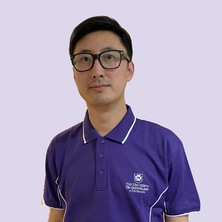
Overview
Background
Dr Hongfu Sun completed his PhD in Biomedical Engineering at the University of Alberta in 2015, followed by postdoctoral training in Calgary until 2018. He joined the Imaging, Sensing and Biomedical Engineering team in the School of ITEE at UQ in 2019 and was awarded the ARC DECRA fellowship in 2021. His research interests include developing novel magnetic resonance imaging (MRI) contrast mechanisms, e.g. Quantitative Susceptibility Mapping (QSM), fast and multi-parametric MRI acquisitions, and advanced image reconstruction techniques, including deep learning and artificial intelligence, to advance medical imaging techniques for clinical applications.
Dr Sun is currently recruiting graduate students. Check out Available Projects for details. Open to both Domestic and International students.
Availability
- Dr Hongfu Sun is:
- Available for supervision
Fields of research
Qualifications
- Doctor of Philosophy, University of Alberta
Research interests
-
MR image processing through advanced optimization techniques and deep learning
My research interest is to develop advanced image reconstruction and processing methods to solve some of the mathematical challenges in MRI research, such as the ill-posed inverse problem in Quantitative Susceptibility Mapping (QSM). Some of the approaches I am particularly interested in are (1) image optimization techniques such as image regularization and compressed sensing, and (2) machine learning and especially deep learning through the convolutional neural network (CNN) and transformers in vision.
-
Fast, multi-parametric, and quantitative MRI acquisition methods at ultra-high field
A single MRI acquisition usually takes 3-5 minutes, and a standard clinical protocol may require a couple of them for complementary contrasts. My research interest is to significantly reduce the total scan time for each patient while maintaining the same amount of information for diagnosis, by (1) accelerating individual scans with parallel imaging techniques at ultra-high field, and (2) designing novel MRI sequences that can produce multi-contrast weighted images and multi-parametric quantitative maps from a single MR acquisition.
-
Brain imaging applications in neuroscience and neurological diseases
MRI is one of the best tools to study the brain in vivo, thanks to its excellent soft tissue contrast and its versatile contrast mechanisms. I am interested in applying advanced and comprehensive image analysis on different MRI methods to better understand neuroscience, such as brain development in children and structural and functional connectivity of the brain, as well as some of the neurological diseases such as Alzheimer/Dementia, Multiple Sclerosis, Schizophrenia, and Stroke.
Research impacts
Dr Hongfu Sun is one of the early pioneers in developing a novel MRI technique - Quantitative Susceptibility Mapping (QSM), which is one of the most significant MRI contrast breakthroughs in recent years, that has demonstrated wide clinical applications in healthy, aging and diseased human brains, such as dementia, Alzheimer's disease, Parkinson's disease, multiple sclerosis, schizophrenia, stroke, etc. Since commencing at UQ, Dr Sun has extended his research topics to exploiting novel reconstruction algorithms using state-of-the-art deep learning-based artificial intelligence techniques.
Works
Search Professor Hongfu Sun’s works on UQ eSpace
Featured
2020
Journal Article
Editorial for “Deep‐Learning Detection of Cancer Metastasis to the Brain on MRI”
Sun, Hongfu (2020). Editorial for “Deep‐Learning Detection of Cancer Metastasis to the Brain on MRI”. Journal of Magnetic Resonance Imaging, 52 (4) jmri.27131, 1237-1238. doi: 10.1002/jmri.27131
Featured
2019
Journal Article
Extracting more for less: multi‐echo MP2RAGE for simultaneous T 1 ‐weighted imaging, T 1 mapping, mapping, SWI, and QSM from a single acquisition
Sun, Hongfu, Cleary, Jon O., Glarin, Rebecca, Kolbe, Scott C., Ordidge, Roger J., Moffat, Bradford A. and Pike, G. Bruce (2019). Extracting more for less: multi‐echo MP2RAGE for simultaneous T 1 ‐weighted imaging, T 1 mapping, mapping, SWI, and QSM from a single acquisition. Magnetic Resonance in Medicine, 83 (4) mrm.27975, 1178-1191. doi: 10.1002/mrm.27975
Featured
2018
Journal Article
Whole head quantitative susceptibility mapping using a least-norm direct dipole inversion method
Sun, Hongfu, Ma, Yuhan, MacDonald, M. Ethan and Pike, G. Bruce (2018). Whole head quantitative susceptibility mapping using a least-norm direct dipole inversion method. NeuroImage, 179, 166-175. doi: 10.1016/j.neuroimage.2018.06.036
Featured
2018
Journal Article
Quantitative susceptibility mapping for following intracranial hemorrhage
Sun, Hongfu, Klahr, Ana C., Kate, Mahesh, Gioia, Laura C., Emery, Derek J., Butcher, Kenneth S. and Wilman, Alan H. (2018). Quantitative susceptibility mapping for following intracranial hemorrhage. Radiology, 288 (3), 830-839. doi: 10.1148/radiol.2018171918
Featured
2017
Journal Article
Structural and functional quantitative susceptibility mapping from standard fMRI studies
Sun, H., Seres, P. and Wilman, A. H. (2017). Structural and functional quantitative susceptibility mapping from standard fMRI studies. NMR in Biomedicine, 30 (4) e3619, e3619. doi: 10.1002/nbm.3619
Featured
2016
Journal Article
Quantitative susceptibility mapping using a superposed dipole inversion method: Application to intracranial hemorrhage
Sun, Hongfu, Kate, Mahesh, Gioia, Laura C., Emery, Derek J., Butcher, Kenneth and Wilman, Alan H. (2016). Quantitative susceptibility mapping using a superposed dipole inversion method: Application to intracranial hemorrhage. Magnetic Resonance in Medicine, 76 (3), 781-791. doi: 10.1002/mrm.25919
Featured
2015
Journal Article
Quantitative susceptibility mapping using single-shot echo-planar imaging
Sun, Hongfu and Wilman, Alan H. (2015). Quantitative susceptibility mapping using single-shot echo-planar imaging. Magnetic Resonance in Medicine, 73 (5), 1932-1938. doi: 10.1002/mrm.25316
Featured
2015
Journal Article
Validation of quantitative susceptibility mapping with Perls' iron staining for subcortical gray matter
Sun, Hongfu, Walsh, Andrew J., Lebel, R. Marc, Blevins, Gregg, Catz, Ingrid, Lu, Jian-Qiang, Johnson, Edward S., Emery, Derek J., Warren, Kenneth G. and Wilman, Alan H. (2015). Validation of quantitative susceptibility mapping with Perls' iron staining for subcortical gray matter. NeuroImage, 105, 486-492. doi: 10.1016/j.neuroimage.2014.11.010
Featured
2014
Journal Article
Background field removal using spherical mean value filtering and Tikhonov regularization
Sun, Hongfu and Wilman, Alan H. (2014). Background field removal using spherical mean value filtering and Tikhonov regularization. Magnetic Resonance in Medicine, 71 (3), 1151-1157. doi: 10.1002/mrm.24765
2025
Journal Article
Quantitative susceptibility mapping via deep neural networks with iterative reverse concatenations and recurrent modules
Li, Min, Chen, Chen, Xiong, Zhuang, Liu, Yin, Rong, Pengfei, Shan, Shanshan, Liu, Feng, Sun, Hongfu and Gao, Yang (2025). Quantitative susceptibility mapping via deep neural networks with iterative reverse concatenations and recurrent modules. Medical Physics, 52 (6), 4341-4354. doi: 10.1002/mp.17747
2025
Journal Article
MRF-mixer: a simulation-based deep learning framework for accelerated and accurate magnetic resonance fingerprinting reconstruction
Ding, Tianyi, Gao, Yang, Xiong, Zhuang, Liu, Feng, Cloos, Martijn A. and Sun, Hongfu (2025). MRF-mixer: a simulation-based deep learning framework for accelerated and accurate magnetic resonance fingerprinting reconstruction. Information, 16 (3) 218, 218. doi: 10.3390/info16030218
2024
Conference Publication
Fast controllable diffusion models for undersampled MRI reconstruction
Jiang, Wei, Xiong, Zhuang, Liu, Feng, Ye, Nan and Sun, Hongfu (2024). Fast controllable diffusion models for undersampled MRI reconstruction. 2024 IEEE International Symposium on Biomedical Imaging (ISBI), Athens, Greece, 27-30 May 2024. Piscataway, NJ, United States: IEEE. doi: 10.1109/isbi56570.2024.10635891
2024
Journal Article
Quantitative susceptibility mapping through model-based deep image prior (MoDIP)
Xiong, Zhuang, Gao, Yang, Liu, Yin, Fazlollahi, Amir, Nestor, Peter, Liu, Feng and Sun, Hongfu (2024). Quantitative susceptibility mapping through model-based deep image prior (MoDIP). NeuroImage, 291 120583, 120583. doi: 10.1016/j.neuroimage.2024.120583
2024
Journal Article
Plug-and-Play latent feature editing for orientation-adaptive quantitative susceptibility mapping neural networks
Gao, Yang, Xiong, Zhuang, Shan, Shanshan, Liu, Yin, Rong, Pengfei, Li, Min, Wilman, Alan H., Pike, G. Bruce, Liu, Feng and Sun, Hongfu (2024). Plug-and-Play latent feature editing for orientation-adaptive quantitative susceptibility mapping neural networks. Medical Image Analysis, 94 103160, 1-11. doi: 10.1016/j.media.2024.103160
2024
Journal Article
A dual-responsive microemulsion with macroscale superlubricity and largely switchable friction
Chen, Siwei, Sun, Hong, Liu, Jian, Wang, Jinyu, Lu, Hongsheng, Hao, Jingcheng, Xu, Lu and Liu, Weimin (2024). A dual-responsive microemulsion with macroscale superlubricity and largely switchable friction. Materials Horizons, 11 (7), 1668-1678. doi: 10.1039/d3mh01978k
2024
Conference Publication
QSM Reconstruction of Arbitrary Dipole Orientations using an End-to-end Neural Network via Latent Feature Editing
Gao, Yang, Xiong, Zhuang, Shan, Shanshan, Li, Min, Wilman, Alan H, Pike, G. Bruce, Liu, Feng and Sun, Hongfu (2024). QSM Reconstruction of Arbitrary Dipole Orientations using an End-to-end Neural Network via Latent Feature Editing. 2024 ISMRM & ISMRT Annual Meeting, Singapore, 4-9 May 2024. Concord, CA United States: ISMRM. doi: 10.58530/2024/2453
2023
Journal Article
Editorial: Imaging of neurometabolism
Zheng, Wenbin, Dai, Zhouzhi, Wu, Renhua and Sun, Hongfu (2023). Editorial: Imaging of neurometabolism. Frontiers in Neuroscience, 17 1286361, 1286361. doi: 10.3389/fnins.2023.1286361
2023
Journal Article
MapFlow: latent transition via normalizing flow for unsupervised domain adaptation
Askari, Hossein, Latif, Yasir and Sun, Hongfu (2023). MapFlow: latent transition via normalizing flow for unsupervised domain adaptation. Machine Learning, 112 (8), 2953-2974. doi: 10.1007/s10994-023-06357-2
2023
Journal Article
Increased glymphatic system activity in patients with mild traumatic brain injury
Dai, Zhuozhi, Yang, Zhiqi, Li, Zhaolin, Li, Mu, Sun, Hongfu, Zhuang, Zerui, Yang, Weichao, Hu, Zehuan, Chen, Xiaofeng, Lin, Daiying and Wu, Xianheng (2023). Increased glymphatic system activity in patients with mild traumatic brain injury. Frontiers in Neurology, 14 1148878, 1-7. doi: 10.3389/fneur.2023.1148878
2023
Journal Article
Distortion‐corrected image reconstruction with deep learning on an MRI‐Linac
Shan, Shanshan, Gao, Yang, Liu, Paul Z. Y., Whelan, Brendan, Sun, Hongfu, Dong, Bin, Liu, Feng and Waddington, David E. J. (2023). Distortion‐corrected image reconstruction with deep learning on an MRI‐Linac. Magnetic Resonance in Medicine, 90 (3), 1-15. doi: 10.1002/mrm.29684
Funding
Current funding
Past funding
Supervision
Availability
- Dr Hongfu Sun is:
- Available for supervision
Before you email them, read our advice on how to contact a supervisor.
Available projects
-
MRI and deep learning methods development and applications at ultra-high field
I am currently recruiting Master and PhD students to innovate on novel MRI methods and deep learning image reconstruction techniques that can be eventually applied to neuroscience and neurological diseases. We have an excellent and accessible MRI facility here at UQ, e.g. a state-of-the-art 3T Prisma and a prestigious 7T whole-body system (only two in Australia, the other one in UniMelb). The research projects will involve MRI physics, pulse sequence programming, image processing (e.g. deep learning), and image analysis. By the end of your graduate study, you will be an expert in MRI with comprehensive skills in maths, physics, computer programming, and artificial intelligence.
https://graduate-school.uq.edu.au/project/developing-ai-based-mri-methods-microscopic-imaging
Supervision history
Current supervision
-
Doctor Philosophy
MR image processing through advanced optimisation techniques and deep learning
Principal Advisor
Other advisors: Professor Feng Liu
-
Doctor Philosophy
MR image processing through advanced optimisation techniques and deep learning
Principal Advisor
Other advisors: Professor Feng Liu
-
Doctor Philosophy
Robust and generalizable deep Learning quantitative susceptibility mapping for human brains
Principal Advisor
Other advisors: Professor Feng Liu
-
Doctor Philosophy
Development of novel deep learning methods for medical imaging
Principal Advisor
Other advisors: Professor Feng Liu, Dr Nan Ye
-
Doctor Philosophy
MRI methods development through deep learning
Principal Advisor
Other advisors: Professor Feng Liu
-
Doctor Philosophy
Magnetic Resonance Image Processing with Artificial Intelligence
Associate Advisor
Other advisors: Associate Professor Craig Engstrom, Dr Shakes Chandra
-
Doctor Philosophy
Combined Compressed sensing and machine learning/deep learning methods for rapid MRI
Associate Advisor
Other advisors: Professor Kwun Fong, Associate Professor Henry Marshall, Professor Feng Liu
Completed supervision
-
2024
Doctor Philosophy
Key Applications in Deep Learning Based Quantitative Susceptibility Mapping
Associate Advisor
Other advisors: Emeritus Professor Stuart Crozier, Professor Feng Liu
-
2022
Doctor Philosophy
Deep Learning-based Quantitative Susceptibility Mapping: Methods Development and Applications
Associate Advisor
Other advisors: Emeritus Professor Stuart Crozier, Professor Feng Liu
Media
Enquiries
For media enquiries about Dr Hongfu Sun's areas of expertise, story ideas and help finding experts, contact our Media team: