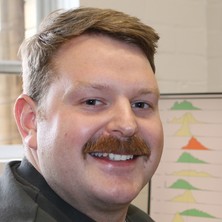
Overview
Background
My research interests centre on using quantitative genetics to drive genetic gain and efficiency in plant and animal breeding programmes.
Previous work in the UK focused on using genomic information prediction to demonstrate and exploit synergies between plant and animal breeding. Stochastic simulations were used to quantify the impact of new genomic breeding strategies in a wide variety of settings; from low to middle-income (LMIC) dairy cattle breeding programs to large, well-funded maize breeding programs.
My work at QAAFI and the ARC Centre of Excellence for Plant Success in Nature & Agriculture focuses on the development of prediction methods that combine biological, environmental and management information under a unifying framework, to enhance our ability to identify breeding parents, varieties and genotype-by-agronomic management (GxM) solutions that are best suited for future climates.
Availability
- Dr Owen Powell is:
- Available for supervision
- Media expert
Qualifications
- Bachelor (Honours) of Development Biology, University of Edinburgh
- Masters (Research) of Science, University of Edinburgh
- Doctor of Philosophy, University of Edinburgh
Research impacts
Dr Powell helps public and private genetic improvement programs to find better ways to predict the outcomes of selective breeding.
His core work focuses on developing, applying and optimising prediction methods to accelerate rates of sustainable genetic improvement.
Dr Powell is involved in the research and HDR student supervision on projects that span plant, animal and aquaculture species.
Works
Search Professor Owen Powell’s works on UQ eSpace
Featured
2025
Journal Article
Improved Genomic Prediction Performance with Ensembles of Diverse Models
Tomura, Shunichiro, Wilkinson, Melanie J., Cooper, Mark and Powell, Owen (2025). Improved Genomic Prediction Performance with Ensembles of Diverse Models. G3: Genes, Genomes, Genetics, 15 (5) jkaf048, 1-15. doi: 10.1093/g3journal/jkaf048
2024
Journal Article
Potential approaches to create ultimate genotypes in crops and livestock
Hayes, Ben J., Mahony, Timothy J., Villiers, Kira, Warburton, Christie, Kemper, Kathryn E., Dinglasan, Eric, Robinson, Hannah, Powell, Owen, Voss-Fels, Kai, Godwin, Ian D. and Hickey, Lee T. (2024). Potential approaches to create ultimate genotypes in crops and livestock. Nature Genetics, 56 (11), 2310-2317. doi: 10.1038/s41588-024-01942-0
Featured
2023
Journal Article
Extending the breeder’s equation to take aim at the target population of environments
Cooper, Mark, Powell, Owen, Gho Brito, Carla, Tang, Tom and Messina, Carlos (2023). Extending the breeder’s equation to take aim at the target population of environments. Frontiers in Plant Science, 14 1129591, 1-10. doi: 10.3389/fpls.2023.1129591
Featured
2022
Journal Article
Investigations into the emergent properties of gene-to-phenotype networks across cycles of selection: a case study of shoot branching in plants
Powell, Owen M., Barbier, Francois, Voss-Fels, Kai P., Beveridge, Christine and Cooper, Mark (2022). Investigations into the emergent properties of gene-to-phenotype networks across cycles of selection: a case study of shoot branching in plants. in silico Plants, 4 (1) diac006, 1-9. doi: 10.1093/insilicoplants/diac006
2024
Other Outputs
Improvements in prediction performance of ensemble approaches for genomic prediction in crop breeding
Tomura, Shunichiro, Cooper, Mark and Powell, Owen (2024). Improvements in prediction performance of ensemble approaches for genomic prediction in crop breeding. doi: 10.1101/2024.09.06.611589
2024
Conference Publication
Prediction of non-additive genetic effects with hierarchical genomic prediction models
Powell, Owen, McLean, Greg, Brider, Jason, Saddigh, Joe, Technow, Frank, Tang, Tom, Totir, Radu, Messina, Carlos D., Hammer, Graeme and Cooper, Mark (2024). Prediction of non-additive genetic effects with hierarchical genomic prediction models. International Conference of Quantitative Genetics (ICQG) 7, Vienna, Austria, 22-26 July 2024. doi: 10.6084/m9.figshare.26425735.v1
2024
Journal Article
Genomic prediction for sugarcane diseases including hybrid Bayesian-machine learning approaches
Chen, Chensong, Bhuiyan, Shamsul A., Ross, Elizabeth, Powell, Owen, Dinglasan, Eric, Wei, Xianming, Atkin, Felicity, Deomano, Emily and Hayes, Ben (2024). Genomic prediction for sugarcane diseases including hybrid Bayesian-machine learning approaches. Frontiers in Plant Science, 15 1398903, 1-13. doi: 10.3389/fpls.2024.1398903
2024
Journal Article
Adaptation and plasticity of yield in hybrid and inbred sorghum
Otwani, Daniel, Hunt, Colleen, Cruickshank, Alan, Powell, Owen, Koltunow, Anna, Mace, Emma and Jordan, David (2024). Adaptation and plasticity of yield in hybrid and inbred sorghum. Crop Science, 64 (2), 560-570. doi: 10.1002/csc2.21160
2023
Journal Article
Use of continuous genotypes for genomic prediction in sugarcane
Yadav, Seema, Ross, Elizabeth M., Wei, Xianming, Liu, Shouye, Nguyen, Loan To, Powell, Owen, Hickey, Lee T., Deomano, Emily, Atkin, Felicity, Voss‐Fels, Kai P. and Hayes, Ben J. (2023). Use of continuous genotypes for genomic prediction in sugarcane. The Plant Genome, 17 (1) e20417, e20417. doi: 10.1002/tpg2.20417
2023
Journal Article
Optimising clonal performance in sugarcane: leveraging non-additive effects via mate-allocation strategies
Yadav, Seema, Ross, Elizabeth M., Wei, Xianming, Powell, Owen, Hivert, Valentin, Hickey, Lee T., Atkin, Felicity, Deomano, Emily, Aitken, Karen S., Voss-Fels, Kai P. and Hayes, Ben J. (2023). Optimising clonal performance in sugarcane: leveraging non-additive effects via mate-allocation strategies. Frontiers in Plant Science, 14 1260517, 1260517. doi: 10.3389/fpls.2023.1260517
2023
Conference Publication
APSIM-WGP: a software platform to predict crop GxExM interactions
Powell, Owen, McLean, Greg, Brider, Jason, Hammer, Graeme and Cooper, Mark (2023). APSIM-WGP: a software platform to predict crop GxExM interactions. GxExM Symposium II, Gainesville, FL USA, 6-7 November 2023.
2023
Journal Article
Comparison of genomic prediction models for general combining ability in early stages of hybrid breeding programs
de Jong, Guilherme, Powell, Owen, Gorjanc, Gregor, Hickey, John M. and Gaynor, R. Chris (2023). Comparison of genomic prediction models for general combining ability in early stages of hybrid breeding programs. Crop Science, 63 (6), 3338-3355. doi: 10.1002/csc2.21105
2023
Journal Article
Genomic prediction with machine learning in sugarcane, a complex highly polyploid clonally propagated crop with substantial non‐additive variation for key traits
Chen, Chensong, Powell, Owen, Dinglasan, Eric, Ross, Elizabeth M., Yadav, Seema, Wei, Xianming, Atkin, Felicity, Deomano, Emily and Hayes, Ben J. (2023). Genomic prediction with machine learning in sugarcane, a complex highly polyploid clonally propagated crop with substantial non‐additive variation for key traits. The Plant Genome, 16 (4) e20390, 1-13. doi: 10.1002/tpg2.20390
2023
Journal Article
Advancing artificial intelligence to help feed the world
Hayes, Ben J., Chen, Chensong, Powell, Owen, Dinglasan, Eric, Villiers, Kira, Kemper, Kathryn E. and Hickey, Lee T. (2023). Advancing artificial intelligence to help feed the world. Nature Biotechnology, 41 (9), 1-2. doi: 10.1038/s41587-023-01898-2
2023
Conference Publication
Random Forest Importance Diagnostics can Capture Quantitative Genetic Properties of Markers for Genomic Prediction
Tomura, Shunichiro, Powell, Owen and Cooper, Mark (2023). Random Forest Importance Diagnostics can Capture Quantitative Genetic Properties of Markers for Genomic Prediction. International Congress of Genetics, Melbourne, VIC Australia, 16-21 July 2023. figShare. doi: 10.6084/m9.figshare.24211230.v1
2023
Other Outputs
Stochastic Simulation of Divergent Selection Experiment on a Gene-Phenotype Network: A Case Study of Shoot Branching in Plants
Powell, Owen and Cooper, Mark (2023). Stochastic Simulation of Divergent Selection Experiment on a Gene-Phenotype Network: A Case Study of Shoot Branching in Plants. figShare. (Dataset) doi: 10.6084/m9.figshare.23590083
2023
Conference Publication
GPU can Accelerate the Prediction of Complex Phenotypes
Tomura, Shunichiro, Powell, Owen and Cooper, Mark (2023). GPU can Accelerate the Prediction of Complex Phenotypes. Australasian Leadership Computing Symposium, Canberra, ACT Australia, 14-16 June 2023. doi: 10.6084/m9.figshare.24484831.v1
2023
Conference Publication
Hierarchical Gene-Phenotype Maps as a Framework to Predict GxExM Interactions
Powell, Owen, McLean, Greg, Brider, Jason, Technow, Frank, Tang, Tom, Messina, Carlos D., Hammer, Graeme and Cooper, Mark (2023). Hierarchical Gene-Phenotype Maps as a Framework to Predict GxExM Interactions. Quantitative Genetics and Genomics Gordon Research Conference, Ventura, CA, United States, 12-17 February 2023.
2023
Book Chapter
Predicting Genotype × Environment × Management (G × E × M) interactions for the design of crop improvement strategies: integrating breeder, agronomist, and farmer perspectives
Cooper, Mark, Messina, Carlos D., Tang, Tom, Gho, Carla, Powell, Owen M., Podlich, Dean W., Technow, Frank and Hammer, Graeme L. (2023). Predicting Genotype × Environment × Management (G × E × M) interactions for the design of crop improvement strategies: integrating breeder, agronomist, and farmer perspectives. Plant breeding reviews. (pp. 467-585) edited by Irwin Goldman. Hoboken, NJ, United States: Wiley Blackwell. doi: 10.1002/9781119874157.ch8
2022
Journal Article
Genomic mate-allocation strategies exploiting additive and non-additive genetic effects to maximise total clonal performance in sugarcane
Yadav, Seema, Ross, Elizabeth, Wei, Xianming, Powell, Owen, Hivert, Valentin, Hickey, Lee T., Atkin, Felicity, Deomano, Emily, Aitken, Karen S., Voss-Fels, Kai P. and Hayes, Ben J. (2022). Genomic mate-allocation strategies exploiting additive and non-additive genetic effects to maximise total clonal performance in sugarcane.
Funding
Past funding
Supervision
Availability
- Dr Owen Powell is:
- Available for supervision
Before you email them, read our advice on how to contact a supervisor.
Supervision history
Current supervision
-
Doctor Philosophy
Predicting Plant Success For Future Generations
Principal Advisor
Other advisors: Professor Mark Cooper
-
Doctor Philosophy
Assessment of machine learning methods to discover novel models of gene networks to improve genomic prediction for plant breeding
Associate Advisor
Other advisors: Dr James Lefevre, Dr Melanie Wilkinson, Professor Mark Cooper
-
Doctor Philosophy
Genomic selection for finfish Breeding Programs
Associate Advisor
Other advisors: Professor Ben Hayes
-
Doctor Philosophy
Experimental investigation in Arabidopsis thaliana of realised selection trajectories for complex branching and flowering traits under the control of gene networks following application of genomic prediction methods.
Associate Advisor
Other advisors: Professor Christine Beveridge, Professor Mark Cooper
-
Doctor Philosophy
New approaches to maximise gain and genetic diversity in plant breeding programs
Associate Advisor
Other advisors: Professor Ben Hayes
-
Doctor Philosophy
Machine learning strategies to accelerate genetic gain in agricultural species
Associate Advisor
Other advisors: Dr Eric Dinglasan, Dr Elizabeth Ross, Professor Ben Hayes
Completed supervision
-
2023
Doctor Philosophy
Optimising Genomic Selection for Sugarcane
Associate Advisor
Other advisors: Professor Lee Hickey, Dr Kai Voss-Fels, Dr Elizabeth Ross, Professor Ben Hayes
Media
Enquiries
Contact Dr Owen Powell directly for media enquiries about:
- Computational Biology
- Computer Simulations
- Data Science
- Genetics
- Plant Breeding
Need help?
For help with finding experts, story ideas and media enquiries, contact our Media team: