dense 3D scene reconstruction. At CSIRO we have developed and deployed state-of-the-art 3D LiDAR-based SLAM systems for the past decade. There is a new direction of research at the intersection of deep learning and geometry-based 3D SLAM. The research in this PhD programme will develop algorithms for geometry-based Deep Learning SLAM in a dynamic and unstructured environment. The PhD programme will involve the development of self or semi-supervised learning methods to address the significant weakness of most current deep networks.
-
Hyperspectral Deep Learning
Hyperspectral cameras are currently undergoing a change from bulky and expensive equipment towards mobile and portable devices. A hyperspectral camera comprises of hundreds of bands with shortwave dependencies. Compared to conventional colour cameras (RGB bands), one could use these shortwave dependencies to design and develop a deep network for object classification, semantic segmentation and scene understanding. Both spectral and spatial relationship needs to be modelled by the deep networks simultaneously. The research in this PhD programme will develop algorithms for hyperspectral deep learning. The PhD programme will involve the development of learning with self-supervision algorithms to address the significant weakness of most current deep networks.
Supervision history
Current supervision
-
Doctor Philosophy
Generalizing Implicit Representations for Robotics Manipulation of Articulated Objects
Associate Advisor
Other advisors: Dr Mahsa Baktashmotlagh
Media
Enquiries
For media enquiries about Dr Peyman Moghadam's areas of expertise, story ideas and help finding experts, contact our Media team: