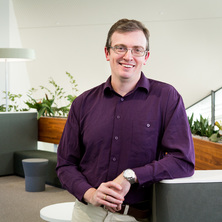
Overview
Background
Prof David Ascher is currently an NHMRC Investigator, immediate past Director of the Biotechnology Program, and Deputy Associate Dean (Research Partnerships) in the Faculty of Science at the University of Queensland. He is also Head of Computational Biology and Clinical Informatics at the Baker Institute.
David’s research focus is in modelling biological data to gain insight into fundamental biological processes. One of his primary research interests has been developing tools to unravel the link between genotype and phenotype, using computational and experimental approaches to understand the effects of mutations on protein structure and function. His group has developed a platform of over 40 widely used programs for assessing the molecular consequences of coding variants (>7 million hits/year).
Working with clinical collaborators in Australia, Brazil and UK, these methods have been translated into the clinic to guide the diagnosis, management and treatment of a number of hereditary diseases, rare cancers and drug resistant infections.
David has a B.Biotech from the University of Adelaide, majoring in Biochemistry, Biotechnology and Pharmacology and Toxicology; and a B.Sci(Hon) from the University of Queensland, majoring in Biochemistry, where he worked with Luke Guddat and Ron Duggleby on the structural and functional characterization of enzymes in the branched-chain amino acid biosynthetic pathway. David then went to St Vincent’s Institute of Medical Research to undertake a PhD at the University of Melbourne in Biochemistry. There he worked under the supervision of Michael Parker using computational, biochemical and structural tools to develop small molecules drugs to improve memory.
In 2013 David went to the University of Cambridge to work with Sir Tom Blundell on using fragment based drug development techniques to target protein-protein interactions; and subsequently on the structural characterisation of proteins involved in non-homologous DNA repair. He returned to Cambridge in 2014 to establish a research platform to characterise the molecular effects of mutations on protein structure and function- using this information to gain insight into the link between genetic changes and phenotypes. He was subsequently recruited as a lab head in the Department of Biochemistry and Molecular Biology at the University of Melbourne in 2016, before joining the Baker Institute in 2019 and the University of Queensland in 2021.
He is an Associate Editor of PBMB and Fronteirs in Bioinformatics, and holds honorary positions at Bio21 Institute, Cambridge University, FIOCRUZ, and the Tuscany University Network.
Availability
- Professor David Ascher is:
- Available for supervision
- Media expert
Fields of research
Research impacts
We have successfully translated our computational tools into the clinic and industry, including:
- Clinical detection of drug resistance from whole-genome sequencing of pathogens, including Tuburculosis and Leprosy
- Genetic counselling for rare diseases and cancers with Addenbrooke's Hospital and Brazilian Ministry of Health
- Patient stratification within clinical trials
- Implementation within industry drug and biologics development programs
The tools we have developed have also been widely adopted within existing academic programs including:
- Integration of intermolecular interaction calculations using our tool Arpeggio in the PDBe, the European resource for the collection, organisation and dissemination of data on biological macromolecular structures.
- Integration of our missense tolerance scores within the widely used VEP tool for variant characterisation.
- Implementation of our resistance prediction tools within the London School of Hygiene & Tropical Medicine's TB-Profiler tool.
Works
Search Professor David Ascher’s works on UQ eSpace
2025
Journal Article
Proximity proteomics reveals a mechanism of fatty acid transfer at lipid droplet-mitochondria- endoplasmic reticulum contact sites
Bezawork-Geleta, Ayenachew, Devereux, Camille J., Keenan, Stacey N., Lou, Jieqiong, Cho, Ellie, Nie, Shuai, De Souza, David P., Narayana, Vinod K., Siddall, Nicole A., Rodrigues, Carlos H. M., Portelli, Stephanie, Zheng, Tenghao, Nim, Hieu T., Ramialison, Mirana, Hime, Gary R., Dodd, Garron T., Hinde, Elizabeth, Ascher, David B., Stroud, David A. and Watt, Matthew J. (2025). Proximity proteomics reveals a mechanism of fatty acid transfer at lipid droplet-mitochondria- endoplasmic reticulum contact sites. Nature Communications, 16 (1) 2135. doi: 10.1038/s41467-025-57405-5
2025
Journal Article
Assessing the predicted impact of single amino acid substitutions in MAPK proteins for CAGI6 challenges
Turina, Paola, Petrosino, Maria, Enriquez Sandoval, Carlos A., Novak, Leonore, Pasquo, Alessandra, Alexov, Emil, Alladin, Muttaqi Ahmad, Ascher, David B., Babbi, Giulia, Bakolitsa, Constantina, Casadio, Rita, Cheng, Jianlin, Fariselli, Piero, Folkman, Lukas, Kamandula, Akash, Katsonis, Panagiotis, Li, Minghui, Li, Dong, Lichtarge, Olivier, Mahmud, Sajid, Martelli, Pier Luigi, Pal, Debnath, Panday, Shailesh Kumar, Pires, Douglas E. V., Portelli, Stephanie, Pucci, Fabrizio, Rodrigues, Carlos H. M., Rooman, Marianne, Savojardo, Castrense ... Capriotti, Emidio (2025). Assessing the predicted impact of single amino acid substitutions in MAPK proteins for CAGI6 challenges. Human Genetics, 144 (2-3) 245403, 1-16. doi: 10.1007/s00439-024-02724-8
2025
Journal Article
Definition and validation of prognostic phenotypes in moderate aortic stenosis
Sen, Jonathan, Wahi, Sudhir, Vollbon, William, Prior, Marcus, de Sá, Alex G.C., Ascher, David B., Huynh, Quan and Marwick, Thomas H. (2025). Definition and validation of prognostic phenotypes in moderate aortic stenosis. JACC: Cardiovascular Imaging, 18 (2), 133-149. doi: 10.1016/j.jcmg.2024.06.013
2025
Journal Article
Exploring the effects of missense mutations on protein thermodynamics through structure-based approaches: findings from the CAGI6 challenges
Rodrigues, Carlos H. M., Portelli, Stephanie and Ascher, David B. (2025). Exploring the effects of missense mutations on protein thermodynamics through structure-based approaches: findings from the CAGI6 challenges. Human Genetics, 144 (2-3), 327-335. doi: 10.1007/s00439-023-02623-4
2024
Journal Article
Use of the energy waveform electrocardiogram to detect subclinical left ventricular dysfunction in patients with type 2 diabetes mellitus
Soh, Cheng Hwee, de Sá, Alex G. C., Potter, Elizabeth, Halabi, Amera, Ascher, David B. and Marwick, Thomas H. (2024). Use of the energy waveform electrocardiogram to detect subclinical left ventricular dysfunction in patients with type 2 diabetes mellitus. Cardiovascular Diabetology, 23 (1) 91. doi: 10.1186/s12933-024-02141-1
2024
Journal Article
Insights into the structure of NLR family member X1: Paving the way for innovative drug discovery
Jewell, Shannon, Nguyen, Thanh Binh, Ascher, David B. and Robertson, Avril A.B. (2024). Insights into the structure of NLR family member X1: Paving the way for innovative drug discovery. Computational and Structural Biotechnology Journal, 23, 3506-3513. doi: 10.1016/j.csbj.2024.09.013
2024
Journal Article
piscesCSM: prediction of anticancer synergistic drug combinations
AlJarf, Raghad, Rodrigues, Carlos H. M., Myung, Yoochan, Pires, Douglas E. V. and Ascher, David B. (2024). piscesCSM: prediction of anticancer synergistic drug combinations. Journal of Cheminformatics, 16 (1) 81. doi: 10.1186/s13321-024-00859-4
2024
Journal Article
Discriminating Myalgic Encephalomyelitis/Chronic Fatigue Syndrome and comorbid conditions using metabolomics in UK Biobank
Huang, Katherine, G. C. de Sá, Alex, Thomas, Natalie, Phair, Robert D., Gooley, Paul R., Ascher, David B. and Armstrong, Christopher W. (2024). Discriminating Myalgic Encephalomyelitis/Chronic Fatigue Syndrome and comorbid conditions using metabolomics in UK Biobank. Communications Medicine, 4 (1) 248, 248. doi: 10.1038/s43856-024-00669-7
2024
Journal Article
Rifaximin prophylaxis causes resistance to the last-resort antibiotic daptomycin
Turner, Adrianna M., Li, Lucy, Monk, Ian R., Lee, Jean Y. H., Ingle, Danielle J., Portelli, Stephanie, Sherry, Norelle L., Isles, Nicole, Seemann, Torsten, Sharkey, Liam K., Walsh, Calum J., Reid, Gavin E., Nie, Shuai, Eijkelkamp, Bart A., Holmes, Natasha E., Collis, Brennan, Vogrin, Sara, Hiergeist, Andreas, Weber, Daniela, Gessner, Andre, Holler, Ernst, Ascher, David B., Duchene, Sebastian, Scott, Nichollas E., Stinear, Timothy P., Kwong, Jason C., Gorrie, Claire L., Howden, Benjamin P. and Carter, Glen P. (2024). Rifaximin prophylaxis causes resistance to the last-resort antibiotic daptomycin. Nature, 635 (8040), 969-977. doi: 10.1038/s41586-024-08095-4
2024
Journal Article
AlzDiscovery: A computational tool to identify Alzheimer's disease‐causing missense mutations using protein structure information
Pan, Qisheng, Parra, Georgina Becerra, Myung, Yoochan, Portelli, Stephanie, Nguyen, Thanh Binh and Ascher, David B. (2024). AlzDiscovery: A computational tool to identify Alzheimer's disease‐causing missense mutations using protein structure information. Protein Science, 33 (10) e5147, e5147. doi: 10.1002/pro.5147
2024
Journal Article
A new method for network bioinformatics identifies novel drug targets for mucinous ovarian carcinoma
Craig, Olivia, Lee, Samuel, Pilcher, Courtney, Saoud, Rita, Abdirahman, Suad, Salazar, Carolina, Williams, Nathan, Ascher, David B., Vary, Robert, Luu, Jennii, Cowley, Karla J., Ramm, Susanne, Li, Mark Xiang, Thio, Niko, Li, Jason, Semple, Tim, Simpson, Kaylene J., Gorringe, Kylie L. and Holien, Jessica K. (2024). A new method for network bioinformatics identifies novel drug targets for mucinous ovarian carcinoma. NAR Genomics and Bioinformatics, 6 (3) lqae096. doi: 10.1093/nargab/lqae096
2024
Journal Article
MTR3D‐AF2: Expanding the coverage of spatially derived missense tolerance scores across the human proteome using AlphaFold2
Kovacs, Aaron S., Portelli, Stephanie, Silk, Michael, Rodrigues, Carlos H. M. and Ascher, David B. (2024). MTR3D‐AF2: Expanding the coverage of spatially derived missense tolerance scores across the human proteome using AlphaFold2. Protein Science, 33 (8) e5112, e5112. doi: 10.1002/pro.5112
2024
Journal Article
EFG‐CS: Predicting chemical shifts from amino acid sequences with protein structure prediction using machine learning and deep learning models
Gu, Xiaotong, Myung, Yoochan, Rodrigues, Carlos H. M. and Ascher, David B. (2024). EFG‐CS: Predicting chemical shifts from amino acid sequences with protein structure prediction using machine learning and deep learning models. Protein Science, 33 (8) e5096, e5096. doi: 10.1002/pro.5096
2024
Conference Publication
Towards evolutionary-based automated machine learning for small molecule pharmacokinetic prediction
de Sá, Alex G. C. and Ascher, David B. (2024). Towards evolutionary-based automated machine learning for small molecule pharmacokinetic prediction. GECCO '24 Companion, Melbourne, VIC, Australia, 14-18 July 2024. New York, NY, United States: ACM. doi: 10.1145/3638530.3664166
2024
Journal Article
Deep-PK: deep learning for small molecule pharmacokinetic and toxicity prediction
Myung, Yoochan, de Sá, Alex G. C. and Ascher, David B. (2024). Deep-PK: deep learning for small molecule pharmacokinetic and toxicity prediction. Nucleic Acids Research, 52 (W1), W469-W475. doi: 10.1093/nar/gkae254
2024
Journal Article
PRIMITI: a computational approach for accurate prediction of miRNA-target mRNA interaction
Uthayopas, Korawich, de Sá, Alex G.C., Alavi, Azadeh, Pires, Douglas E.V. and Ascher, David B. (2024). PRIMITI: a computational approach for accurate prediction of miRNA-target mRNA interaction. Computational and Structural Biotechnology Journal, 23, 3030-3039. doi: 10.1016/j.csbj.2024.06.030
2024
Journal Article
DDMut-PPI: predicting effects of mutations on protein–protein interactions using graph-based deep learning
Zhou, Yunzhuo, Myung, YooChan, Rodrigues, Carlos H.M. and Ascher, David B. (2024). DDMut-PPI: predicting effects of mutations on protein–protein interactions using graph-based deep learning. Nucleic Acids Research, 52 (W1), W207-W214. doi: 10.1093/nar/gkae412
2024
Journal Article
Targeting the Plasmodium falciparum UCHL3 ubiquitin hydrolase using chemically constrained peptides
King, Harry R., Bycroft, Mark, Nguyen, Thanh-Binh, Kelly, Geoff, Vinogradov, Alexander A., Rowling, Pamela J E, Stott, Katherine, Ascher, David B., Suga, Hiroaki, Itzhaki, Laura S. and Artavanis-Tsakonas, Katerina (2024). Targeting the Plasmodium falciparum UCHL3 ubiquitin hydrolase using chemically constrained peptides. Proceedings of the National Academy of Sciences of the United States of America, 121 (21) e2322923121. doi: 10.1073/pnas.2322923121
2024
Journal Article
Engineering G protein‐coupled receptors for stabilization
Velloso, João Paulo L., de Sá, Alex G. C., Pires, Douglas E. V. and Ascher, David B. (2024). Engineering G protein‐coupled receptors for stabilization. Protein Science, 33 (6) e5000, 1-13. doi: 10.1002/pro.5000
2024
Journal Article
Joint genotypic and phenotypic outcome modeling improves base editing variant effect quantification
Ryu, Jayoung, Barkal, Sam, Yu, Tian, Jankowiak, Martin, Zhou, Yunzhuo, Francoeur, Matthew, Phan, Quang Vinh, Li, Zhijian, Tognon, Manuel, Brown, Lara, Love, Michael I., Bhat, Vineel, Lettre, Guillaume, Ascher, David B., Cassa, Christopher A., Sherwood, Richard I. and Pinello, Luca (2024). Joint genotypic and phenotypic outcome modeling improves base editing variant effect quantification. Nature Genetics, 56 (5), 925-937. doi: 10.1038/s41588-024-01726-6
Supervision
Availability
- Professor David Ascher is:
- Available for supervision
Before you email them, read our advice on how to contact a supervisor.
Supervision history
Current supervision
-
Doctor Philosophy
Computational approaches to engineer and modulate G protein-coupled receptors
Principal Advisor
-
Doctor Philosophy
Exploring Cardiotoxicity Risk Factors
Principal Advisor
Other advisors: Dr Thanh-Binh Nguyen
-
Doctor Philosophy
Developing structure-based deep learning methods to predict mutation effects on proteins
Principal Advisor
-
Doctor Philosophy
Exploring Cardiotoxicity Risk Factors
Principal Advisor
Other advisors: Dr Thanh-Binh Nguyen
-
Master Philosophy
Explore the dark spots in PDB
Principal Advisor
-
Doctor Philosophy
Post-transcriptional gene regulation: towards a better understanding of pathogenesis and medical applications
Principal Advisor
-
Doctor Philosophy
Exploring Cardiotoxicity Risk Factors
Principal Advisor
Other advisors: Dr Thanh-Binh Nguyen
-
Doctor Philosophy
Computational approaches to engineer and modulate G protein-coupled receptors
Principal Advisor
-
Doctor Philosophy
Personalising treatments for genetic diseases
Principal Advisor
Other advisors: Dr Stephanie Portelli
-
Doctor Philosophy
Deep Learning Algorithms for Polygenic Genotype-Phenotype Predictions and the development of genetics computation tools
Principal Advisor
-
Doctor Philosophy
Computer-aided drug design: predicting and mitigating drug toxicity
Principal Advisor
Other advisors: Dr Stephanie Portelli
-
Doctor Philosophy
Towards the accurate functional characterisation of protein coding mutations
Principal Advisor
Other advisors: Dr Stephanie Portelli, Dr Thanh-Binh Nguyen
-
Doctor Philosophy
Improving rational antibody design using machine learning
Principal Advisor
-
Doctor Philosophy
Harnessing AlphaFold and explainable AI to better characterise human missense variants and diseases
Principal Advisor
Other advisors: Dr Stephanie Portelli, Dr Thanh-Binh Nguyen
-
Doctor Philosophy
Machine Learning for Protein Dynamics: Predicting Post-Translational Modifications and Mutation Effects
Principal Advisor
-
Doctor Philosophy
Using Deep Learning in Cell & Gene Therapy
Principal Advisor
Other advisors: Dr Stephanie Portelli
-
Doctor Philosophy
Protein structure guided precision medicine
Principal Advisor
Other advisors: Professor Phil Hugenholtz, Dr Stephanie Portelli
-
Master Philosophy
Explore the dark spots in PDB
Principal Advisor
-
Doctor Philosophy
Rational protein engineering and inhibition
Principal Advisor
-
Doctor Philosophy
Post-transcriptional gene regulation: towards a better understanding of pathogenesis and medical applications
Principal Advisor
-
Doctor Philosophy
Unravelling the Physicochemical Drivers of Biomolecular Self-Assembly though Multiscale Simulations
Associate Advisor
Other advisors: Dr Evelyne Deplazes, Professor Megan O'Mara
-
Doctor Philosophy
Breaking the chain of inflammation through targetting NLR proteins
Associate Advisor
Other advisors: Professor Avril Robertson
-
Doctor Philosophy
Therapeutic Resolution of Inflammation in the Central Nervous System for Neuroprotection in Parkinson's Disease
Associate Advisor
Other advisors: Professor Avril Robertson
-
Doctor Philosophy
Use of structural phylogeny and reconciliation in molecular phylogenetics
Associate Advisor
Other advisors: Dr Kate Bowerman, Professor Phil Hugenholtz
-
Doctor Philosophy
Therapeutic Resolution of Inflammation in the Central Nervous System for Neuroprotection in Parkinson's Disease
Associate Advisor
Other advisors: Professor Avril Robertson
-
Doctor Philosophy
Computational design of targeted lipid technologies
Associate Advisor
Other advisors: Professor Megan O'Mara
Completed supervision
-
2025
Doctor Philosophy
Computational approaches to engineer and modulate G protein-coupled receptors
Principal Advisor
Media
Enquiries
Contact Professor David Ascher directly for media enquiries about their areas of expertise.
Need help?
For help with finding experts, story ideas and media enquiries, contact our Media team: