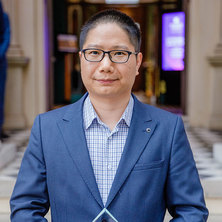
Overview
Background
Prof. Hongzhi Yin works as an ARC Future Fellow and Professor and director of the Responsible Big Data Intelligence Lab (RBDI) at The University of Queensland, Australia. He has made notable contributions to predictive analytics, recommendation systems, graph learning, social media analytics, and decentralized and edge intelligence. He has received numerous awards and recognition for his research achievements. He has been named to IEEE Computer Society’s AI’s 10 to Watch 2022 and Field Leader of Data Mining & Analysis in The Australian's Research 2020 magazine. In addition, he has received the prestigious 2023 Young Tall Poppy Science Awards, Australian Research Council Future Fellowship 2021, the Discovery Early Career Researcher Award 2016, UQ Foundation Research Excellence Award 2019, Rising Star of Science Award (2022-2024) and 2024 Computer Science in Australia Leader Award, AI 2000 Most Influential Scholar Honorable Mention in Data Mining (2022-2024). His research has won 8 international and national Best Paper Awards, including Best Student Full Paper Award at CIKM 2024, Best Paper Award - Honorable Mention at WSDM 2023, Best Paper Award at ICDE 2019, Best Student Paper Award at DASFAA 2020, Best Paper Award Nomination at ICDM 2018, ACM Computing Reviews' 21 Annual Best of Computing Notable Books and Articles, Best Paper Award at ADC 2018 and 2016. His Ph.D. thesis won Peking University Outstanding Ph.D. Dissertation Award 2014 and CCF Outstanding Ph.D. Dissertation Award (Nomination) 2014. He has ten conference papers recognized as the Most Influential Papers in Paper Digest, including KDD 2021 and 2013, AAAI 2021, SIGIR 2022, WWW 2023 and 2021, CIKM 2021, 2019, 2016, and 2015. He has published over 300 papers with an H-index of 83 (20000+ citations), including 250+ CCF A/CORE A* and 80+ CCF B/CORE A, such as KDD, SIGIR, WWW, ACL, WSDM, SIGMOD, VLDB, ICDE, NeurIPS, AAAI, IJCAI, ACM Multimedia, ECCV, IEEE TKDE, TNNL, VLDB Journal, and ACM TOIS. He has been the leading author (first/co-first author or corresponding author) for 250+. He has been an SPC/PC member for many top conferences, such as AAAI, IJCAI, KDD, ICML, ICLR, NeurIPS, SIGIR, WWW, WSDM, VLDB, ICDE, ICDM, and CIKM. He has been serving as Associate Editor/Guest Editor/Editorial Board for Neural Networks (JCR Q1, CCF B, 中科院一区), Science China Information Sciences (JCR Q1, CCF A, 中科院一区), Data Science and Engineering (JCR Q1, 中科院一区), Journal of Computer Science and Technology (JCST, CCF B), Journal of Social Computing, ACM Transactions on Information Systems 2022-2023 (JCR Q1, CCF A, CORE A, 中科院一区), ACM Transactions on Intelligent Systems and Technology 2020-2021 (JCR Q1), Information Systems 2020-2021 (CORE A*), and World Wide Web 2020-2021 and 2017-2018 (CORE A, CCF B). Dr. Yin has also been attracting wide media coverage, such as The Australian, SBS Radio Interviews, UQ News, Sohu.com, Faculty News of EAIT, IEEE Computer Society, ACM Computing Reviews.
I am now looking for highly motivated Ph.D. students. The University of Queensland ranks in the top 50 as measured by the Performance Ranking of Scientific Papers for World Universities. The University also ranks 40 in the QS World University Rankings and 41 in the US News Best Global Universities Rankings. The University of Queensland is the best in Australia according to the Australian Financial Review (AFR), which has now ranked UQ in the #1 position for 2 consecutive years. Please find the following two PhD scholarships.
Latest News
-
[4 April 2025] We have four full research papers accepted by the top conference SIGIR 2025 (CORE A*, CCF A).
-
ID-Free Not Risk-Free: LLM-Powered Agents Unveil Risks in ID-Free Recommender Systems
-
Diversity-aware Dual-promotion Poisoning Attack on Sequential Recommendation
-
Towards Distribution Matching between Collaborative and Language Spaces for Generative Recommendation
-
STAR-Rec: Making Peace with Length Variance and Pattern Diversity in Sequential Recommendation
-
-
[2 April 2025] Congratulations to the three new doctors, Dr. Wei Yuan, Dr. Jing Long and Dr. Yuting Sun, who were awarded their PhD by The University of Queensland.
-
[10 March 2025] Our survey paper "A Survey on Point-of-Interest Recommendation: Models, Architectures, and Security " has been accepted by TKDE 2025 (CORE A*, CCF A).
-
[21 Feb 2025] Our joint foundation work "On the Trustworthiness of Generative Foundation Models– Guideline, Assessment, and Perspective" has been released on both arXiv and Hugging Face. This research is the result of a broad collaboration with leading universities and research institutions worldwide, including the University of Notre Dame, Massachusetts Institute of Technology, University of Waterloo, Carnegie Mellon University, University of Illinois Urbana-Champaign, Stanford University, University of California, Santa Barbara, IBM Research, Microsoft Research, The University of Queensland and more.
-
[20 Feb 2025] I have been recognized as a Highly Ranked Scholar - Prior 5 Years (top 0.05% of all scholars) and #15 in Data Mining on ScholarGPS.
-
[26 January 2025] Our survey paper "Graph Condensation: A Survey" has been accepted by TKDE 2025 (CORE A*, CCF A).
-
[20 January 2025] We have three full research papers and one demo paper accepted by the top conference WWW 2025 (CORE A*, CCF A).
-
Rethinking and Accelerating Graph Condensation: A Training-Free Approach with Class Partition
-
BiasNavi: LLM-Empowered Data Bias Management
-
-
[18 January 2025] We have two research papers accepted by AAAI 2025 (CCF A, CORE A*) for Oral Presentation.
-
[5 December 2024] Our tutorial "Graph Condensation: Foundations, Methods and Prospects" has been accepted for presentation at The Web Conference 2025.
-
[30 November 2024] I have been invited to serve as SPC for IJCAI 2025 and DASFAA 2025.
-
[29 November 2024] I was honored with The Faculty Higher Degree Research Supervision Excellence Award.
-
[19 November 2024] Congratulations to Dr. Liang Qu on being awarded his PhD degree by The University of Queensland.
-
[17 November 2024] Our research paper "Progressive Generalization Risk Reduction for Data-Efficient Causal Effect Estimation" was accepted by the top conference KDD 2025 (CCF A, CORE A*). Congratulations to Hechuan.
-
[24 October 2024] Our research paper "Physics-guided Active Sample Reweighting for Urban Flow Prediction" won the Best Student Full Paper Award at the top conference CIKM 2024. Congratulations to Wei!
-
[18 October 2024] We have published two survey papers in top-tier journals: ACM Computing Surveys and Science China Information Sciences. Additionally, we have recently released two new survey papers on arXiv.
-
[17 October 2024] We have two research papers "PUMA: Efficient Continual Graph Learning with Graph Condensation" and "Handling Low Homophily in Recommender Systems with Partitioned Graph Transformer" accepted by the top journal TKDE.
- [26 September 2024] We have one research paper "Distribution-Aware Data Expansion with Diffusion Models" accepted by NeurIPS 2024 (CCF A, CORE A*).
-
[23 September 2024] We have three journal papers recognized as ESI Hot and Highly Cited papers.
-
[10 September 2024] I have been recognized with the 2024 Rising Star of Science Award in Research.com and ranked #8 in Australia among Rising Stars for 2024.
-
[24 August 2024] Two of my PhD graduates have been awarded the competitive ARC DECRA Fellowship. Congratulations to Weiqing and Junliang.
-
[23 July 2024] Recently, we have released 3 comprehensive survey papers.
-
[2 July 2024] I have been invited to serve as area chair at KDD 2025.
-
[27 June 2024] Our ARC Linkage Project "Building an Aussie Information Recommendation System You Can Trust" has been granted and funded.
-
[16 June 2024] I have been invited to co-chair the User modeling, personalization and recommendation track at The Web Conference 2025.
-
[6 June 2024] Recently, we have released 2 comprehensive survey papers.
-
[23 May 2024] Our project Personalized On-Device Large Language Models was shortlisted as a finalist for the 2024 iAwards.
-
[22 May 2024] Our research paper "Adversarial Item Promotion on Visually-Aware Recommender Systems by Guided Diffusion" has been accepted by the top journal TOIS 2024 (CORE A and CCF A).
-
[17 May 2024] We have 4 full research research papers accepted by the prestigious conference KDD 2024 (CORE A*, CCF A).
Availability
- Professor Hongzhi Yin is:
- Available for supervision
Qualifications
- Postgraduate Diploma, Peking University
- Doctor of Philosophy, Peking University
Research interests
-
Recommender System and User Modeling
-
Graph Mining and Embedding
-
Decentralized and Federated Learning
-
Edge Machine Learning and Applications
-
Trustworthy Machine Learning and Applications
-
QA, Chatbot and Information Retrieval
-
Time Series and Sequence Mining and Prediction
-
Spatiotemporal Data Mining
-
Smart Healthcare
Research impacts
Prof. Yin is currently directing the Responsible Big Data Intelligence Lab (RBDI). RBDI Lab aims and strives to develop decentralized, on-device, and trustworthy (e.g., privacy-preserving, robust, explainable and fair) data mining and machine learning techniques with theoretical backbones to better discover actionable patterns and intelligence from large-scale, heterogeneous, networked, dynamic and sparse data. RBDI joins forces with other fields such as urban transportation, healthcare, agriculture, E-commerce and marketing to help solve societal, environmental and economic challenges facing humanity in pursuit of a sustainable future. His research has also attracted media coverage, such as The Australian, SBS, UQ News, Faculty News of EAIT, ACM Computing Reviews, 360 News.
Works
Search Professor Hongzhi Yin’s works on UQ eSpace
2021
Conference Publication
GDFM: Gene Vectors Embodied Deep Attentional Factorization Machines for Interaction prediction
Mansha, Sameen, Khalid, Tayyab, Kamiran, Faisal, Hussain, Masroor, Hussain, Syed Fawad and Yin, Hongzhi (2021). GDFM: Gene Vectors Embodied Deep Attentional Factorization Machines for Interaction prediction. CIKM '21: The 30th ACM International Conference on Information and Knowledge Management, Virtual, 1-5 November 2021. New York, NY USA: Association for Computing Machinery. doi: 10.1145/3459637.3482110
2021
Conference Publication
Self-supervised graph co-training for session-based recommendation
Cui, Lizhen, Shao, Yingxia, Yu, Junliang, Yin, Hongzhi and Xia, Xin (2021). Self-supervised graph co-training for session-based recommendation. CIKM '21: The 30th ACM International Conference on Information and Knowledge Management, Virtual, 1-5 November 2021. New York, NY USA: Association for Computing Machinery. doi: 10.1145/3459637.3482388
2021
Conference Publication
A knowledge-aware recommender with attention-enhanced dynamic convolutional network
Liu, Yi, Li, Bohan, Zang, Yalei, Li, Aoran and Yin, Hongzhi (2021). A knowledge-aware recommender with attention-enhanced dynamic convolutional network. CIKM '21: The 30th ACM International Conference on Information and Knowledge Management, Virtual, 1-5 November 2021. New York, NY USA: Association for Computing Machinery. doi: 10.1145/3459637.3482406
2021
Conference Publication
Lightweight self-attentive sequential recommendation
Li, Yang, Chen, Tong, Zhang, Peng-Fei and Yin, Hongzhi (2021). Lightweight self-attentive sequential recommendation. CIKM '21: The 30th ACM International Conference on Information and Knowledge Management, Virtual, 1-5 November 2021. New York, NY USA: Association for Computing Machinery. doi: 10.1145/3459637.3482448
2021
Conference Publication
Double-scale self-supervised hypergraph learning for group recommendation
Zhang, Junwei, Gao, Min, Yu, Junliang, Guo, Lei, Li, Jundong and Yin, Hongzhi (2021). Double-scale self-supervised hypergraph learning for group recommendation. CIKM '21: The 30th ACM International Conference on Information and Knowledge Management, Virtual, 1-5 November 2021. New York, NY USA: Association for Computing Machinery. doi: 10.1145/3459637.3482426
2021
Journal Article
Quaternion factorization machines: a lightweight solution to intricate feature interaction modeling
Chen, Tong, Yin, Hongzhi, Zhang, Xiangliang, Huang, Zi, Wang, Yang and Wang, Meng (2021). Quaternion factorization machines: a lightweight solution to intricate feature interaction modeling. IEEE Transactions on Neural Networks and Learning Systems, PP (99), 1-14. doi: 10.1109/TNNLS.2021.3118706
2021
Conference Publication
Joint-teaching: learning to refine knowledge for resource-constrained unsupervised cross-modal retrieval
Zhang, Peng-Fei, Duan, Jiasheng, Huang, Zi and Yin, Hongzhi (2021). Joint-teaching: learning to refine knowledge for resource-constrained unsupervised cross-modal retrieval. MM '21: ACM Multimedia Conference, Virtual, 20-24 October 2021. New York, NY USA: Association for Computing Machinery. doi: 10.1145/3474085.3475286
2021
Conference Publication
CausalRec: causal inference for visual debiasing in visually-aware recommendation
Qiu, Ruihong, Wang, Sen, Chen, Zhi, Yin, Hongzhi and Huang, Zi (2021). CausalRec: causal inference for visual debiasing in visually-aware recommendation. MM '21: ACM Multimedia Conference, Virtual, 20-24 October 2021. New York, NY USA: Association for Computing Machinery. doi: 10.1145/3474085.3475266
2021
Journal Article
Fast-adapting and privacy-preserving federated recommender system
Wang, Qinyong, Yin, Hongzhi, Chen, Tong, Yu, Junliang, Zhou, Alexander and Zhang, Xiangliang (2021). Fast-adapting and privacy-preserving federated recommender system. The VLDB Journal, 31 (5), 877-896. doi: 10.1007/s00778-021-00700-6
2021
Journal Article
A block-based generative model for attributed network embedding
Liu, Xueyan, Yang, Bo, Song, Wenzhuo, Musial, Katarzyna, Zuo, Wanli, Chen, Hongxu and Yin, Hongzhi (2021). A block-based generative model for attributed network embedding. World Wide Web, 24 (5), 1439-1464. doi: 10.1007/s11280-021-00918-y
2021
Conference Publication
Socially-aware self-supervised tri-training for recommendation
Yu, Junliang, Yin, Hongzhi, Gao, Min, Xia, Xin, Zhang, Xiangliang and Viet Hung, Nguyen Quoc (2021). Socially-aware self-supervised tri-training for recommendation. 27th ACM SIGKDD Conference on Knowledge Discovery & Data Mining, Virtual (Singapore), 14-18 August 2021. New York, NY, United States: ACM. doi: 10.1145/3447548.3467340
2021
Conference Publication
ImGAGN: imbalanced network embedding via generative adversarial graph networks
Qu, Liang, Zhu, Huaisheng, Zheng, Ruiqi, Shi, Yuhui and Yin, Hongzhi (2021). ImGAGN: imbalanced network embedding via generative adversarial graph networks. 27th ACM SIGKDD Conference on Knowledge Discovery & Data Mining, Virtual (Singapore), 14-18 August 2021. New York, NY, United States: ACM. doi: 10.1145/3447548.3467334
2021
Conference Publication
Learning elastic embeddings for customizing on-device recommenders
Chen, Tong, Yin, Hongzhi, Zheng, Yujia, Huang, Zi, Wang, Yang and Wang, Meng (2021). Learning elastic embeddings for customizing on-device recommenders. 27th ACM SIGKDD Conference on Knowledge Discovery & Data Mining, Virtual (Singapore), 14-18 August 2021. New York, NY, United States: ACM. doi: 10.1145/3447548.3467220
2021
Conference Publication
Pre-training graph neural networks for cold-start users and items representation
Hao, Bowen, Zhang, Jing, Yin, Hongzhi, Li, Cuiping and Chen, Hong (2021). Pre-training graph neural networks for cold-start users and items representation. WSDM '21: Proceedings of the 14th ACM International Conference on Web Search and Data Mining, Virtual Event, 8-12 March 2021. New York, NY USA: Association for Computing Machinery. doi: 10.1145/3437963.3441738
2021
Conference Publication
Temporal meta-path guided explainable recommendation
Chen, Hongxu, Li, Yicong, Sun, Xiangguo, Xu, Guandong and Yin, Hongzhi (2021). Temporal meta-path guided explainable recommendation. WSDM '21: Proceedings of the 14th ACM International Conference on Web Search and Data Mining, Virtual Event Israel, 8 - 12 March 2021. New York, NY, United States: Association for Computing Machinery. doi: 10.1145/3437963.3441762
2021
Conference Publication
Heterogeneous hypergraph embedding for graph classification
Sun, Xiangguo, Yin, Hongzhi, Liu, Bo, Chen, Hongxu, Cao, Jiuxin, Shao, Yingxia and Viet Hung, Nguyen Quoc (2021). Heterogeneous hypergraph embedding for graph classification. WSDM '21: Proceedings of the 14th ACM International Conference on Web Search and Data Mining, Virtual Event, 8-12 March 2021. New York, NY USA: Association for Computing Machinery. doi: 10.1145/3437963.3441835
2021
Conference Publication
Discovering collaborative signals for next POI recommendation with iterative Seq2Graph augmentation
Li, Yang, Chen, Tong, Luo, Yadan, Yin, Hongzhi and Huang, Zi (2021). Discovering collaborative signals for next POI recommendation with iterative Seq2Graph augmentation. Proceedings of the Thirtieth International Joint Conference on Artificial Intelligence, Montreal, QC Canada, 19 - 27 August 2021. Palo Alto, CA United States: A A A I Press. doi: 10.24963/ijcai.2021/206
2021
Conference Publication
DA-GCN: a domain-aware attentive graph convolution network for shared-account cross-domain sequential recommendation
Guo, Lei, Tang, Li, Chen, Tong, Zhu, Lei, Nguyen, Quoc Viet Hung and Yin, Hongzhi (2021). DA-GCN: a domain-aware attentive graph convolution network for shared-account cross-domain sequential recommendation. International Joint Conference on Artificial Intelligence, Montreal, Canada, 19-27 August 2021. San Francisco, CA, United States: International Joint Conferences on Artificial Intelligence Organization. doi: 10.24963/ijcai.2021/342
2021
Conference Publication
Where are we in embedding spaces?
Zhang, Sixiao, Chen, Hongxu, Ming, Xiao, Cui, Lizhen, Yin, Hongzhi and Xu, Guandong (2021). Where are we in embedding spaces?. KDD '21: 27th ACM SIGKDD Conference on Knowledge Discovery & Data Mining, Singapore, Singapore, 14 - 18 August 2021. New York, NY, United States: Association for Computing Machinery. doi: 10.1145/3447548.3467421
2021
Conference Publication
Enhancing domain-level and user-level adaptivity in diversified recommendation
Liang, Yile, Qian, Tieyun, Li, Qing and Yin, Hongzhi (2021). Enhancing domain-level and user-level adaptivity in diversified recommendation. SIGIR '21: Proceedings of the 44th International ACM SIGIR Conference on Research and Development in Information Retrieval, Virtual Event, 11-15 July 2021. New York, NY USA: Association for Computing Machinery. doi: 10.1145/3404835.3462957
Funding
Current funding
Past funding
Supervision
Availability
- Professor Hongzhi Yin is:
- Available for supervision
Before you email them, read our advice on how to contact a supervisor.
Available projects
-
Decentralised Collaborative Predictive Analytics on Personal Smart Devices
This project tackles the challenging problem of personalised predictive analytics with resource-constrained personal devices and massive-scale data. The knowledge to be generated concerns privacy, fairness, and resource efficiency in the era of Internet of Things. The expected outcomes include a collaborative learning paradigm for building personalised models on personal smart devices in open and fully decentralised settings. Privacy and model fairness are core tenets of the paradigm. Personalised predictive analytics is frontier research that will position Australia at the forefront of AI and give business the tools needed to deploy innovative business systems for market exploitation with a secure, equitable and competitive advantage.
This Earmarked Scholarship project is aligned with a recently awarded Category 1 research grant. It offers you the opportunity to work with leading researchers and contribute to large projects of national significance.
-
Building an Trustworthy Information Recommendation System
Build a trustworthy information recommender system by spearheading the design and development of cutting-edge LLM4Rec techniques, misinformation filters, and privacy protection mechanisms.
This Earmarked Scholarship project is aligned with a recently awarded Category 1 research grant. It offers you the opportunity to work with leading researchers and contribute to large projects of national significance.
Supervision history
Current supervision
-
Doctor Philosophy
Secure Cross-device Federated Recommender Systems
Principal Advisor
Other advisors: Dr Miao Xu
-
Doctor Philosophy
Deep Learning for Univariate Time Series Anomaly Detection in Industrial IoT
Principal Advisor
Other advisors: Dr Thomas Taimre, Dr Slava Vaisman
-
Doctor Philosophy
Decentralised Collaborative Predictive Analytics on Personal Smart Devices
Principal Advisor
Other advisors: Dr Rocky Chen
-
Doctor Philosophy
Decentralised Collaborative Predictive Analytics on Personal Smart Devices
Principal Advisor
Other advisors: Dr Rocky Chen
-
Doctor Philosophy
Deep Learning for Univariate Time Series Anomaly Detection in Industrial IoT
Principal Advisor
Other advisors: Dr Thomas Taimre, Dr Slava Vaisman
-
Doctor Philosophy
Joint Feature Learning for Recommender System
Principal Advisor
Other advisors: Dr Rocky Chen
-
Doctor Philosophy
Secure Cross-device Federated Recommender Systems
Principal Advisor
Other advisors: Dr Miao Xu
-
Doctor Philosophy
Deep Learning for Graph Data Analysis
Principal Advisor
Other advisors: Dr Rocky Chen
-
Doctor Philosophy
Decentralized Learning for On-device Recommendation
Principal Advisor
Other advisors: Dr Rocky Chen
-
-
Doctor Philosophy
Knowledge Graph-based Conversational Recommender Systems
Principal Advisor
Other advisors: Dr Miao Xu
-
Doctor Philosophy
Secure Cross-device Federated Recommender Systems
Principal Advisor
Other advisors: Dr Miao Xu
-
Doctor Philosophy
Federated Graph Neural Network-based Recommender Systems
Principal Advisor
Other advisors: Dr Miao Xu
-
Doctor Philosophy
Decentralized Learning for On-device Recommendation
Principal Advisor
Other advisors: Dr Rocky Chen
-
Doctor Philosophy
Decentralised Collaborative Predictive Analytics on Personal Smart Devices
Principal Advisor
Other advisors: Dr Rocky Chen
-
Doctor Philosophy
Image Generation from Texts
Principal Advisor
Other advisors: Dr Thomas Taimre, Dr Slava Vaisman
-
Doctor Philosophy
Decentralized Point-Of-Interest (POI) Recommender Systems
Principal Advisor
Other advisors: Dr Rocky Chen
-
Doctor Philosophy
Meeting Challenges on Secure Recommender Systems
Principal Advisor
Other advisors: Dr Rocky Chen
-
Doctor Philosophy
Decentralized Point-Of-Interest (POI) Recommender Systems
Principal Advisor
Other advisors: Dr Rocky Chen
-
Doctor Philosophy
Understanding and mitigating greenhouse gas emissions from wastewater system in the data era
Associate Advisor
Other advisors: Dr Haoran Duan, Professor Liu Ye
-
Doctor Philosophy
Scalable and Lightweight On-Device Recommender Systems
Associate Advisor
Other advisors: Dr Rocky Chen
-
Doctor Philosophy
Understanding nitrous oxide emissions from wastewater treatment processes with stable isotopes and mathematical modelling
Associate Advisor
Other advisors: Dr Haoran Duan, Professor Liu Ye
-
Doctor Philosophy
Understanding and mitigating greenhouse gas emissions from wastewater system in the data era
Associate Advisor
Other advisors: Dr Haoran Duan, Professor Liu Ye
-
Doctor Philosophy
Scalable and Lightweight On-Device Recommender Systems
Associate Advisor
Other advisors: Dr Rocky Chen, Dr Junliang Yu
-
Doctor Philosophy
Scalable and Generalizable Graph Neural Networks
Associate Advisor
Other advisors: Dr Rocky Chen
-
Doctor Philosophy
Sustainable On-Device Recommender Systems
Associate Advisor
Other advisors: Dr Rocky Chen
-
Doctor Philosophy
Lightweight Graph Neural Networks for Recommendation
Associate Advisor
Other advisors: Dr Rocky Chen
-
Doctor Philosophy
Causal Analysis for Decision Support in Public Health
Associate Advisor
Other advisors: Professor Shazia Sadiq, Dr Rocky Chen
-
Doctor Philosophy
Integrated high-throughput material synthesis and characterisation system
Associate Advisor
Other advisors: Associate Professor Jingwei Hou
Completed supervision
-
2025
Doctor Philosophy
Deep Learning for Univariate Time Series Anomaly Detection in Industrial IoT
Principal Advisor
Other advisors: Dr Thomas Taimre, Dr Slava Vaisman
-
2025
Doctor Philosophy
Decentralized Point-Of-Interest (POI) Recommender Systems
Principal Advisor
Other advisors: Dr Rocky Chen
-
2025
Doctor Philosophy
Secure Cross-device Federated Recommender Systems
Principal Advisor
Other advisors: Dr Miao Xu
-
2024
Doctor Philosophy
Federated Graph Neural Network-based Recommender Systems
Principal Advisor
Other advisors: Dr Miao Xu
-
2023
Doctor Philosophy
From Cloud to Device: Transforming Recommender Systems for On-Device Deployment
Principal Advisor
Other advisors: Dr Miao Xu
-
2023
Doctor Philosophy
Decentralized On-device Machine Learning and Unlearning for IoT Collaboration
Principal Advisor
Other advisors: Dr Miao Xu
-
2023
Doctor Philosophy
Enhancing Recommender Systems wtih Self-Supervised Learning
Principal Advisor
Other advisors: Professor Helen Huang
-
2022
Doctor Philosophy
Toward Deep Conversational Recommender Systems
Principal Advisor
Other advisors: Professor Helen Huang
-
2022
Doctor Philosophy
Secure Recommender Systems
Principal Advisor
Other advisors: Professor Helen Huang
-
2022
Doctor Philosophy
Decentralized Framework for Embedding Large-scale Networks
Principal Advisor
Other advisors: Professor Helen Huang
-
2021
Doctor Philosophy
Lightweight and Secure Deep Learning-based Mobile Recommender Systems
Principal Advisor
Other advisors: Professor Helen Huang
-
2020
Doctor Philosophy
Sequence Modelling for E-Commerce
Principal Advisor
Other advisors: Professor Xue Li
-
2020
Doctor Philosophy
Graph Representation Learning with Attribute Information
Principal Advisor
Other advisors: Professor Xue Li
-
2020
Master Philosophy
Advanced Machine Learning Algorithms for Discrete Datasets
Principal Advisor
Other advisors: Professor Shazia Sadiq
-
2017
Doctor Philosophy
POINT OF INTERESTS RECOMMENDATION IN LOCATION-BASED SOCIAL NETWORKS
Principal Advisor
Other advisors: Professor Shazia Sadiq
-
2025
Doctor Philosophy
Understanding and mitigating greenhouse gas emissions from wastewater system in the data era
Associate Advisor
Other advisors: Dr Haoran Duan, Professor Liu Ye
-
2023
Doctor Philosophy
Multi-modal Data Modeling with Awareness of Efficiency, Reliability, and Privacy
Associate Advisor
Other advisors: Professor Helen Huang
-
2022
Doctor Philosophy
Neural Attentive Recommender Systems
Associate Advisor
Other advisors: Professor Helen Huang, Dr Rocky Chen
-
2022
Master Philosophy
An exploration into the correlation between users' intentions and candidates for query- and non-query-based retrieval
Associate Advisor
Other advisors: Professor Helen Huang
-
2021
Doctor Philosophy
Towards Efficient Similarity Search with Semantic Hashing Techniques
Associate Advisor
Other advisors: Professor Helen Huang
-
2021
Doctor Philosophy
Multimedia Content Analytics with Modality Transition
Associate Advisor
Other advisors: Professor Helen Huang
-
2018
Doctor Philosophy
Understand Video Event by Exploiting Semantic and Temporal Information for Classification and Retrieval
Associate Advisor
Other advisors: Professor Helen Huang
-
Doctor Philosophy
Modelling Sequential Patterns of User Behaviour in Recommender Systems
Associate Advisor
Other advisors: Professor Helen Huang
Media
Enquiries
For media enquiries about Professor Hongzhi Yin's areas of expertise, story ideas and help finding experts, contact our Media team: