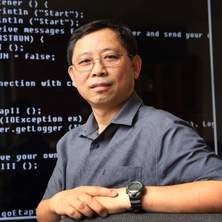
Overview
Availability
- Professor Xue Li is:
- Not available for supervision
Fields of research
Qualifications
- Bachelor of Science, Chongqing University
- Masters (Research) of Science, The University of Queensland
- Doctor of Philosophy, Queensland University of Technology
Works
Search Professor Xue Li’s works on UQ eSpace
2025
Journal Article
High-order neighbors aware representation learning for knowledge graph completion
Yin, Hong, Zhong, Jiang, Li, Rongzhen, Shang, Jiaxing, Wang, Chen and Li, Xue (2025). High-order neighbors aware representation learning for knowledge graph completion. IEEE Transactions on Neural Networks and Learning Systems, 36 (3), 5273-5287. doi: 10.1109/tnnls.2024.3383873
2025
Journal Article
Document-level relation extraction via commonsense knowledge enhanced graph representation learning
Dai, Qizhu, Li, Rongzhen, Xue, Zhongxuan, Li, Xue and Zhong, Jiang (2025). Document-level relation extraction via commonsense knowledge enhanced graph representation learning. Applied Intelligence, 55 (2) 165, 1-13. doi: 10.1007/s10489-024-05985-y
2025
Conference Publication
Recent advances on multi-modal dialogue systems: a survey
Cheng, Fenghua, Li, Xue, Wu, Haoyang, Sang, Jiangcheng and Zhao, Wenqi (2025). Recent advances on multi-modal dialogue systems: a survey. 20th International Conference, ADMA 2024, Sydney, NSW, Australia, 3 - 5 December 2024. Singapore, Singapore: Springer Nature Singapore. doi: 10.1007/978-981-96-0847-8_3
2025
Conference Publication
Automatic summarization of life-critical situations by generative AI
Sun, Yuxuan and Li, Xue (2025). Automatic summarization of life-critical situations by generative AI. 20th International Conference, ADMA 2024, Sydney, NSW, Australia, 3 - 5 December 2024. Heidelberg, Germany: Springer. doi: 10.1007/978-981-96-0840-9_5
2025
Conference Publication
TinyMetaFed: efficient federated meta-learning for TinyML
Ren, Haoyu, Li, Xue, Anicic, Darko and Runkler, Thomas A. (2025). TinyMetaFed: efficient federated meta-learning for TinyML. International Workshops of ECML PKDD 2023, Turin, Italy, 18 - 22 September 2023. Cham, Switzerland: Springer Nature Switzerland. doi: 10.1007/978-3-031-74640-6_10
2024
Journal Article
Clinical and radiographic parameters for early periodontitis diagnosis: a comparative study
Fidyawati, Desy, Masulili, Sri Lelyati C., Iskandar, Hanna Bachtiar, Suhartanto, Heru, Kiswanjaya, Bramma and Li, Xue (2024). Clinical and radiographic parameters for early periodontitis diagnosis: a comparative study. Dentistry Journal, 12 (12) 407, 1-12. doi: 10.3390/dj12120407
2024
Conference Publication
Evidence sentence augmented sequence-to-sequence method for document-level relation extraction
Dai, Qizhu, Zhong, Jiang, Li, Kuan, Li, Rongzhen, Wang, Chen, Yang, Xuejiao, Yang, Sen and Li, Xue (2024). Evidence sentence augmented sequence-to-sequence method for document-level relation extraction. 2024 IEEE International Conference on Bioinformatics and Biomedicine (BIBM), Lisbon, Portugal, 3-6 December 2024. Piscataway, NJ, United States: Institute of Electrical and Electronics Engineers. doi: 10.1109/bibm62325.2024.10822111
2024
Conference Publication
Contrastive learning enhanced graph relation representation for document-level relation extraction
Dai, Qizhu, Zhong, Jiang, Li, Kuan, Li, Rongzhen, Wang, Chen, Lv, Lebin, Chen, Wenjun and Li, Xue (2024). Contrastive learning enhanced graph relation representation for document-level relation extraction. 2024 IEEE International Conference on Bioinformatics and Biomedicine (BIBM), Lisbon, Portugal, 3-6 December 2024. Piscataway, NJ, United States: Institute of Electrical and Electronics Engineers. doi: 10.1109/bibm62325.2024.10822629
2024
Journal Article
Investigation of age-hardening behaviour of Al alloys via feature screening-assisted machine learning
Hu, Mingwei, Tan, Qiyang, Knibbe, Ruth, Jiang, Bin, Li, Xue and Zhang, Ming-Xing (2024). Investigation of age-hardening behaviour of Al alloys via feature screening-assisted machine learning. Materials Science and Engineering: A, 916 147381, 1-12. doi: 10.1016/j.msea.2024.147381
2024
Journal Article
Self-supervised commonsense knowledge learning for document-level relation extraction
Li, Rongzhen, Zhong, Jiang, Xue, Zhongxuan, Dai, Qizhu and Li, Xue (2024). Self-supervised commonsense knowledge learning for document-level relation extraction. Expert Systems with Applications, 250 123921. doi: 10.1016/j.eswa.2024.123921
2024
Journal Article
Designing unique and high-performance Al alloys via machine learning: mitigating data bias through active learning
Hu, Mingwei, Tan, Qiyang, Knibbe, Ruth, Xu, Miao, Liang, Guofang, Zhou, Jianxin, Xu, Jun, Jiang, Bin, Li, Xue, Ramajayam, Mahendra, Dorin, Thomas and Zhang, Ming-Xing (2024). Designing unique and high-performance Al alloys via machine learning: mitigating data bias through active learning. Computational Materials Science, 244 113204, 113204. doi: 10.1016/j.commatsci.2024.113204
2024
Conference Publication
Enhancing NER with Sentence-Level Entity Detection as an Simple Auxiliary Task
Wang, Chen, Hu, Cong, Zhong, Jiang, Liu, Huawen, Li, Qi, Yu, Donghua and Li, Xue (2024). Enhancing NER with Sentence-Level Entity Detection as an Simple Auxiliary Task. 8th International Joint Conference, APWeb-WAIM 2024, Jinhua, China, 30 August-1September 2024. Heidelberg, Germany: Springer. doi: 10.1007/978-981-97-7232-2_2
2024
Journal Article
Multi-level alignments for compressed video super-resolution
Wei, Liu, Ye, Mao, Ji, Luping, Gan, Yan, Li, Shuai and Li, Xue (2024). Multi-level alignments for compressed video super-resolution. IEEE Transactions on Consumer Electronics, 70 (3), 5101-5114. doi: 10.1109/tce.2024.3411144
2024
Conference Publication
CaseLink: inductive graph learning for legal case retrieval
Tang, Yanran, Qiu, Ruihong, Yin, Hongzhi, Li, Xue and Huang, Zi (2024). CaseLink: inductive graph learning for legal case retrieval. 47th International ACM SIGIR Conference on Research and Development in Information Retrieval, Washington, DC, United States, 14-18 July 2024. New York, NY, United States: ACM. doi: 10.1145/3626772.3657693
2024
Journal Article
Disentangled relational graph neural network with contrastive learning for knowledge graph completion
Yin, Hong, Zhong, Jiang, Li, Rongzhen and Li, Xue (2024). Disentangled relational graph neural network with contrastive learning for knowledge graph completion. Knowledge-Based Systems, 295 111828. doi: 10.1016/j.knosys.2024.111828
2024
Journal Article
On-device online learning and semantic management of TinyML systems
Ren, Haoyu, Anicic, Darko, Li, Xue and Runkler, Thomas (2024). On-device online learning and semantic management of TinyML systems. ACM Transactions on Embedded Computing Systems, 23 (4) 55, 1-32. doi: 10.1145/3665278
2024
Journal Article
Stable viewport-based unsupervised compressed 360° video quality enhancement
Zou, Zizhuang, Ye, Mao, Li, Xue, Ji, Luping and Zhu, Ce (2024). Stable viewport-based unsupervised compressed 360° video quality enhancement. IEEE Transactions on Broadcasting, 70 (2), 607-619. doi: 10.1109/tbc.2024.3380435
2024
Conference Publication
Privacy-preserving and fairness-aware federated learning for critical infrastructure protection and resilience
Zhang, Yanjun, Sun, Ruoxi, Shen, Liyue, Bai, Guangdong, Xue, Minhui, Meng, Mark Huasong, Li, Xue, Ko, Ryan and Nepal, Surya (2024). Privacy-preserving and fairness-aware federated learning for critical infrastructure protection and resilience. WWW '24: ACM Web Conference 2024, Singapore, Singapore, 13-17 May 2024. New York, NY, United States: ACM. doi: 10.1145/3589334.3645545
2024
Journal Article
Spatial-temporal adaptive compressed screen content video quality enhancement
Shu, Chen, Ye, Mao, Guo, Hongwei and Li, Xue (2024). Spatial-temporal adaptive compressed screen content video quality enhancement. IEEE Transactions on Circuits and Systems II: Express Briefs, 71 (5), 2884-2888. doi: 10.1109/tcsii.2024.3350772
2024
Journal Article
Integrating crack causal augmentation framework and dynamic binary threshold for imbalanced crack instance segmentation
Lei, Qin, Zhong, Jiang, Wang, Chen and Li, Xue (2024). Integrating crack causal augmentation framework and dynamic binary threshold for imbalanced crack instance segmentation. Expert Systems with Applications, 240 122552. doi: 10.1016/j.eswa.2023.122552
Funding
Current funding
Past funding
Supervision
Availability
- Professor Xue Li is:
- Not available for supervision
Supervision history
Current supervision
-
Doctor Philosophy
Open-domain Dialogue Generation
Principal Advisor
Other advisors: Professor Helen Huang
-
Doctor Philosophy
Short Sequence Representation Learning with Limited Supervision
Principal Advisor
Other advisors: Associate Professor Sen Wang
-
Doctor Philosophy
Distribution-aware Automatic Summary Generalisation from Multi-modal Medical Data
Principal Advisor
-
Doctor Philosophy
Context-aware Representation Learning for Code Analysis
Principal Advisor
-
Doctor Philosophy
Entity Alignment for Evolving Temporal Knowledge Graphs
Principal Advisor
-
Doctor Philosophy
Weighted Ensembles for Different machine learning model that support non-data-sharing / vertical partition
Principal Advisor
Other advisors: Dr Priyanka Singh
-
Doctor Philosophy
Information Extraction from Large-scale Low-quality Data
Principal Advisor
-
Doctor Philosophy
Federated transfer learning on clinical multimodal data
Principal Advisor
-
Master Philosophy
Multi-modal Detection of Video Disinformation
Associate Advisor
Other advisors: Dr Priyanka Singh
-
Doctor Philosophy
Short Sequence Representation Learning with Limited Supervision
Associate Advisor
Other advisors: Associate Professor Jiajun Liu, Associate Professor Sen Wang
-
Doctor Philosophy
Graph Mining for Legal Case Retrieval
Associate Advisor
Other advisors: Professor Helen Huang
-
Doctor Philosophy
Privacy preservation with Large Language Models
Associate Advisor
Other advisors: Dr Priyanka Singh
-
Doctor Philosophy
Data Mining on Many-to-Many Complex Relationships
Associate Advisor
Other advisors: Professor Guido Zuccon
-
Doctor Philosophy
Data Mining on Many-to-Many Complex Relationships
Associate Advisor
Other advisors: Professor Guido Zuccon
Completed supervision
-
2021
Doctor Philosophy
Privacy-preserving Sharing for Genome-wide Analysis
Principal Advisor
Other advisors: Professor Ryan Ko, Dr Caitlin Curtis, Associate Professor Guangdong Bai
-
-
2020
Master Philosophy
Suicidal Ideation Detection in Online Social Content
Principal Advisor
Other advisors: Professor Helen Huang
-
-
2019
Doctor Philosophy
Deep learning on graphs - applications to brain network connectivity
Principal Advisor
-
-
2019
Doctor Philosophy
Constraint-based Recommendations of Query Visualization in Big Data
Principal Advisor
-
2018
Doctor Philosophy
Enhanced Learning for Smart Sensing in Environment Monitoring
Principal Advisor
-
-
-
-
-
-
-
2013
Doctor Philosophy
Data Linkage for Querying Heterogeneous Databases
Principal Advisor
Other advisors: Dr Ian Wood
-
-
-
-
2010
Master Philosophy
Maintaining Global Consistency in Advanced Database Systems
Principal Advisor
-
2010
Doctor Philosophy
Efficient grid computing based algorithms for power system data analysis
Principal Advisor
-
-
2007
Doctor Philosophy
PRIVACY-PRESERVING DATA MINING OF CLASSIFICATION RULES
Principal Advisor
-
2006
Doctor Philosophy
ON INTERACTIVE DOCUMENT CLASSIFICATION
Principal Advisor
-
2005
Doctor Philosophy
IMPROVEMENTS ON MULTIMEDIA SECURITY ALGORITHMS
Principal Advisor
-
2005
Doctor Philosophy
Knowledge discovery in a long temporal event sequence.
Principal Advisor
-
2022
Doctor Philosophy
On Encoding Causality for Natural Language Understanding
Associate Advisor
Other advisors: Associate Professor Mahsa Baktashmotlagh
-
2022
Doctor Philosophy
Electronic Health Record Representation for Similarity Computing
Associate Advisor
Other advisors: Professor Guido Zuccon
-
2020
Doctor Philosophy
Minimal interaction Information Retrieval: A theoretical framework with applications in clinical decision support
Associate Advisor
Other advisors: Professor Guido Zuccon
-
2020
Doctor Philosophy
Sequence Modelling for E-Commerce
Associate Advisor
Other advisors: Professor Hongzhi Yin
-
2020
Doctor Philosophy
Graph Representation Learning with Attribute Information
Associate Advisor
Other advisors: Professor Hongzhi Yin
-
2015
Doctor Philosophy
A Framework for Socialisation of Work Practice for Improving Business Process Performance
Associate Advisor
Other advisors: Professor Shazia Sadiq
-
2014
Doctor Philosophy
Effective Hashing for Searching Large-scale Multimedia Databases
Associate Advisor
-
2013
Doctor Philosophy
Supporting real-time query processing for advanced spatial database applications
Associate Advisor
-
2013
Doctor Philosophy
A Framework for Investigating, Analysing and Recommending eLearning Experience
Associate Advisor
Other advisors: Professor Shazia Sadiq
-
2012
Doctor Philosophy
Customizing activity behaviour for flexible business process execution
Associate Advisor
Other advisors: Professor Shazia Sadiq
-
2010
Doctor Philosophy
Accent classification from speech samples by use of machine learning
Associate Advisor
-
2007
Doctor Philosophy
A Data Partitioning Approach to Frequent Pattern Mining
Associate Advisor
-
2007
Doctor Philosophy
Electricity Market Management and Analysis using Advanced Data Mining and Statistical Methods
Associate Advisor
-
2006
Master Philosophy
DATA INTENSIVE MEDIATOR-BASED WEB SERVICES COMPOSITION WITH SELF-TUNING HISTOGRAM
Associate Advisor
Media
Enquiries
For media enquiries about Professor Xue Li's areas of expertise, story ideas and help finding experts, contact our Media team: