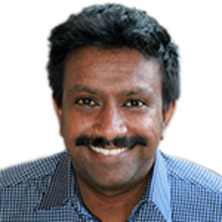
Overview
Background
Shakes an imaging expert that leads a strong deep learning, artificial intelligence (AI) focused research team interested in medical image analysis and signal/image processing applied to many areas of science and medicine. He received his Ph.D in Theoretical Physics from Monash University, Melbourne and has been involved in applying machine learning in medical imaging for over a decade.
Shakes’ past work has involved developing shape model-based algorithms for knee, hip and shoulder joint segmentation that is being developed and deployed as a product on the Siemens syngo.via platform. More recent work involves deep learning based algorithms for semantic segmentation and manifold learning of imaging data. Broadly, he is interested in understanding and developing the mathematical basis of imaging, image analysis algorithms and physical systems. He has developed algorithms that utilise exotic mathematical structures such as fractals, turbulence, group theoretic concepts and number theory in the image processing approaches that he has developed.
He is currently a Senior Lecturer and leads a team of 20+ researchers working image analysis and AI research across healthcare and medicine. He currently teaches the computer science courses Theory of Computation and Pattern Recognition and Analysis.
Availability
- Dr Shakes Chandra is:
- Available for supervision
Fields of research
Qualifications
- Doctor of Philosophy, Monash University
Research interests
-
Magnetic Resonance Imaging
Making MRI faster and more affordable through better image reconstruction, processing and analysis.
-
Image Processing
Image reconstruction, segmentation and registration.
-
Deep learning
Dimensionality reduction, machine learning and Artificial Intelligence
-
Fractals and Chaos
Applying fractals and chaos to image processing and computer science.
-
Number Theory
Applying number theory to image processing and computer science.
-
Medical Image Analysis
Medical image segmentation and shape analysis
Works
Search Professor Shakes Chandra’s works on UQ eSpace
2023
Conference Publication
Medical shape pattern analysis with MeshCNN
Leung, Sze-Nung, Dowling, Jason A., Fripp, Jurgen, Shen, Kai-Kai and Chandra, Shekhar S. (2023). Medical shape pattern analysis with MeshCNN. 20th IEEE International Symposium on Biomedical Imaging (ISBI), Cartagena, Colombia, 18-21 April 2023. Piscataway, NJ United States: Institute of Electrical and Electronics Engineers. doi: 10.1109/isbi53787.2023.10230427
2023
Conference Publication
TriFormer: A multi-modal transformer framework for mild cognitive impairment conversion prediction
Liu, Linfeng, Lyu, Junyan, Liu, Siyu, Tang, Xiaoying, Chandra, Shekhar S. and Nasrallah, Fatima A. (2023). TriFormer: A multi-modal transformer framework for mild cognitive impairment conversion prediction. 20th IEEE International Symposium on Biomedical Imaging (ISBI), Cartagena, Colombia, 18-21 April 2023. Piscataway, NJ United States: Institute of Electrical and Electronics Engineers. doi: 10.1109/isbi53787.2023.10230709
2023
Journal Article
An unsupervised deep learning-based image translation method for retrospective motion correction of high resolution kidney MRI
Moinian, Shahrzad, Kurniawan, Nyoman D., Chandra, Shekhar S., Vegh, Viktor and Reutens, David C. (2023). An unsupervised deep learning-based image translation method for retrospective motion correction of high resolution kidney MRI. Intelligence-Based Medicine, 8 100108, 1-15. doi: 10.1016/j.ibmed.2023.100108
2023
Journal Article
Cascaded multi-modal mixing transformers for Alzheimer’s disease classification with incomplete data
Liu, Linfeng, Liu, Siyu, Zhang, Lu, To, Xuan Vinh, Nasrallah, Fatima and Chandra, Shekhar S. (2023). Cascaded multi-modal mixing transformers for Alzheimer’s disease classification with incomplete data. NeuroImage, 277 120267, 120267. doi: 10.1016/j.neuroimage.2023.120267
2023
Conference Publication
Towards trustable skin cancer diagnosis via rewriting model's decision
Yan, Siyuan, Yu, Zhen, Zhang, Xuelin, Mahapatra, Dwarikanath, Chandra, Shekhar S., Janda, Monika, Soyer, Peter and Ge, Zongyuan (2023). Towards trustable skin cancer diagnosis via rewriting model's decision. 2023 IEEE/CVF Conference on Computer Vision and Pattern Recognition (CVPR), Vancouver, BC, Canada, 17-24 June 2023. Piscataway, NJ, United States: IEEE. doi: 10.1109/cvpr52729.2023.01113
2023
Journal Article
PREdiction and Diagnosis using Imaging and Clinical biomarkers Trial in Traumatic Brain Injury (PREDICT-TBI) study protocol: an observational, prospective, multicentre cohort study for the prediction of outcome in moderate-to-severe TBI
Nasrallah, Fatima, Bellapart, Judith, Walsham, James, Jacobson, Esther, To, Xuan Vinh, Manzanero, Silvia, Brown, Nathan, Meyer, Jason, Stuart, Janine, Evans, Tracey, Chandra, Shekhar S., Ross, Jason, Campbell, Lewis, Senthuran, Siva, Newcombe, Virginia, McCullough, James, Fleming, Jennifer, Pollard, Clifford and Reade, Michael (2023). PREdiction and Diagnosis using Imaging and Clinical biomarkers Trial in Traumatic Brain Injury (PREDICT-TBI) study protocol: an observational, prospective, multicentre cohort study for the prediction of outcome in moderate-to-severe TBI. BMJ Open, 13 (4) e067740, 1-9. doi: 10.1136/bmjopen-2022-067740
2023
Conference Publication
Semantic segmentation of 3D medical images through a kaleidoscope: data from the Osteoarthritis Initiative
Woo, Boyeong, Bran Lorenzana, Marlon, Engstrom, Craig, Baresic, William, Fripp, Jurgen, Crozier, Stuart and Chandra, Shekhar S. (2023). Semantic segmentation of 3D medical images through a kaleidoscope: data from the Osteoarthritis Initiative. Medical Imaging with Deep Learning, Nashville, TN, United States, 10-12 July 2023. Cambridge, MA, United States: ML Research Press.
2023
Conference Publication
Semantic segmentation of 3D medical images through a kaleidoscope: data from the Osteoarthritis Initiative
Woo, Boyeong, Bran Lorenzanal, Marlon, Engstrom, Craig, Baresic, William, Fripp, Jurgen, Crozier, Stuart and Chandra, Shekhar S. (2023). Semantic segmentation of 3D medical images through a kaleidoscope: data from the Osteoarthritis Initiative. 6th International Conference on Medical Imaging with Deep Learning (MIDL), Nashville, TN, United States, 10-12 July 2023. San Diego, CA, United States: JMLR-Journal of Machine Learning Research.
2023
Conference Publication
Style-based manifold for weakly-supervised disease characteristic discovery
Liu, Siyu, Liu, Linfeng, Engstrom, Craig, To, Xuan Vinh, Ge, Zongyuan, Crozier, Stuart, Nasrallah, Fatima and Chandra, Shekhar S. (2023). Style-based manifold for weakly-supervised disease characteristic discovery. MICCAI 2023: 26th International Conference, Vancouver, BC, Canada, 8-12 October 2023. Heidelberg, Germany: Springer. doi: 10.1007/978-3-031-43904-9_36
2022
Other Outputs
Osteoarthritis Initiative (OAI) - UQ
Woo, Boyeong , Chandra, Shekhar S. , Engstrom, Craig and Crozier, Stuart (2022). Osteoarthritis Initiative (OAI) - UQ. The University of Queensland. (Dataset) doi: 10.48610/d8e13fb
2022
Conference Publication
Transformer compressed sensing via global image tokens
Bran Lorenzana, Marlon, Engstrom, Craig and Chandra, Shekhar S. (2022). Transformer compressed sensing via global image tokens. 2022 IEEE International Conference on Image Processing (ICIP), Bordeaux, France, 16-19 October 2022. Piscataway, NJ, United States: IEEE. doi: 10.1109/icip46576.2022.9897630
2022
Conference Publication
Skin lesion recognition with class-hierarchy regularized hyperbolic embeddings
Yu, Zhen, Nguyen, Toan, Gal, Yaniv, Ju, Lie, Chandra, Shekhar S., Zhang, Lei, Bonnington, Paul, Mar, Victoria, Wang, Zhiyong and Ge, Zongyuan (2022). Skin lesion recognition with class-hierarchy regularized hyperbolic embeddings. 25th International Conference on Medical Image Computing and Computer Assisted Intervention (MICCAI), Singapore, Singapore, 18-22 September 2022. Heidelberg, Germany: Springer. doi: 10.1007/978-3-031-16437-8_57
2022
Journal Article
CAN3D: Fast 3D medical image segmentation via compact context aggregation
Dai, Wei, Woo, Boyeong, Liu, Siyu, Marques, Matthew, Engstrom, Craig, Greer, Peter B., Crozier, Stuart, Dowling, Jason A. and Chandra, Shekhar S. (2022). CAN3D: Fast 3D medical image segmentation via compact context aggregation. Medical Image Analysis, 82 102562, 1-17. doi: 10.1016/j.media.2022.102562
2022
Journal Article
Deep neural networks predict the need for CT in pediatric mild traumatic brain injury: a corroboration of the PECARN rule
Ellethy, Hanem, Chandra, Shekhar S. and Nasrallah, Fatima A. (2022). Deep neural networks predict the need for CT in pediatric mild traumatic brain injury: a corroboration of the PECARN rule. Journal of the American College of Radiology, 19 (6), 769-778. doi: 10.1016/j.jacr.2022.02.024
2022
Journal Article
Automated 3D analysis of clinical magnetic resonance images demonstrates significant reductions in cam morphology following arthroscopic intervention in contrast to physiotherapy
Bugeja, Jessica M., Xia, Ying, Chandra, Shekhar S., Murphy, Nicholas J., Eyles, Jillian, Spiers, Libby, Crozier, Stuart, Hunter, David J., Fripp, Jurgen and Engstrom, Craig (2022). Automated 3D analysis of clinical magnetic resonance images demonstrates significant reductions in cam morphology following arthroscopic intervention in contrast to physiotherapy. Arthroscopy, Sports Medicine, and Rehabilitation, 4 (4), e1353-e1362. doi: 10.1016/j.asmr.2022.04.020
2022
Conference Publication
Anomaly-aware 3D segmentation of knee magnetic resonance images
Woo, Boyeong, Engstrom, Craig, Fripp, Jurgen, Crozier, Stuart and Chandra, Shekhar S. (2022). Anomaly-aware 3D segmentation of knee magnetic resonance images. 5th International Conference on Medical Imaging with Deep Learning, Zurich, Switzerland, 6-8 July 2022. Cambridge, MA United States: ML Research Press.
2022
Journal Article
Automated volumetric and statistical shape assessment of cam-type morphology of the femoral head-neck region from clinical 3D magnetic resonance images
Bugeja, Jessica M., Xia, Ying, Chandra, Shekhar S., Murphy, Nicholas J., Eyles, Jillian, Spiers, Libby, Crozier, Stuart, Hunter, David J., Fripp, Jurgen and Engstrom, Craig (2022). Automated volumetric and statistical shape assessment of cam-type morphology of the femoral head-neck region from clinical 3D magnetic resonance images. Quantitative Imaging in Medicine and Surgery, 12 (10), 4941. doi: 10.21037/qims-22-332
2022
Conference Publication
Undersampled MRI reconstruction with side information-guided normalisation
Liu, Xinwen, Wang, Jing, Peng, Cheng, Chandra, Shekhar S., Liu, Feng and Zhou, S. Kevin (2022). Undersampled MRI reconstruction with side information-guided normalisation. Medical Image Computing and Computer Assisted Intervention – MICCAI, Singapore, Singapore, 18-22 September 2022. Cham, Switzerland: Springer Nature Switzerland. doi: 10.1007/978-3-031-16446-0_31
2022
Conference Publication
FDGATII: Fast Dynamic Graph Attention with Initial Residual and Identity
Kulatilleke, Gayan K., Portmann, Marius, Ko, Ryan and Chandra, Shekhar S. (2022). FDGATII: Fast Dynamic Graph Attention with Initial Residual and Identity. 35th Australasian Joint Conference on Artificial Intelligence: AI 2022, Perth, WA Australia, 5–8 December 2022. Cham, Switzerland: Springer. doi: 10.1007/978-3-031-22695-3_6
2021
Conference Publication
Slim-YOLO: a simplified object detection model for the detection of pigmented iris freckles as a potential biomarker for cutaneous melanoma
Naranpanawa, D. Nathasha U., Gu, Yanyang, Chandra, Shekhar S., Betz-Stablein, Brigid, Sturm, Richard A., Soyer, H. Peter and Eriksson, Anders P. (2021). Slim-YOLO: a simplified object detection model for the detection of pigmented iris freckles as a potential biomarker for cutaneous melanoma. Digital Image Computing: Techniques and Applications (DICTA), Gold Coast, Australia, 29 November - 1 December 2021. Piscataway, NJ, United States: Institute of Electrical and Electronics Engineers. doi: 10.1109/dicta52665.2021.9647150
Funding
Current funding
Past funding
Supervision
Availability
- Dr Shakes Chandra is:
- Available for supervision
Before you email them, read our advice on how to contact a supervisor.
Available projects
-
Machine learning applied to 3D magnetic resonance images
Magnetic resonance (MR) imaging has become an important non-invasive radiological modality for various clinical applications, such as cartilage assessment for Osteoarthritis and treatment planning for prostate cancer. MR images in 3D, while providing a wealth of anatomical information, including bones and soft tissue, are difficult to analyse due to the presence of a large number of complex structures. Thus, extracting meaningful clinical information without human interaction is a challenging task. Developing such automatic methods are important in order to reduce human errors and the time taken by clinicians in completing mundane tasks, such as marking or delineating 3D images by hand, from hours to just a few minutes by utilising computers.
In this project, the student will develop novel algorithms to solve segmentation and detection problems for MR imaging that could possibly be deployed to MRI scanners and may eventually used for diagnostic purposes. The project will involve applying computer vision and machine learning techniques (including deep learning) to MR image processing and analysis.
Supervision history
Current supervision
-
Doctor Philosophy
Medical Shape Analysis of 3D MRI Segmentation with Deep Learning
Principal Advisor
Other advisors: Emeritus Professor Stuart Crozier
-
Doctor Philosophy
Automated Detection and Classification of Suspicious Naevi in Dermoscopy Images Through Artificial Intelligence
Principal Advisor
Other advisors: Professor Peter Soyer, Professor Monika Janda
-
Doctor Philosophy
Novel deep learning approaches to understanding human diseases
Principal Advisor
Other advisors: Associate Professor Craig Engstrom, Emeritus Professor Stuart Crozier
-
Doctor Philosophy
Magnetic Resonance Image Processing with Artificial Intelligence
Principal Advisor
Other advisors: Associate Professor Craig Engstrom, Dr Hongfu Sun
-
Doctor Philosophy
Manifold Learning for Magnetic Resonance Imaging
Principal Advisor
Other advisors: Associate Professor Craig Engstrom, Emeritus Professor Stuart Crozier
-
Doctor Philosophy
Foundational models for visual recognition
Principal Advisor
Other advisors: Associate Professor Craig Engstrom, Emeritus Professor Stuart Crozier
-
Doctor Philosophy
Deep Learning Applied to Medical Image Analysis
Principal Advisor
Other advisors: Associate Professor Craig Engstrom, Emeritus Professor Stuart Crozier
-
Doctor Philosophy
Deployable and Explainable Deep Learning Architectures: Navigating Clinical Challenges in Medical Image Segmentation
Principal Advisor
Other advisors: Associate Professor Craig Engstrom, Emeritus Professor Stuart Crozier
-
Doctor Philosophy
Deep Learning Strategies for Enhanced mTBI Diagnosis Using Clinical and CT Data
Principal Advisor
-
Doctor Philosophy
Towards Analysis of Contextual Melanoma Indicators and Identification of Total-Body Ugly Duckling Lesions with Deep Neural Networks
Principal Advisor
Other advisors: Associate Professor Mahsa Baktashmotlagh
-
Doctor Philosophy
Manifold Learning for Magnetic Resonance Imaging
Principal Advisor
Other advisors: Associate Professor Craig Engstrom, Emeritus Professor Stuart Crozier
-
Doctor Philosophy
Improving Feature Extraction and Feature Interpretability for Deep Learning in Medical Image Analysis
Principal Advisor
Other advisors: Associate Professor Craig Engstrom, Emeritus Professor Stuart Crozier
-
Doctor Philosophy
Artifical intelligence approaches for diagnosing and phenotyping sleep disorders
Associate Advisor
Other advisors: Professor Juha Toyras, Associate Professor Philip Terrill
-
Doctor Philosophy
Advanced Deep Learning Approaches for Improving Diagnosis and Prognosis in Brain Disease
Associate Advisor
Other advisors: Associate Professor Fatima Nasrallah
-
Doctor Philosophy
Development of a model for prognostication of patient outcome following traumatic brain injury
Associate Advisor
Other advisors: Associate Professor Fatima Nasrallah
-
Doctor Philosophy
Machine learning methods for visualisation and quantification of nephrons with MRI.
Associate Advisor
Other advisors: Dr Nyoman Kurniawan
-
Doctor Philosophy
Advanced Deep Learning Approaches for Improving Diagnosis and Prognosis in Brain Disease
Associate Advisor
Other advisors: Associate Professor Fatima Nasrallah
-
Doctor Philosophy
Using 3D total body imaging to study the spatial distribution of naevi and melanoma
Associate Advisor
Other advisors: Professor Peter Soyer, Professor Monika Janda
-
Doctor Philosophy
New deep learning based methods for assessment of sleep apnea severity and risk of related short and long term health consequences
Associate Advisor
Other advisors: Professor Juha Toyras, Associate Professor Philip Terrill
-
Doctor Philosophy
Artifical intelligence approaches for diagnosing and phenotyping sleep disorders
Associate Advisor
Other advisors: Professor Juha Toyras, Associate Professor Philip Terrill
-
Doctor Philosophy
Virtual Agricultural Imaging and Sensing through Artificial Intelligence and Computer Vision
Associate Advisor
Other advisors: Professor Scott Chapman
-
Doctor Philosophy
Establishment of a National Anterior Cruciate Ligament (ACL) Registry in Australia
Associate Advisor
Other advisors: Associate Professor Craig Engstrom
-
Doctor Philosophy
New deep learning based methods for assessment of sleep apnea severity and risk of related short and long term health consequences
Associate Advisor
Other advisors: Professor Juha Toyras, Associate Professor Philip Terrill
Completed supervision
-
2024
Doctor Philosophy
Deployable and Interpretable Deep Learning Architectures: Navigating Clinical Challenges in Medical Image Segmentation
Principal Advisor
Other advisors: Associate Professor Craig Engstrom, Emeritus Professor Stuart Crozier
-
2024
Doctor Philosophy
Efficient Image Representations for Compressed Sensing MRI
Principal Advisor
Other advisors: Associate Professor Craig Engstrom, Professor Feng Liu, Professor Markus Barth
-
2024
Doctor Philosophy
Deep Learning Strategies for Enhanced mTBI Diagnosis using Clinical and CT Data
Principal Advisor
-
2024
Doctor Philosophy
Towards Analysis of Contextual Melanoma Indicators and Identification of Total-Body Ugly Duckling Lesions with Deep Neural Networks
Principal Advisor
Other advisors: Associate Professor Mahsa Baktashmotlagh
-
2023
Doctor Philosophy
Towards Efficient Graph Neural Networks for Optimizing Illicit Dark Web Interventions
Principal Advisor
Other advisors: Professor Marius Portmann
-
2022
Doctor Philosophy
Automated Assessment of Cartilage Composition and Cam Morphology using Magnetic Resonance Images of the Hip Joint
Principal Advisor
Other advisors: Associate Professor Craig Engstrom, Emeritus Professor Stuart Crozier
-
2020
Doctor Philosophy
Computer aided lesion detection, segmentation and characterization on mammography and breast MRI
Principal Advisor
Other advisors: Emeritus Professor Stuart Crozier
-
2023
Doctor Philosophy
In vitro evaluation of porous PHBV-based scaffolds for tissue regeneration application
Associate Advisor
Other advisors: Dr Mingyuan Lu
-
2022
Doctor Philosophy
Improving deep learning-based fast MRI with pre-acquired image guidance
Associate Advisor
Other advisors: Emeritus Professor Stuart Crozier, Professor Feng Liu
Media
Enquiries
For media enquiries about Dr Shakes Chandra's areas of expertise, story ideas and help finding experts, contact our Media team: