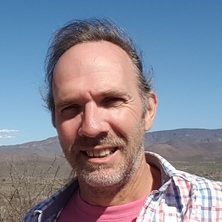
Scott Chapman
- Email:
- scott.chapman@uq.edu.au
- Phone:
- +61 7 54601 108
- Phone:
- +61 7 54601 152
Overview
Background
Summary of Research:
- My current research at UQ is as Professor in this School (teaching AGRC3040 Crop Physiology) and as an Affiliate Professor of QAAFI. Since 2020, with full-time appointment at UQ, my research portfolio has included multiple projects in applications of machine learning and artificial intelligence into the ag domain. This area is developing rapidly and across UQ, I am engaging with faculty in multiple schools (ITEE, Maths and Physics, Mining and Mech Engineering) as well as in the Research Computing Centre to develop new projects and training opportunities at the interface of field agriculture and these new digital analytics.
- My career research has been around genetic and environment effects on physiology of field crops, particularly where drought dominates. Application of quantitative approaches (crop simulation and statistical methods) and phenotyping (aerial imaging, canopy monitoring) to integrate the understanding of interactions of genetics, growth and development and the bio-physical environment on crop yield. In recent years, this work has expanded more generally into various applications in digital agriculture from work on canopy temperature sensing for irrigation decisions (CSIRO Entrepreneurship Award 2022) through to applications of deep-learning to imagery to assist breeding programs.
- Much of this research was undertaken with CSIRO since 1996. Building on an almost continuous collaboration with UQ over that time, including as an Adjunct Professor to QAAFI, Prof Chapman was jointly appointed (50%) as a Professor in Crop Physiology in the UQ School of Agriculture and Food Sciences from 2017 to 2020, and at 100% with UQ from Sep 2020. He has led numerous research projects that impact local and global public and private breeding programs in wheat, sorghum, sunflower and sugarcane; led a national research program on research in ‘Climate-Ready Cereals’ in the early 2010s; and was one of the first researchers to deploy UAV technologies to monitor plant breeding programs. Current projects include a US DoE project with Purdue University, and multiple projects with CSIRO, U Adelaide, La Trobe, INRA (France) and U Tokyo. With > 8500 citations, Prof Chapman is currently in the top 1% of authors cited in the ESI fields of Plant and Animal Sciences and in Agricultural Sciences.
Availability
- Professor Scott Chapman is:
- Available for supervision
- Media expert
Fields of research
Qualifications
- Bachelor (Honours), The University of Queensland
- Doctor of Philosophy, The University of Queensland
Research interests
-
Applications of deep learning in crop phenotyping
-
Use of simulation models in plant breeding programs and managing climate change
-
Deployment of IoT, UAV and remote sensing technologies in research and commercial field scales
Research impacts
Optimization of genotype evaluation methods in breeding programs
- By 2005, completed two sugarcane projects that radically changed the priorities and evaluation methods of Australian breeding programs such that the delivery of new varieties now happens 3 to 5 years earlier. The major outcome was a confidential industry report. Supervised similar research for Advanta sunflower breeding in Argentina to reorganise and accelerate preliminary testing program.
- Led the public sector’s most extensive global collaborative study of wheat variety performance (>200 trials). This has assisted the delivery of better spring-wheat varieties into developing countries and into Australia.
- Extended research to use “environment characterization”, which I co-developed in the late 90s. The basic methodology to better identify stable varieties in the face of drought stress, has been adopted by international seed companies and local breeding programs in a range of crops.
- From 2009 to 2017, led the development of applications of ‘Pheno-Copter’ autonomous aerial robot platform at CSIRO based on hardware and software processing systems to allow capture and analysis of high-throughput image information from field crop experiments in wheat, sorghum, sugarcane and cotton.
- Since 2019/2020, have begun to lead two new research projects funded by GRDC involving both UQ and CSIRO. One project (AG-FE-ML) with partners in France (INRAe/ARVALIS) and Japan (U Tokyo) is in the applications of deep learning/feature extraction on agricultural imagery to allow automated segmentation of plant parts from images and to enable counting of reproductive structures (heads/panicles/grains) that are associated with grain yield of crops. The second project (INVITA) is applying a range of technologies (in-field sensors, cameras, satellite imagery, computer simulation) and methods (multi-variate statistics and machine learning) to attempt to improve the prediction of differences in yields among crop genotypes in the National Variety Trials. This research aims to allow the interpolation of results across the national production areas.
Exploiting crop adaptation traits through experiments and simulation studies
- Supervised and co-investigated to demonstrate the adaptive yield and quality value of major wheat genes around the world (dwarfing and disease genes) and across Australia (water soluble carbohydrates, transpiration efficiency and tillering genes)
- As a co-investigator, developed a unique platform (to the public sector) in the simulation modelling of crop growth and plant breeding programs. This platform has attracted >$6 million co-investment (ARC and private company) and provides the full capability to model the breeding systems of major crops. It continues development in the current ARC CoE for Plant Success.
- Co-published pioneering research on the simulation of genetic controls of leaf growth processes within crop models. This original contribution has opened novel opportunities for the high-throughput simulation, testing and improvement of fully-specified physiological, breeding and statistical methodologies that are applied in plant breeding.
- As lead PI (wheat) and co-PI (sorghum), ran experiments and improved models to analyse potential of genetic variation in heat tolerance to cope with current and future climates in Australian environments.
Works
Search Professor Scott Chapman’s works on UQ eSpace
2025
Journal Article
Unmanned aerial vehicle phenotyping of agronomic and physiological traits in mungbean
Van Haeften, Shanice, Smith, Daniel, Robinson, Hannah, Dudley, Caitlin, Kang, Yichen, Douglas, Colin A., Hickey, Lee T., Potgieter, Andries, Chapman, Scott and Smith, Millicent R. (2025). Unmanned aerial vehicle phenotyping of agronomic and physiological traits in mungbean. The Plant Phenome Journal, 8 (1) e70016, 1-18. doi: 10.1002/ppj2.70016
2025
Journal Article
Integrating yield gap analysis to capture genotype by environment by management interactions for Australian broadacre sorghum cropping systems
Garba, Ismail I., Fernandez, Javier A., Chen, Qiaomin, Gho, Carla, deVoil, Peter, Cooper, Mark and Chapman, Scott C. (2025). Integrating yield gap analysis to capture genotype by environment by management interactions for Australian broadacre sorghum cropping systems. Field Crops Research, 326 109858, 109858. doi: 10.1016/j.fcr.2025.109858
2025
Journal Article
From soil health to agricultural productivity: The critical role of soil constraint management
Li, Tong, Cui, Lizhen, Filipović, Vilim, Tang, Caixian, Lai, Yunru, Wehr, Bernhard, Song, Xiufang, Chapman, Scott, Liu, Hongdou, Dalal, Ram C. and Dang, Yash P. (2025). From soil health to agricultural productivity: The critical role of soil constraint management. Catena, 250 108776, 1-15. doi: 10.1016/j.catena.2025.108776
2025
Journal Article
Multimodal sequential cross-modal transformer for predicting plant available water capacity (PAWC) from time series of weather and crop biological data
Nguyen, Dung, de Voil, Peter, Potgieter, Andries, Dang, Yash P., Orton, Thomas G., Nguyen, Duc Thanh, Nguyen, Thanh Thi and Chapman, Scott C. (2025). Multimodal sequential cross-modal transformer for predicting plant available water capacity (PAWC) from time series of weather and crop biological data. Agricultural Water Management, 307 109124, 1-12. doi: 10.1016/j.agwat.2024.109124
2024
Journal Article
Prediction accuracy and repeatability of UAV based biomass estimation in wheat variety trials as affected by variable type, modelling strategy and sampling location
Smith, Daniel T. L., Chen, Qiaomin, Massey-Reed, Sean Reynolds, Potgieter, Andries B. and Chapman, Scott C. (2024). Prediction accuracy and repeatability of UAV based biomass estimation in wheat variety trials as affected by variable type, modelling strategy and sampling location. Plant Methods, 20 (1) 129. doi: 10.1186/s13007-024-01236-w
2024
Conference Publication
Multivariate characterisation of envirotypes for wheat G×E in Australian Variety Trials
Fernandez, Javier A., Hu, Pengcheng, Arief, Vivi, Gho, Carla, Ramakers, Jip, Hemerik, Jesse, Boer, Martin, van Eeuwijk, Fred and Chapman, Scott (2024). Multivariate characterisation of envirotypes for wheat G×E in Australian Variety Trials. GxExM Symposium III, Wageningen, Netherlands, 30-31 October 2024.
2024
Conference Publication
Assessing crop water use in wheat and sorghum National Variety Trials in Australia
Fernandez, J. A., Gho, C., Smith, D., Zheng, B. and Chapman, S.C. (2024). Assessing crop water use in wheat and sorghum National Variety Trials in Australia. 21st Australian Society of Agronomy Conference, Albany, WA, Australia, 21-24 October 2024. Australian Society of Agronomy.
2024
Conference Publication
Environment characterisation of wheat national variety trials in Australia
Fernandez, Javier A., Arief, Vivi, Hu, Pengcheng, Zheng, Bangyou, Gho, Carla, Ramakers, Jip, Boer, Martin, van Eeuwijk, Fred and Chapman, Scott (2024). Environment characterisation of wheat national variety trials in Australia. 3rd International Wheat Congress, Perth, WA, Australia, 22-27 September 2024.
2024
Journal Article
Estimating aboveground biomass dynamics of wheat at small spatial scale by integrating crop growth and radiative transfer models with satellite remote sensing data
Hu, Pengcheng, Zheng, Bangyou, Chen, Qiaomin, Grunefeld, Swaantje, Choudhury, Malini Roy, Fernandez, Javier, Potgieter, Andries and Chapman, Scott C. (2024). Estimating aboveground biomass dynamics of wheat at small spatial scale by integrating crop growth and radiative transfer models with satellite remote sensing data. Remote Sensing of Environment, 311 114277, 1-15. doi: 10.1016/j.rse.2024.114277
2024
Conference Publication
Insights in the ability of high-resolution narrow band multispectral and thermal sensors to estimate cotton production in Australia
Devoto, F., Reynolds-Massey-Reed, S., Segura, Pinzon C., Bell, M., Mclaren, T., Awale, R., Camino, C., Bange, M., Woodgate, W., Chapman, S. and Potgieter, A. B. (2024). Insights in the ability of high-resolution narrow band multispectral and thermal sensors to estimate cotton production in Australia. 2024 IEEE International Geoscience and Remote Sensing Symposium, Athens, Greece, 7-12 July 2024. Piscataway, NJ, United States: IEEE. doi: 10.1109/igarss53475.2024.10642663
2024
Other Outputs
Enhancing crop trial data to strengthen variety decisions
Fernandez, Javier and Chapman, Scott (2024, 07 01). Enhancing crop trial data to strengthen variety decisions GRDC GroundCover Supplement: Analytics, Data and Phenomics 12-13.
2024
Journal Article
Seasonal dynamics of fallow and cropping lands in the broadacre cropping region of Australia
Xie, Zunyi, Zhao, Yan, Jiang, Ruizhu, Zhang, Miao, Hammer, Graeme, Chapman, Scott, Brider, Jason and Potgieter, Andries B. (2024). Seasonal dynamics of fallow and cropping lands in the broadacre cropping region of Australia. Remote Sensing of Environment, 305 114070, 1-14. doi: 10.1016/j.rse.2024.114070
2024
Journal Article
GrainPointNet: a deep-learning framework for non-invasive sorghum panicle grain count phenotyping
James, Chrisbin, Smith, Daniel, He, Weigao, Chandra, Shekhar S. and Chapman, Scott C. (2024). GrainPointNet: a deep-learning framework for non-invasive sorghum panicle grain count phenotyping. Computers and Electronics in Agriculture, 217 108485, 108485. doi: 10.1016/j.compag.2023.108485
2023
Journal Article
Preliminary results in innovative solutions for soil carbon estimation: integrating remote sensing, machine learning, and proximal sensing spectroscopy
Li, Tong, Xia, Anquan, McLaren, Timothy I., Pandey, Rajiv, Xu, Zhihong, Liu, Hongdou, Manning, Sean, Madgett, Oli, Duncan, Sam, Rasmussen, Peter, Ruhnke, Florian, Yüzügüllü, Onur, Fajraoui, Noura, Beniwal, Deeksha, Chapman, Scott, Tsiminis, Georgios, Smith, Chaya, Dalal, Ram C. and Dang, Yash P. (2023). Preliminary results in innovative solutions for soil carbon estimation: integrating remote sensing, machine learning, and proximal sensing spectroscopy. Remote Sensing, 15 (23) 5571, 1-17. doi: 10.3390/rs15235571
2023
Conference Publication
Toward a unified framework for RGB and RGB-D visual navigation
Du, Heming, Huang, Zi, Chapman, Scott and Yu, Xin (2023). Toward a unified framework for RGB and RGB-D visual navigation. 36th Australasian Joint Conference on Artificial Intelligence, AJCAI 2023, Brisbane, QLD Australia, 28 November –1 December 2023. Singapore: Springer. doi: 10.1007/978-981-99-8391-9_29
2023
Other Outputs
Capacity building and knowledge transfer in seaweed mapping in Indonesia
Abdul Aziz, Ammar, Wicaksono, Prama, Arjasakusuma, Sanjiwana, Chapman, Scott, Langford, Zannie, Grunefeld, Swaantje, Azizan, Fathin Ayuni and Maishella, Amanda (2023). Capacity building and knowledge transfer in seaweed mapping in Indonesia. Melbourne, VIC, Australia: The Australia-Indonesia Centre.
2023
Conference Publication
Advances in the study of biochemical, morphological and physiological traits of wheat and sorghum crops in australia using hyperspectral data and machine learning
Potgieter, A. B., Camino, C., Poblete, T., Zhi, X., Reynolds-Massey-Reed, S., Zhao, Y., Belwalkar, A., Ruizhu, J., George-Jaeggli, B., Chapman, S., Jordan, D., Wu, A., Hammer, G. L. and J, Zarco-Tejada P. (2023). Advances in the study of biochemical, morphological and physiological traits of wheat and sorghum crops in australia using hyperspectral data and machine learning. 2023 IEEE International Geoscience and Remote Sensing Symposium, Pasadena, CA USA, 16-21 July 2023. Piscataway, NJ USA: Institute of Electrical and Electronics Engineers. doi: 10.1109/igarss52108.2023.10282230
2023
Journal Article
Modelling the impacts of diverse cover crops on soil water and nitrogen and cash crop yields in a sub-tropical dryland
Garba, Ismail I., Bell, Lindsay W., Chapman, Scott, deVoil, Peter, Kamara, Alpha Y. and Williams, Alwyn (2023). Modelling the impacts of diverse cover crops on soil water and nitrogen and cash crop yields in a sub-tropical dryland. Field Crops Research, 301 109019, 1-14. doi: 10.1016/j.fcr.2023.109019
2023
Journal Article
Utilisation of unmanned aerial vehicle imagery to assess growth parameters in mungbean (Vigna radiata (L.) Wilczek)
Xiong, Yiyi, Chiau, Lucas Mauro Rogerio, Wenham, Kylie, Collins, Marisa and Chapman, Scott C. (2023). Utilisation of unmanned aerial vehicle imagery to assess growth parameters in mungbean (Vigna radiata (L.) Wilczek). Crop and Pasture Science, 75 (1) CP22335CO. doi: 10.1071/cp22335
2023
Conference Publication
Predicting yield in multi-environment breeding trials using penalized regression and multiple-covariate random-regression models
Ramakers, Jip, Hemerik, Jesse, Boer, Martin, Bustos-Korts, Daniela, Fernandez, Javier A., Pengcheng Hu, Noviati, Vivi, Chapman, Scott and van Eeuwijk, Fred (2023). Predicting yield in multi-environment breeding trials using penalized regression and multiple-covariate random-regression models. Channel Network Conference 2023, Wageningen, Netherlands, 23-25 August 2023.
Funding
Current funding
Supervision
Availability
- Professor Scott Chapman is:
- Available for supervision
Before you email them, read our advice on how to contact a supervisor.
Available projects
-
See Research Interests
We have multiple opportunities for agricultural and maths/IT/engineering students to enrol or be co-supervised in research with our teams.
Please contact me or carla.gho@uq.edu.au
Supervision history
Current supervision
-
Doctor Philosophy
Virtual Agricultural Imaging and Sensing through Artificial Intelligence and Computer Vision
Principal Advisor
Other advisors: Dr Shakes Chandra
-
Doctor Philosophy
Using phenotyping and modelling methods to improve estimation of crop performance in variety trials
Principal Advisor
Other advisors: Associate Professor Andries Potgieter
-
Doctor Philosophy
Estimating biomass and radiation-use-efficiency in wheat variety trials using unmanned aerial vehicles
Principal Advisor
Other advisors: Associate Professor Andries Potgieter
-
Doctor Philosophy
Utilizing Remote Sensing and Machine Learning to Detect Plantation Trees Infected by Fungal Diseases
Associate Advisor
Other advisors: Associate Professor Anthony Young, Professor Ammar Abdul Aziz
-
Doctor Philosophy
Determining the effects of abiotic stress on crop growth development, and yield under different nitrogen applications using remotely sensed data for cotton and wheat.
Associate Advisor
Other advisors: Dr William Woodgate, Associate Professor Andries Potgieter
-
Doctor Philosophy
Enhancing Plant Phenotyping Accuracy through Analysing Video Data
Associate Advisor
Other advisors: Dr Yadan Luo, Associate Professor Mahsa Baktashmotlagh
-
Doctor Philosophy
Evaluating Diverse Taro (Colocasia) Germplasm to Enhance Food Security and Climate Resilience in the Pacific Islands
Associate Advisor
Other advisors: Professor Ian Godwin, Dr Eric Dinglasan, Dr Millicent Smith, Dr Bradley Campbell
Completed supervision
-
2023
Doctor Philosophy
In-season phenotyping of crop growth via the integration of imaging, modelling, and machine learning
Principal Advisor
Other advisors: Associate Professor Karine Chenu
-
2023
Doctor Philosophy
Cover cropping in drylands for improved agronomic and environmental outcomes
Associate Advisor
Other advisors: Professor Bhagirath Chauhan, Dr Alwyn Williams
-
2022
Doctor Philosophy
Climatic and epidemiological characterisation of new rubber leaf fall disease: A remote sensing approach
Associate Advisor
Other advisors: Associate Professor Anthony Young, Professor Ammar Abdul Aziz
-
2022
Doctor Philosophy
High-throughput phenotyping using UAV thermal imaging integrated with field experiments and statistical modelling techniques to quantify water use of wheat genotypes on rain-fed sodic soils
Associate Advisor
Other advisors: Dr Yash Dang
-
2022
Doctor Philosophy
High-throughput phenotyping and spatial modelling to aid understanding of wheat genotype adaptation on sodic soils
Associate Advisor
Other advisors: Dr Yash Dang
-
2010
Doctor Philosophy
Evaluation of reduced-tillering (tin gene) wheat lines for water limiting environments in northern Australia
Associate Advisor
Other advisors: Emeritus Professor Shu Fukai
-
2007
Doctor Philosophy
AN INVESTIGATION INTO THE GENETICS AND PHYSIOLOGY OF SUGAR ACCUMULATION IN SWEET SORGHUM AS A POTENTIAL MODEL FOR SUGARCANE
Associate Advisor
Other advisors: Professor Ian Godwin, Professor David Jordan
-
2003
Doctor Philosophy
QUANTIFYING NITROGEN EFFECT IN CROP GROWTH PROCESS IN SUNFLOWER AND MAIZE
Associate Advisor
Other advisors: Professor Graeme Hammer, Emeritus Professor Shu Fukai
Media
Enquiries
Contact Professor Scott Chapman directly for media enquiries about:
- ag tech
- climate change and crop production
- crop science
- digital agriculture
Need help?
For help with finding experts, story ideas and media enquiries, contact our Media team: