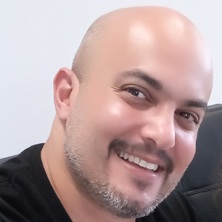
Overview
Availability
- Professor Fred Roosta is:
- Available for supervision
Fields of research
Qualifications
- Doctor of Philosophy, The University of British Columbia
Research interests
-
Artificial Intelligence
-
Machine Learning
-
Numerical Optimization
-
Numerical Analysis
-
Computational Statistics
-
Scientific Computing
Works
Search Professor Fred Roosta’s works on UQ eSpace
2024
Journal Article
Non-uniform smoothness for gradient descent
Berahas, Albert S., Roberts, Lindon and Roosta, Fred (2024). Non-uniform smoothness for gradient descent. Transactions on Machine Learning Research.
2024
Conference Publication
Manifold integrated gradients: Riemannian geometry for feature attribution
Zaher, Eslam, Trzaskowski, Maciej, Nguyen, Quan and Roosta, Fred (2024). Manifold integrated gradients: Riemannian geometry for feature attribution. International Conference on Machine Learning, Vienna, Austria, 21-27 July 2024. Proceedings of Machine Learning Research.
2024
Conference Publication
Inexact Newton-type methods for optimisation with nonnegativity constraints
Smee, Oscar and Roosta, Fred (2024). Inexact Newton-type methods for optimisation with nonnegativity constraints. International Conference on Machine Learning, Vienna, Austria, 21-27 July 2024. Proceedings of Machine Learning Research.
2023
Conference Publication
Monotonicity and double descent in uncertainty estimation with gaussian processes
Hodgkinson, Liam, Van Der Heide, Chris, Roosta, Fred and Mahoney, Michael W. (2023). Monotonicity and double descent in uncertainty estimation with gaussian processes. International Conference on Machine Learning, Honolulu, HI United States, 23 - 29 July 2023. San Diego, CA United States: International Conference on Machine Learning.
2023
Journal Article
Generalising uncertainty improves accuracy and safety of deep learning analytics applied to oncology
MacDonald, Samual, Foley, Helena, Yap, Melvyn, Johnston, Rebecca L., Steven, Kaiah, Koufariotis, Lambros T., Sharma, Sowmya, Wood, Scott, Addala, Venkateswar, Pearson, John V., Roosta, Fred, Waddell, Nicola, Kondrashova, Olga and Trzaskowski, Maciej (2023). Generalising uncertainty improves accuracy and safety of deep learning analytics applied to oncology. Scientific Reports, 13 (1) 7395, 1-14. doi: 10.1038/s41598-023-31126-5
2023
Journal Article
Inexact Newton-CG algorithms with complexity guarantees
Yao, Zhewei, Xu, Peng, Roosta, Fred, Wright, Stephen J. and Mahoney, Michael W. (2023). Inexact Newton-CG algorithms with complexity guarantees. IMA Journal of Numerical Analysis, 43 (3), 1855-1897. doi: 10.1093/imanum/drac043
2022
Journal Article
MINRES: From negative curvature detection to monotonicity properties
Liu, Yang and Roosta, Fred (2022). MINRES: From negative curvature detection to monotonicity properties. SIAM Journal on Optimization, 32 (4), 2636-2661. doi: 10.1137/21m143666x
2022
Journal Article
Confirming the Lassonde Curve through life cycle analysis and its effect on share price: A case study of three ASX listed gold companies
Rijsdijk, Timothy, Nehring, Micah, Kizil, Mehmet and Roosta, Fred (2022). Confirming the Lassonde Curve through life cycle analysis and its effect on share price: A case study of three ASX listed gold companies. Resources Policy, 77 102704, 1-12. doi: 10.1016/j.resourpol.2022.102704
2022
Journal Article
Newton-MR: inexact Newton Method with minimum residual sub-problem solver
Roosta, Fred, Liu, Yang, Xu, Peng and Mahoney, Michael W. (2022). Newton-MR: inexact Newton Method with minimum residual sub-problem solver. EURO Journal on Computational Optimization, 10 100035, 1-44. doi: 10.1016/j.ejco.2022.100035
2022
Conference Publication
Crop type prediction utilising a long short-term memory with a self-attention for winter crops in Australia
Nguyen, Dung, Zhao, Yan, Zhang, Yifan, Huynh, Anh Ngoc-Lan, Roosta, Fred, Hammer, Graeme, Chapman, Scott and Potgieter, Andries (2022). Crop type prediction utilising a long short-term memory with a self-attention for winter crops in Australia. IGARSS 2022 - 2022 IEEE International Geoscience and Remote Sensing Symposium, Kuala Lumpur, Malaysia, 17-22 July 2022. Piscataway, NJ, United States: Institute of Electrical and Electronics Engineers. doi: 10.1109/IGARSS46834.2022.9883737
2022
Journal Article
LSAR: efficient leverage score sampling algorithm for the analysis of big time series data
Eshragh, Ali, Roosta, Fred, Nazari, Asef and Mahoney, Michael W. (2022). LSAR: efficient leverage score sampling algorithm for the analysis of big time series data. Journal of Machine Learning Research, 23, 1-36.
2021
Journal Article
Implicit Langevin algorithms for sampling from log-concave densities
Hodgkinson, Liam, Salomone, Robert and Roosta, Fred (2021). Implicit Langevin algorithms for sampling from log-concave densities. Journal of Machine Learning Research, 22 136, 1-30.
2021
Conference Publication
Shadow Manifold Hamiltonian Monte Carlo
van der Heide, Chris, Hodgkinson, Liam, Roosta, Fred and Kroese, Dirk (2021). Shadow Manifold Hamiltonian Monte Carlo. International Conference on Artificial Intelligence and Statistics, Online, 27-30- July 2021. Tempe, AZ United States: ML Research Press.
2021
Journal Article
Evolution and application of digital technologies to predict crop type and crop phenology in agriculture
Potgieter, A. B., Zhao, Yan, Zarco-Tejada, Pablo J, Chenu, Karine, Zhang, Yifan, Porker, Kenton, Biddulph, Ben, Dang, Yash P., Neale, Tim, Roosta, Fred and Chapman, Scott (2021). Evolution and application of digital technologies to predict crop type and crop phenology in agriculture. In Silico Plants, 3 (1) diab017, 1-23. doi: 10.1093/insilicoplants/diab017
2021
Journal Article
Inexact nonconvex Newton-type methods
Yao, Zhewei, Xu, Peng, Roosta, Fred and Mahoney, Michael W. (2021). Inexact nonconvex Newton-type methods. INFORMS Journal on Optimization, 3 (2), 154-182. doi: 10.1287/ijoo.2019.0043
2021
Journal Article
Convergence of Newton-mr under inexact hessian information
Liu, Yang and Roosta, Fred (2021). Convergence of Newton-mr under inexact hessian information. SIAM Journal on Optimization, 31 (1), 59-90. doi: 10.1137/19M1302211
2021
Conference Publication
Avoiding kernel fixed points: Computing with ELU and GELU infinite networks
Tsuchida, Russell, Pearce, Tim, van der Heide, Chris, Roosta, Fred and Gallagher, Marcus (2021). Avoiding kernel fixed points: Computing with ELU and GELU infinite networks. 35th AAAI Conference on Artificial Intelligence, AAAI 2021, Online, 2 - 9 February 2021. Menlo Park, CA United States: Association for the Advancement of Artificial Intelligence. doi: 10.1609/aaai.v35i11.17197
2021
Journal Article
Limit theorems for out-of-sample extensions of the adjacency and Laplacian spectral embeddings
Levin, Keith D., Roosta, Fred, Tang, Minh, Mahoney, Michael W. and Priebe, Carey E. (2021). Limit theorems for out-of-sample extensions of the adjacency and Laplacian spectral embeddings. Journal of Machine Learning Research, 22 194, 1-59.
2021
Conference Publication
Stochastic continuous normalizing flows: training SDEs as ODEs
Hodgkinson, Liam, van der Heide, Chris, Roosta, Fred and Mahoney, Michael W. (2021). Stochastic continuous normalizing flows: training SDEs as ODEs. Conference on Uncertainty in Artificial Intelligence, Online, 27-29 July 2021. San Diego, CA, United States: Association For Uncertainty in Artificial Intelligence (AUAI).
2021
Conference Publication
Non-PSD matrix sketching with applications to regression and optimization
Feng, Zhili, Roosta, Fred and Woodruff, David P. (2021). Non-PSD matrix sketching with applications to regression and optimization. Conference on Uncertainty in Artificial Intelligence, Online, 27-29 July 2021. San Diego, CA United States: Association For Uncertainty in Artificial Intelligence (AUAI).
Supervision
Availability
- Professor Fred Roosta is:
- Available for supervision
Before you email them, read our advice on how to contact a supervisor.
Available projects
-
Non-convex Optimization for Machine Learning
Design, analysis, and implementation of novel optimization algorithms for optimization of modern non-convex machine learning problems.
-
Interpretable AI - Theory and Practice
This project will extend and innovate, both theoretically and practically, interpretable methods in AI that are transparent and explainable to improve trust and usability. It will also explore novel approaches for uncertainty quantification and understanding causality.
-
Exploring Predictivity--Parsimony Trade-off In Scientific Machine Learning
This project will investigate, both theoretically and empirically, novel statistical techniques to explore the trade-offs between high-generalization performance and low-model complexity for scientific machine learning.
-
Novel Machine Learning Models for Scientific Discovery
To extend the application range of machine learning to scientific domains, this project will design, analyze and implement novel machine learning techniques that learn from data, while conform with known properties of the underlying scientific models.
-
Automated Discovery of Optimization and Linear Algebra Algorithms
Using reinforcement learning to automate algorithmic discovery, this project aims to develop novel variants of first- and second-order optimization methods, randomized numerical linear algebra techniques, and mixed-integer programming approaches.
-
Second-order Optimization Algorithms for Machine Learning
This project aims to develop the next generation of second-order optimization methods for training complex machine learning models, with particular focus on constrained problems arising in scientific machine learning applications.
-
Distributed Optimization Algorithms for Large-scale Machine Learning
This project aims to design, analyze and implement efficient optimization algorithms suitable for distributed computing environments, with focus on large-scale machine learning.
Supervision history
Current supervision
-
Doctor Philosophy
Characterizing Influence and Sensitivity in the Interpolating Regime
Principal Advisor
Other advisors: Associate Professor Marcus Gallagher
-
Doctor Philosophy
AI/ML Framework for Mixed-integer Nonlinear Optimisation
Principal Advisor
-
Doctor Philosophy
Newton type methods for constrained optimization
Principal Advisor
-
Doctor Philosophy
Stochastic Simulation and Optimization Methods for Machine Learning
Principal Advisor
-
Doctor Philosophy
AI/ML Framework for Mixed-integer Nonlinear Optimisation
Principal Advisor
Other advisors: Dr Nan Ye
-
Doctor Philosophy
Interpretable AI-Theory and Practice
Principal Advisor
Other advisors: Dr Quan Nguyen
-
Doctor Philosophy
Novel Machine Learning Models for Scientific Discovery
Principal Advisor
-
Doctor Philosophy
Forecasting the Market Capitalisation of ASX Listed Junior Resource Companies through an Artificial Neural Network
Associate Advisor
Other advisors: Associate Professor Mehmet Kizil, Dr Micah Nehring
-
Doctor Philosophy
Offline Reinforcement Learning Theory and Algorithms
Associate Advisor
Other advisors: Dr Nan Ye
Completed supervision
-
2023
Doctor Philosophy
Newton-MR Methods for Non-convex Smooth Unconstrained Optimizations
Principal Advisor
Other advisors: Professor Dirk Kroese
-
2022
Doctor Philosophy
Efficient second-order optimisation methods for large scale machine learning
Principal Advisor
Other advisors: Associate Professor Marcus Gallagher
-
2022
Doctor Philosophy
Discounting-free Policy Gradient Reinforcement Learning from Transient States
Associate Advisor
Other advisors: Associate Professor Marcus Gallagher
-
2020
Doctor Philosophy
Results on Infinitely Wide Multi-layer Perceptrons
Associate Advisor
Other advisors: Associate Professor Marcus Gallagher
-
2018
Doctor Philosophy
Advances in Monte Carlo Methodology
Associate Advisor
Other advisors: Dr Slava Vaisman, Professor Dirk Kroese
Media
Enquiries
For media enquiries about Professor Fred Roosta's areas of expertise, story ideas and help finding experts, contact our Media team: